Learning Joint Wasserstein Auto-Encoders for Joint Distribution Matching
user-5e8423bd4c775ee160ac3e1a(2018)
Abstract
We study the joint distribution matching problem which aims at learning bidirectional mappings to match the joint distribution of two domains. This problem occurs in unsupervised image-to-image translation and video-to-video synthesis tasks, which, however, has two critical challenges:(i) it is difficult to exploit sufficient information from the joint distribution;(ii) how to theoretically and experimentally evaluate the generalization performance remains an open question. To address the above challenges, we propose a new optimization problem and design a novel Joint Wasserstein Auto-Encoders (JWAE) to minimize the Wasserstein distance of the joint distributions in two domains. We theoretically prove that the generalization ability of the proposed method can be guaranteed by minimizing the Wasserstein distance of joint distributions. To verify the generalization ability, we apply our method to unsupervised video-to-video synthesis by performing video frame interpolation and producing visually smooth videos in two domains, simultaneously. Both qualitative and quantitative comparisons demonstrate the superiority of our method over several state-of-the-arts.
MoreTranslated text
AI Read Science
Must-Reading Tree
Example
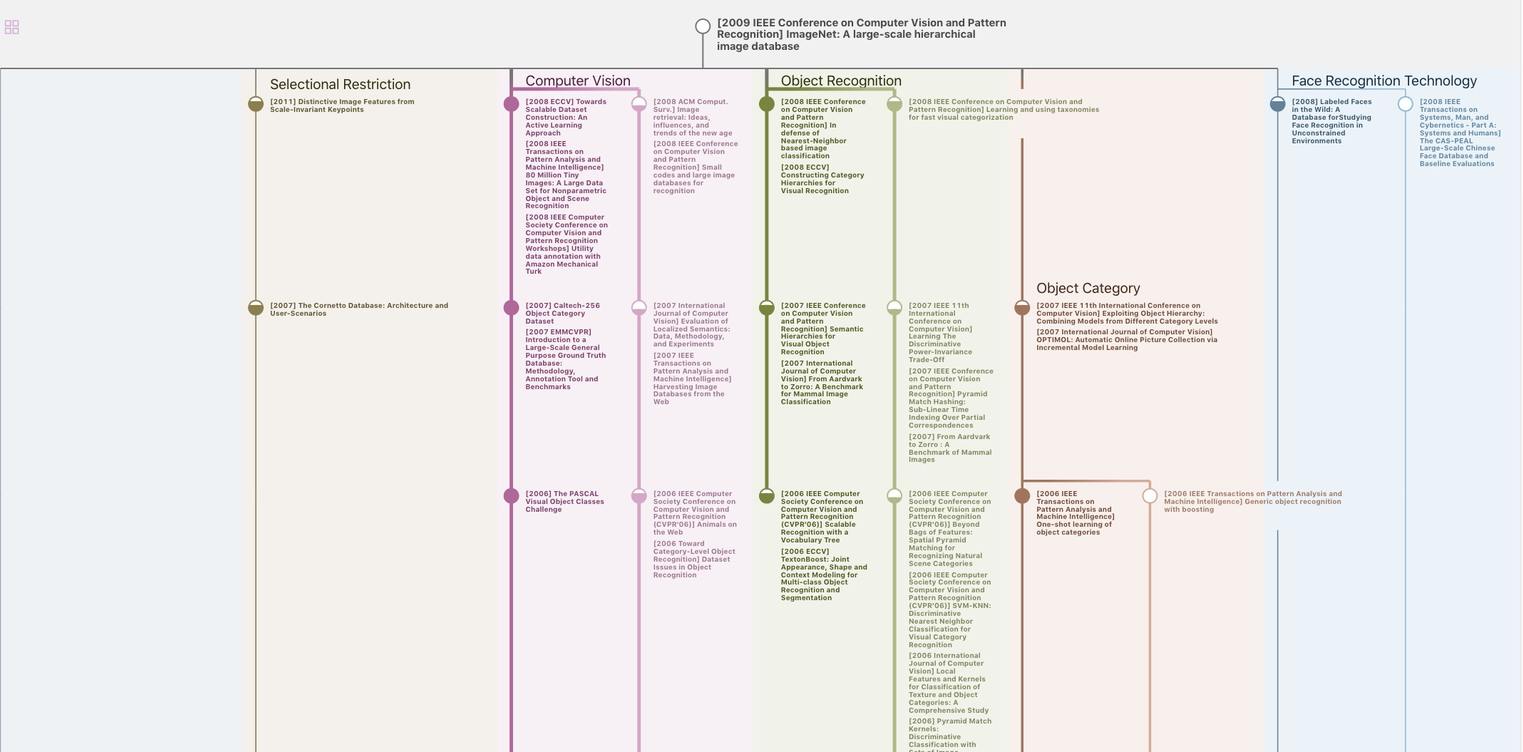
Generate MRT to find the research sequence of this paper
Chat Paper
Summary is being generated by the instructions you defined