Adversarial NLI for Factual Correctness in Text Summarisation Models
CoRR(2020)
Abstract
We apply the Adversarial NLI dataset to train the NLI model and show that the model has the potential to enhance factual correctness in abstract summarization. We follow the work of Falke et al. (2019), which rank multiple generated summaries based on the entailment probabilities between an source document and summaries and select the summary that has the highest entailment probability. The authors' earlier study concluded that current NLI models are not sufficiently accurate for the ranking task. We show that the Transformer models fine-tuned on the new dataset achieve significantly higher accuracy and have the potential of selecting a coherent summary.
MoreTranslated text
AI Read Science
Must-Reading Tree
Example
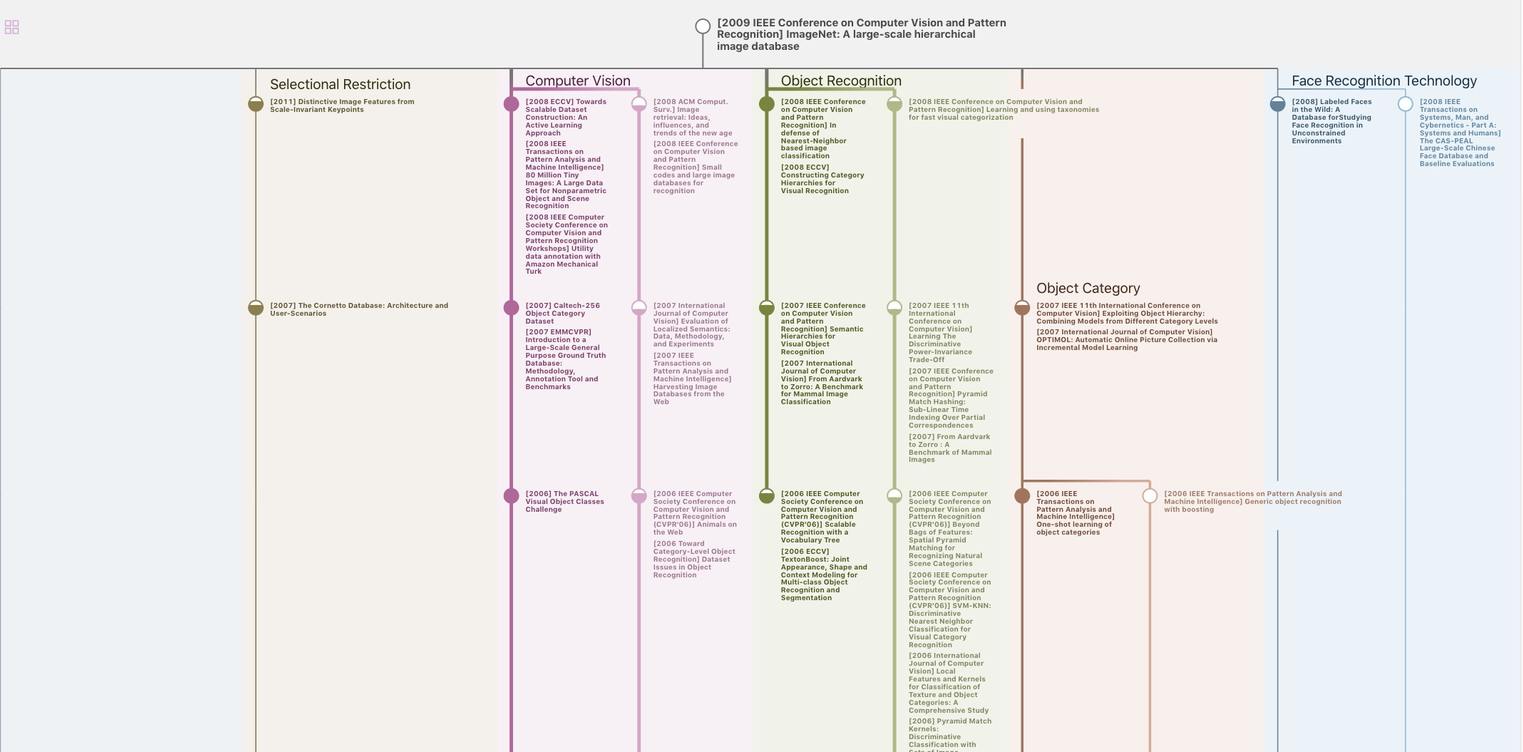
Generate MRT to find the research sequence of this paper
Chat Paper
Summary is being generated by the instructions you defined