Employment of Multi-classifier and Multi-domain Features for PCG Recognition
2019 12th International Conference on Developments in eSystems Engineering (DeSE)(2019)
摘要
In this paper, multi-classifier of K-Nearest Neighbor and Support Vector Machine (SVM) classifiers with multi-domain features are employed, as a proposed methodology for recognizing the normality status of the heart sound recordings (so-called Phonocardiogram - PCG). The PhysioNet/CinC Challenge 2016 offers the dataset used in this paper. Heart sounds are complex signals and required trained clinicians for diagnosis, which motivated us to develop an algorithm for automatic classification of heart sounds into two classes normal and abnormal. Entropy, high-order statistics, Cyclo-stationarity, cepstrum, the frequency spectrum of records, energy, state amplitude, the frequency spectrum of states, and time interval, are the nine-domain features employed. These domain features are extracted to a total of 527 features. These features have been used to train the KNearest Neighbor and Support Vector Machine (SVM) classifiers. Fine-KNN classifier outperformed types of SVM classifiers by achieving the accuracy of 93.5% while Cubic-SVM classifier achieved 90.9% which is the highest accuracy of all SVMs. The Fine-KNN classifier and the proposed features are both efficient and significant for PCG recognition.
更多查看译文
关键词
SVM,KNN,Phonocardiogram,PCG,Heart sound Recognition,Multi-domain features.
AI 理解论文
溯源树
样例
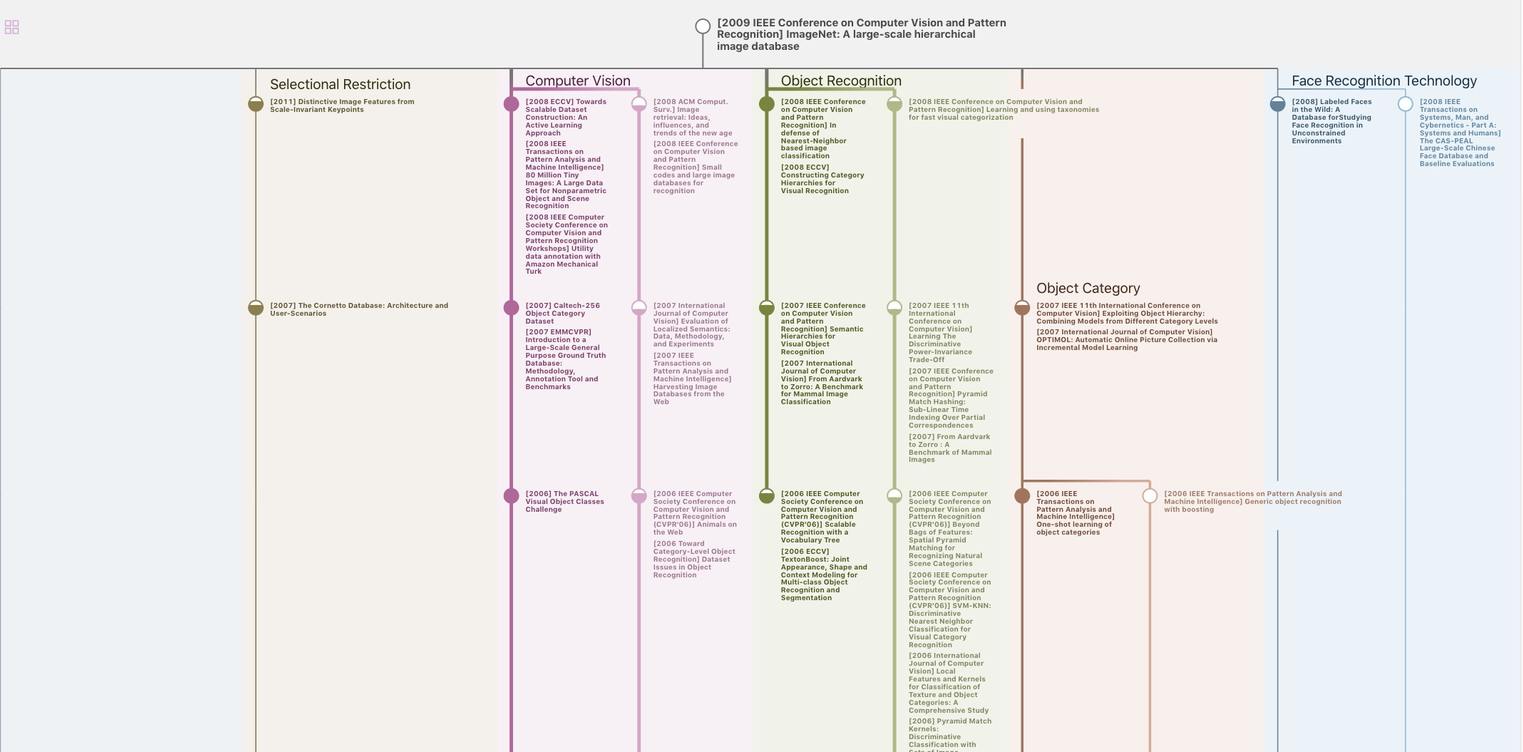
生成溯源树,研究论文发展脉络
Chat Paper
正在生成论文摘要