P126 An analysis of the quality and readability of online information for osteoarthritis
Rheumatology(2020)
摘要
Abstract Background Osteoarthritis is the most common cause of disability in people over 65 years old. The readability of of online osteoarthritis information has never been assessed. A 2003 study found the quality of online osteoarthritis information to be poor. This study reviews the quality of online information regarding osteoarthritis in 2018 using three validated scoring systems. Readability is reviewed for the first time, again using three validated tools. Methods The term osteoarthritis was searched across the three most popular English language search engines. The first 25 pages from each search engine were analysed. Duplicate pages, websites featuring paid advertisements, inaccessible pages (behind a pay wall, not available for geographical reasons) and non-text pages were excluded. Readability was measured using Flesch Reading Ease Score (FRES), Flesch-Kincaid Grade Level (FKGL) and Gunning-Fog Index (GFI). Website quality was scored using the the Journal of the American Medical Association (JAMA) benchmark criteria and DISCERN criteria. Presence or absence of HONcode certification, age of content, content producer and author characteristics were noted. Results 37 unique websites were suitable for analysis. Readability varied by assessment tool from 8th to 12th grade level. This compares with the recommended 7- 8th grade level. One (2.7%) website met all four JAMA Criteria. Mean DISCERN quality of information for OA websites was “fair”, comparing favourably with the “poor” grading of a 2003 study. HONCode endorsed websites (43.2%) were of a statistically significantly higher quality. Conclusion Quality of online health information for OA is “fair”. 2.7% of websites met JAMA benchmark criteria for quality. Readability was equal to or more difficult than recommendations. HONcode certification was indicative of higher quality, but not readability. Disclosures K. Murray None. T. Murray None. C. Low None. A. O'Rourke None. D.J. Veale None.
更多查看译文
AI 理解论文
溯源树
样例
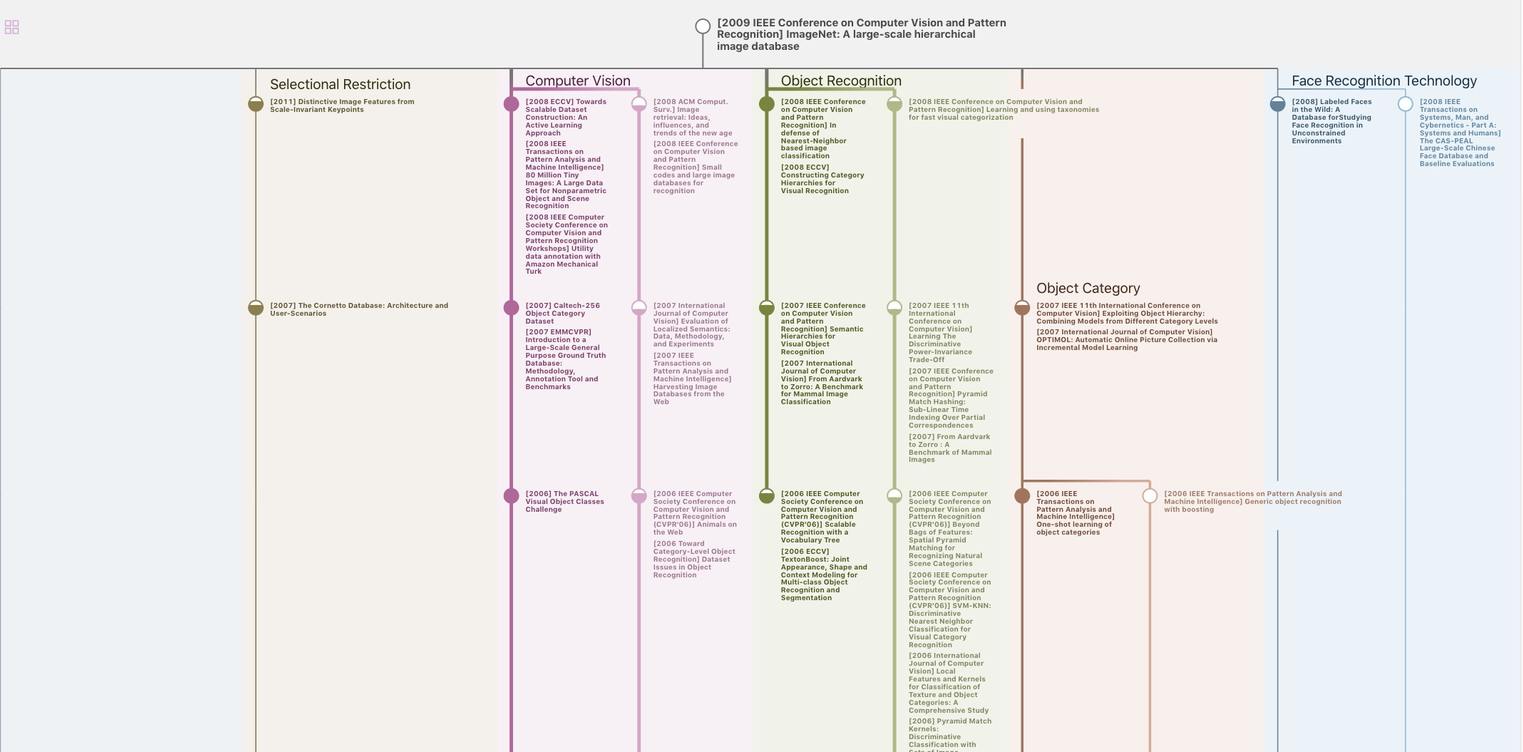
生成溯源树,研究论文发展脉络
Chat Paper
正在生成论文摘要