PnPNet: End-to-End Perception and Prediction With Tracking in the Loop
CVPR(2020)
摘要
We tackle the problem of joint perception and motion forecasting in the context of self-driving vehicles. Towards this goal we propose PnPNet, an end-to-end model that takes as input sequential sensor data, and outputs at each time step object tracks and their future trajectories. The key component is a novel tracking module that generates object tracks online from detections and exploits trajectory level features for motion forecasting. Specifically, the object tracks get updated at each time step by solving both the data association problem and the trajectory estimation problem. Importantly, the whole model is end-to-end trainable and benefits from joint optimization of all tasks. We validate PnPNet on two large-scale driving datasets, and show significant improvements over the state-of-the-art with better occlusion recovery and more accurate future prediction.
更多查看译文
关键词
large-scale driving datasets,joint optimization,trajectory estimation problem,data association problem,trajectory level features,tracking module,time step object tracks,input sequential sensor data,end-to-end model,motion forecasting,joint perception,end-to-end perception,PnPNet,occlusion recovery
AI 理解论文
溯源树
样例
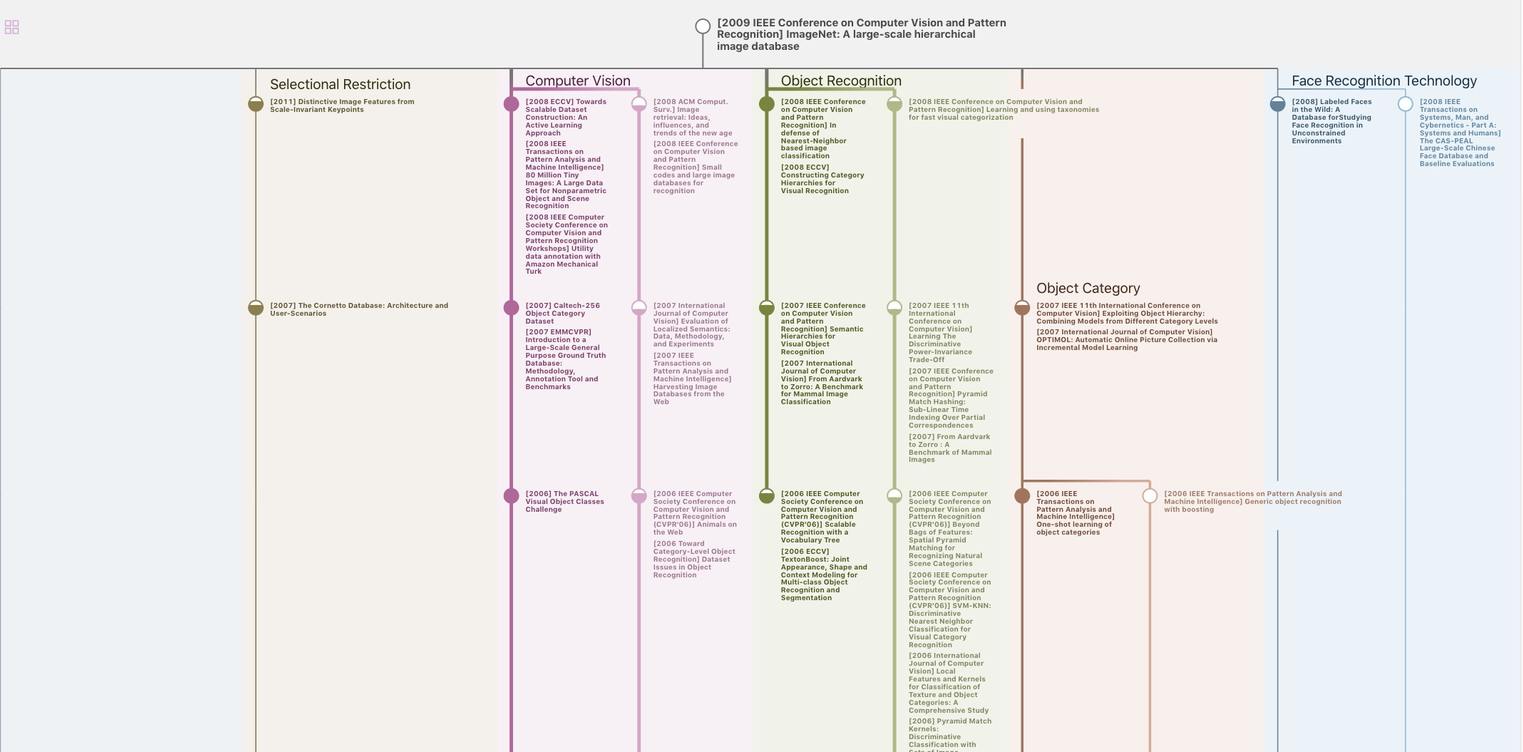
生成溯源树,研究论文发展脉络
Chat Paper
正在生成论文摘要