Global Optimality for Point Set Registration Using Semidefinite Programming
CVPR(2020)
摘要
In this paper we present a study of global optimality conditions for Point Set Registration (PSR) with missing data. PSR is the problem of aligning multiple point clouds with an unknown target point cloud. Since non-linear rotation constraints are present the problem is inherently non-convex and typically relaxed by computing the Lagrange dual, which is a Semidefinite Program (SDP). In this work we show that given a local minimizer the dual variables of the SDP can be computed in closed form. This opens up the possibility of verifying the optimally, using the SDP formulation without explicitly solving it. In addition it allows us to study under what conditions the relaxation is tight, through spectral analysis. We show that if the errors in the (unknown) optimal solution are bounded the SDP formulation will be able to recover it.
更多查看译文
关键词
point set registration,PSR,unknown target point cloud,nonlinear rotation constraints,nonconvex,Lagrange dual,dual variables,SDP formulation,semidefinite programming,global optimality conditions,spectral analysis,local minimizer,missing data
AI 理解论文
溯源树
样例
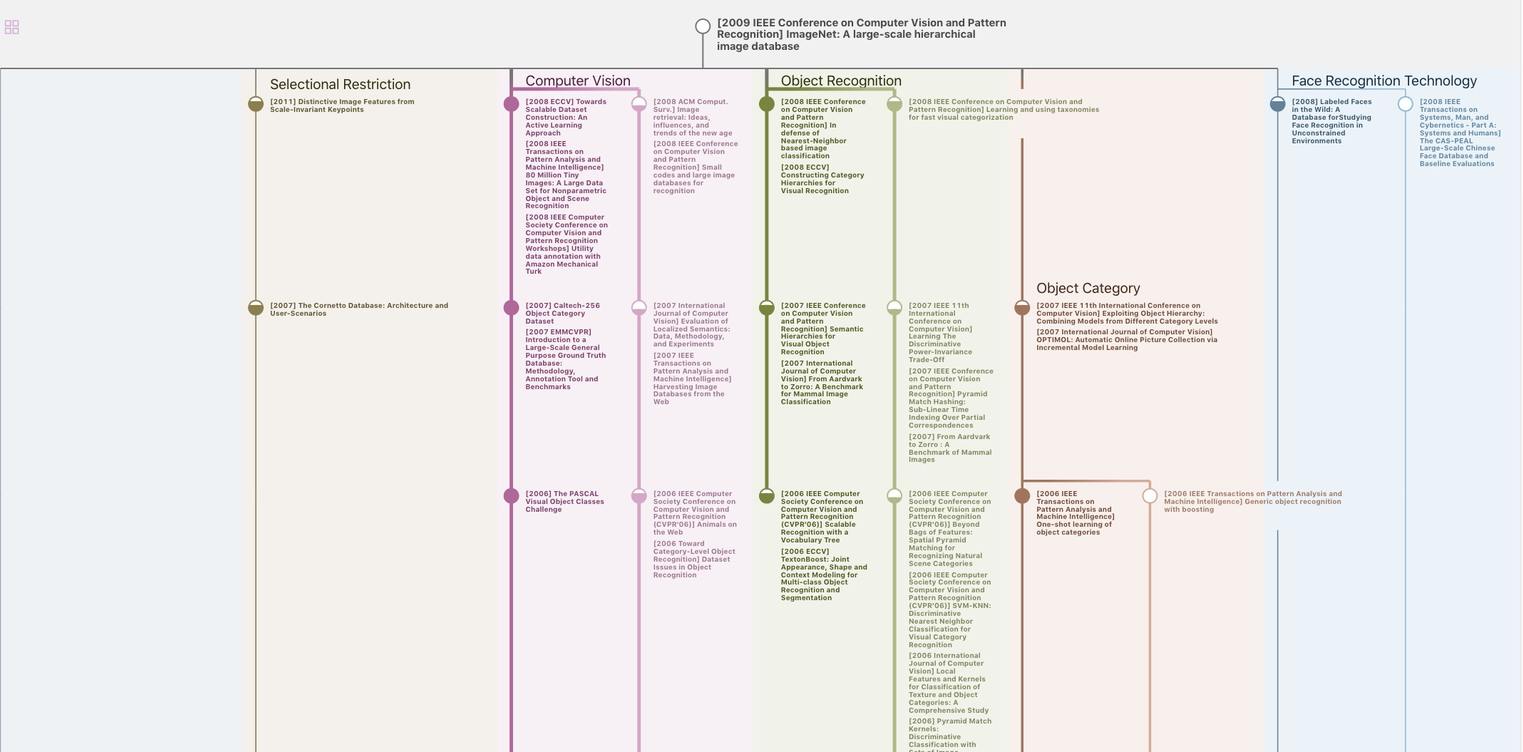
生成溯源树,研究论文发展脉络
Chat Paper
正在生成论文摘要