HIVEC: A Hierarchical Approach for Vector Representation Learning of Graphs
user-5d54d98b530c705f51c2fe5a(2018)
摘要
This paper presents a new method : HIVEC to learn latent vector representations of graphs in a manner that captures the semantic dependencies of sub-structures. The representations can then be used in machine learning algorithms for tasks such as graph classification, clustering etcetera. The method proposed is unsupervised and uses the information of co-occurrence of sub-structures. It introduces a notion of hierarchical embeddings that allows us to avoid repetitive learning of sub-structures for every new graph. As an alternative to deep learning methods, the edit distance similarity between sub-structures is also used to learn vector representations. We compare the performance of these methods against previous work.
更多查看译文
AI 理解论文
溯源树
样例
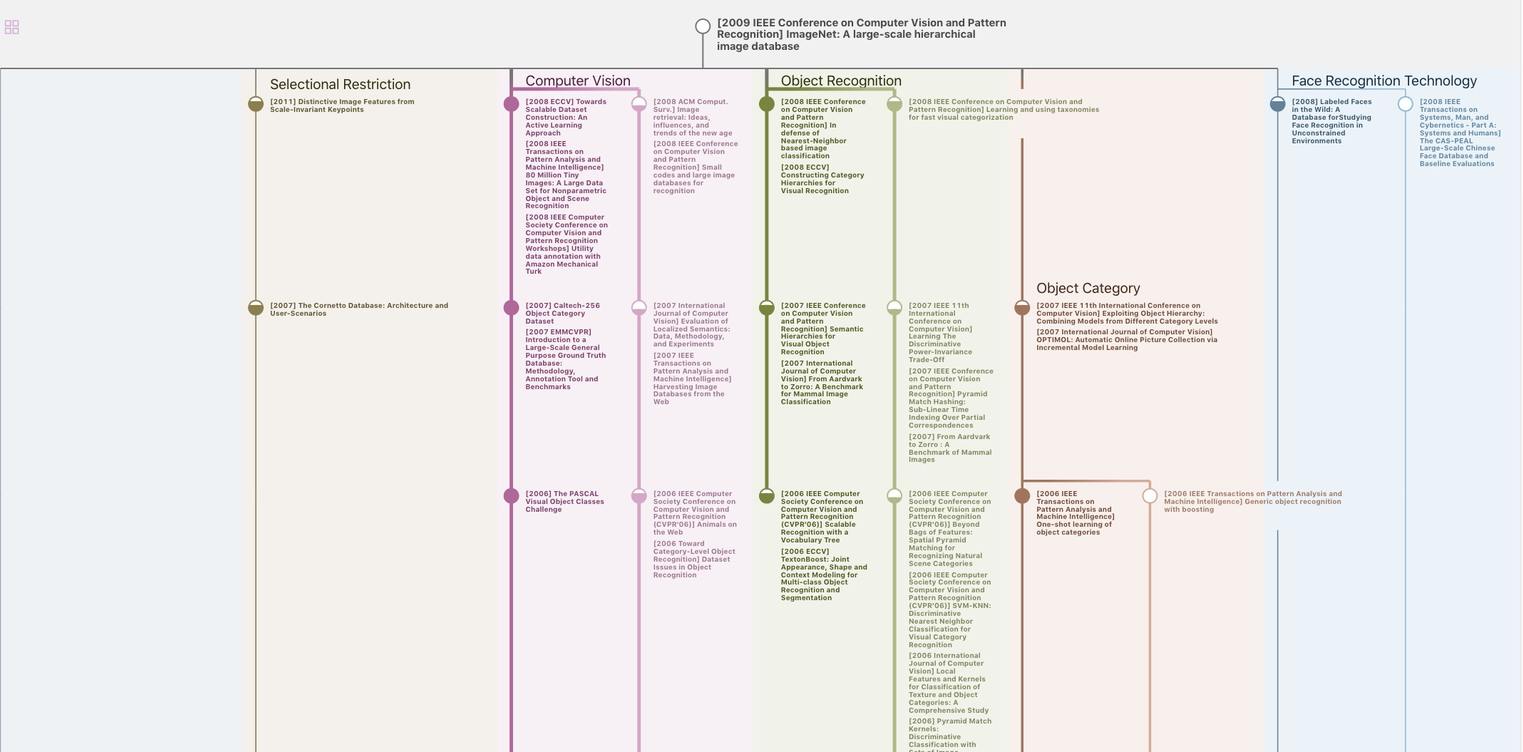
生成溯源树,研究论文发展脉络
Chat Paper
正在生成论文摘要