Mathematical Modeling of Estrogen Degradation in Enzymatic Membrane Reactor
Industrial biotechnology(2020)
摘要
Industrial BiotechnologyVol. 16, No. 2 Catalyzing InnovationFree AccessMathematical Modeling of Estrogen Degradation in Enzymatic Membrane ReactorCamila L. S. Carvalho, Fabrício M. Gomes, and Félix M. PereiraCamila L. S. CarvalhoUniversity of São Paulo, Lorena, São Paulo, BrazilSearch for more papers by this author, Fabrício M. GomesUniversity of São Paulo, Lorena, São Paulo, BrazilSearch for more papers by this author, and Félix M. PereiraDr. Félix M. Pereira is a professor in the Department of Chemical Engineering, School of Engineering of Lorena, University of São Paulo, Lorena - SP, Brazil. E-mail Address: felixmpereira@usp.br.University of São Paulo, Lorena, São Paulo, BrazilSearch for more papers by this authorPublished Online:17 Apr 2020https://doi.org/10.1089/ind.2020.29200.cscAboutSectionsPDF/EPUB Permissions & CitationsPermissionsDownload CitationsTrack CitationsAdd to favorites Back To Publication ShareShare onFacebookTwitterLinked InRedditEmail IntroductionEstrogens are endocrine-disrupting chemicals (EDCs) that enter waterbodies via wastewater. Because of this, some countries are creating legislation aimed at developing processes to degrade EDCs in wastewater-treatment plants. Estrone and estradiol are the estrogens found in high concentrations in waterbodies. Some processes in literature suggest using the enzyme laccase to degrade EDCs. This work mathematically models EDCs degradation by laccase in an enzymatic membrane reactor (EMR). The models were built from the mass balances of EDCs and active enzymes in EMR, including Michaelis Menten kinetics and a parameter coupling the enzyme activation, deactivation, and loss by the outflow. Experimental data from literature were used in the models' regressions. The experiments considered three experimental conditions to evaluate two process parameters: EMR residence time and O2 pulse frequency. The models' assessment considered the following statistical tests: Student's t to evaluate the parameters' significance; Akaike Information Criteria to select the best models; and R2 to evaluate the models' fit. Results show the best assessed kinetic models were able to represent degradation in an EMR, and the best operation conditions were a residence time of 4 h and an O2 pulse frequency of 0.5 h. The methods and mathematical models presented in this manuscript can contribute to wastewater plant design for reducing contamination by EDCs in waterbodies.Humans are the main producers of estrogens currently present in the waterbodies.1 Estrone and estradiol—quoted in literature as EDCs found in high amount in waterbodies2—are toxic when found in micro concentrations for both aquatic and nonaquatic animals, including human beings.3,4 Laccase, a multicopper polyphenol oxidase enzyme present in many organisms, can degrade these compounds with a low degree of substrate specificity.5,6Operating with free enzymes in a conventional reactor requires high enzyme consumption because they are washed from the reactor by the outflow. To reduce the enzyme loss, an ultrafiltration membrane can be used to recover enzymes back to the reaction vessel. The system is composed of a continuous stirred tank reactor and ultrafiltration using a semipermeable membrane, or EMR.7,8An important step for reactor design and operation is the estimation of kinetic parameters by the regression of phenomenological models to experimental data.9 This work presents an estimation of kinetic parameters of estrone and estradiol degradation by laccase from Myceliophthora thermophila performed in an EMR.8Mathematical ModelingFigure 1 presents a flow scheme of the EMR used for mass balance building. In Fig. 1, F is the volumetric flow rate of inlet/outlet flow (L h−1); EDCi is the concentration of EDC of inlet flow (mg L−1); V is the working volume of the reactor (L); EDC is the concentration of EDC in the reactor (mg L−1); E is the concentration of active enzymes in the reactor (U L−1); R is the volumetric flow rate in recycle (L h−1); EDCo is the concentration of EDC of outlet flow (mg L−1); Eo is the concentration of enzymes of outlet flow (mg L−1); τ = V/F is the hydraulic residence time (h).Fig. 1. Flow scheme of EMR.Assuming that EDC concentration in the outlet is close to the concentration inside the reactor, EDCo = EDC (Fig. 1), Equations 1 and 2 were built from the mass balance of EDCs and active enzymes in the EMR. (Equation 1)where v (g L−1 h−1) is the rate of EDC degradation. (Equation 2)where c (h−1) is a constant resulting from the balance between the enzyme constants of activation (ka), inactivation (ki), and enzyme loss by the outflow (klo) given by Equation 3. (Equation 3)Equations 1 and 2 are subjected to the initial conditions (at t = 0) presented by Equations 4 and 5, respectively. (Equation 4)(Equation 5)where Ei is the initial concentration of active enzymes in the EMR.The integration of Equation 1 depends on the kinetic equation for v. For Equation 2, the integration can be made analytically, resulting in Equation 6. (Equation 6)A consolidated kinetic equation to estimate the rate of substrate consumption (v) in enzymatic catalysis is the Michaelis-Menten kinetic, presented by Equation 7.10,11,12(Equation 7)where, kd (mg U−1 h−1), KM (mg L−1) and vd = kd · Ei (g L−1 h−1) are constants.For EDC << KM, Equation 7 can be approximated to first-order kinetics as shown in Equation 8, where (h−1).13(Equation 8)For EDC >> KM, Eq. 7 can be approximated to zero-order kinetics as shown in Eq. 9.13(Equation 9)If the constant c is statistically insignificant, Equations 7, 8, and 9 are reduced to those represented by Equations 10, 11, and 12. (Equation 10)(Equation 11)(Equation 12)The percentage yield of EDC degradation, Y (%), is estimated by the quotient between the mass of EDC degraded and the mass of EDC added to the reactor multiplied by 100%, resulting in Equation 13. (Equation 13)The aim of this work is to evaluate, based on statistical analysis, the form of the kinetic equation (Equations 7–12) that best describes the data found in literature,8 using technology advances and improved understanding of EDCs degradation, to achieve a reliable process for wastewater treatment.Material and MethodsMATERIALSThe software GetData Graph Digitizer® was used to extract experimental data from literature8 that evaluated the degradation of two estrogen types—estrone and estradiol—by laccase from M. thermophila in an EMR and study the influence of hydraulic residence time (τ) and O2 pulse frequency. The experiments shown on Table 18 were performed at pH 7 with initial laccase activity of 500 U L−1.Table 1. Experimental ConditionsEXPERIMENTEDC ADDITION RATE (mg L−1 h−1)HYDRAULIC RESIDENCE TIME (h)O2 PULSE FREQUENCY (h)12212220.53140.5The Wolfram Mathematica® 12 software (WM) was used in this work for regression and statistical tests.METHODSThis work used the following functions of WM: NDSolveValue to solve the ordinary differential equations (Equation 1);NonlinearModelFit for regression, estimation of kinetic parameters and statistical tests;NIntegrate to solve the integral in Equation 13.The evaluation of the model adjustment to experimental data took into account the following parameters:Sum of Squares of Regression (SSR), presented in Equation 14. SSR is the sum of the squares of the deviations of the predicted values of EDC () from the mean value of experimental values of EDC () for each experimental point i (for i from 1 to the number of experimental points n);14 ((Equation 14)) Residual Sum of Squares (RSS), presented in Equation 15. RSS is the sum of the squares of residuals between and the EDC for each experimental point (EDCi). It is a measure of the discrepancy between the data and an estimation model. A small RSS indicates a tight fit of the model to the data;(Equation 15)Analysis of parameters significance for Student's t-test (p value): if p < 0.05, the parameter is significant for the confidence level of 95%;15Coefficient of determination, R2, Equation 16. R2 is a statistical measure of how well the regression predictions approximate the real data points; a R2 near to 1 indicates that the regression predictions have a good fit to the experimental data;(Equation 16)Akaike information criterion (AIC), Equation 17 (where k is the number of estimated parameters in the model and n is the number of experimental data points). AIC is an asymptotically unbiased estimator of the Kullback-Leibler divergence (K-L). In model assessment, the K–L divergence is a measure of how one model response is different of a second one. Thus, the best model loses the least information relative to other models in a set of mathematical models. The lower the AIC, the better the model;16(Equation 17)Corrected Akaike information criterion (AICc), Equation 18. AICc is preferred to AIC for small amounts of experimental data. As a rule, AICc is indicated when n/k < 40 n.17,18(Equation 18)The choice of a good model to represent the experimental data depends on both the complexity and the adjustment of the model. Mathematical models with poor adjustment may not satisfactorily describe the experimental data behavior, resulting in a poor fit of the model to the experimental data.Complex models tend to describe the experimental points well, however, they also tend to distribute the experimental errors in their parameters, resulting in parameters with low or no statistical significance. Besides that, they may have a low capacity to describe the experimental behavior due to the occurrence of a random noise in the answer. Thereby, a reliable model will be one that coordinates a good fit to the experimental data to a good capacity of representing the behavior of the experiment. Thus, the corrected Akaike Information Criterion (AICc) was used in this work aiming at assessing the better between the six kinetic models presented (Equations 7–12).The assessment of the candidate models took into account the differences between the AICc,j (Δj) of a mathematical model (j) and the minimum value (min) of AICc for the set of models, as shown in Equation 19.19(Equation 19)The Δj of the best model in the set is equal to zero and the other models have positive Δj. As a rule, models with Δj ≤ 2 have substantial predictability support, those with 2 < Δj ≤ 7 have considerably less support and models with Δj > 7 have no support.19Accounting for these aspects, this work considered the following sequence of requirements in the model assessment:1.Statistical significance of parameters based on Student's t-test to 95% of confidence (pv ≤ 0.05 to vd or v1; pc ≤ 0.05 to c; and pKM ≤ 0.05 to KM);2.Support of the model to predict the experiments behavior using the corrected Akaike Information Criterion (AICc), considering a substantial support level (Δ ≤ 2);3.Quality of model adjustment to experimental data R2 ≈ 1.The best assessed model in estimating the percentage yield of EDCs degradation, Equation 13, was used to evaluate the effect of each experimental condition on the degradation of estrone and estradiol.Results and DiscussionTable 2 presents the results of regression and statistical tests used to assess the best models for each experiment of estrone and estradiol degradation in the EMR.Table 2. Assessment of Kinetic Models for Estrone and Estradiol Degradation in the EMREDCEXPERIMENTEQUATIONvd or v1pvcpcKMpKMR2AICcΔestrone17132360.9980.0090.136300470.9980.9995−53.23.5estrone180.44050.0000.0090.105--0.9995−56.70estrone190.61570.0000.0640.000--0.9917−7.049.7estrone11085750.997--39730.9970.9994−53.63.1estrone1110.46310.000----0.9994−56.60.1estrone1120.84390.000----0.97757.964.6estrone271.20530.0000.0110.0000.0250.0000.9999−108.50estrone281.40200.0000.0600.001--0.9877−15.093.5estrone291.14570.0000.0160.000--0.9996−75.633.5estrone2101.33350.000--0.0800.0000.9992−66.243.2estrone2111.01610.000----0.9763−6.2102estrone2121.23290.000----0.9958−37.271.3estrone371.73040.0000.1280.0001.6320.0050.9992−79.40estrone380.69900.0000.1480.000--0.9981−69.210.2estrone390.57070.0000.0750.000--0.9268−6.972.5estrone310497711.000--680581.0000.9247−6.572.9estrone3111.36740.000----0.9247−9.569.9estrone3120.79420.000----0.691014.583.7estradiol17730530.9980.0750.000945080.9980.9981−45.93.6estradiol180.77300.0000.0750.000--0.9981−49.50estradiol190.93740.0000.0660.000--0.96224.254estradiol110141990.999--160830.9990.9803−7.542estradiol1111.13260.000----0.9803−10.539estradiol1121.29780.000----0.879322.172estradiol271.62740.0000.00810.0000.0630.0000.9998−107.70estradiol282.53360.000−0.0330.019--0.9854−30.178estradiol291.42010.0000.0170.000--0.9940−46.262estradiol2100.11980.000--1.8200.0000.9984−70.837estradiol2112.14690.000----0.9792−26.881estradiol2121.54280.000----0.9735−22.486estradiol372.10770.0010.1580.0001.6010.0100.9990−86.10estradiol380.91920.0000.1820.000--0.9983−79.96.2estradiol390.65510.0000.0610.000--0.8779−7.279estradiol3102361041.000--4665151.0000.8791−7.479estradiol3111.97590.000----0.8791−10.576estradiol3120.85570.000----0.574810.997Results in Table 2 indicate that the best kinetic equations were Equation 11 for estrone in Experiment 1; Equation 8 for estradiol in Experiment 1; and Equation 7 for estrone and estradiol in both Experiments 2 and 3.For the best kinetic equations, all estimated parameters are statistically significant to 95% of confidence level to Student's t-test and have R2> 0.998, indicating a good model fit to experimental data.Considering the best assessed kinetic models, the parameter related to the enzyme activation/inactivation/loss (c, Equation 3) is insignificant to estrone and Experiment 1 and has maximum values for Experiment 3 conditions, indicating that τ = 4 h and oxygen pulse frequency of 0.5 h (Table 1) was the experimental condition that promoted the highest concentration of active enzymes in the EMR, increasing the rate of EDC consumption.Figure 2 presents the adjustment of best models to experimental data and the percentage yield of EDCs degradation, Y (%).Fig. 2. Adjustment of the best models to experimental data and the percentage yield of EDCs degradation.The results presented in Fig. 2 indicated that the best models were able to represent EDCs degradation in the EMR, presenting a good adjustment to experimental data.The analysis of Y behavior in Fig. 2 shows that:Y in Experiment 2 is greater than Experiment 1 for both EDCs, indicating that the concentration of active enzymes is higher in Experiment 2. Thus, it can be concluded that the O2 pulse rate of 0.5 h (Table 1, Experiment 2) provides greater enzyme activity than 1 h (Table 1, Experiment 1);For estrone and estradiol, for low reaction times, Y in Experiment 2 is higher than in Experiment 3. This occurs because the substrate concentration in the reactor increases faster to low τ, resulting in a higher consumption rate;For estrone and estradiol, for high reaction times, Y in Experiment 3 is higher than in Experiment 2. This occurs since, for high τ, the permanence of the EDCs in the reactor is lower than for the lower τ, resulting in a higher substrate concentration at the EMR outflow.Based on the presented results, the best experimental conditions to degrade estrone and estradiol in the EMR were used in Experiment 3 (Table 1). However, it should be considered that, for the same amount of wastewater to be treated, the designed reactor volume for the experimental conditions of Experiment 3 will be twice the experimental conditions of Experiment 2.In wastewater plant design, two parameters that should be considered are the reactor cost and its performance. These design parameters are closely related to the methods and mathematical models proposed in this manuscript. So, this work can inform the design of wastewater treatment plants aiming to reduce EDCs in waterbodies.ConclusionThe results indicated the assessed kinetic models were able to represent the experimental data for estrone and estradiol degradation in the reactor. The best assessed models presented good adjustments to experimental data and parameter values with 95% of confidence according to Student's t-test.The percentage of degradation estimated for each experimental condition and the estimated values for the model parameter related to the enzyme activation/inactivation/loss indicated the O2 pulse rate of 0.5 h and a residence time of 4 h were the better experimental conditions to maximize degradation in the EMR.As seen in the models' plots and estimated parameter values, laccase has clearly different degradation and activity behaviors for estrone and estradiol. So, other conditions and processes may be evaluated to discover a feasible process for wastewater treatment. Therefore, the methods and models presented in this manuscript are potentially useful to these future works.References1. Godfray HCJ, Stephens AE, Jepson PD, et al. A Restatement of the natural science evidence base on the effects of endocrine disrupting chemicals on wildlife. Proc R Soc B 2019;286(1897):20182416. Crossref, Medline, Google Scholar2. Zhang F, Yu Q, Yang L. Watershed scale patterns in steroid hormones composition and content characters at a typical eutrophic lake in southeastern China. Environ Sci Pollut Res 2019;26(6):6107–6115. Crossref, Medline, Google Scholar3. Cunha DL, Silva SMC, Bila DM, et al. Regulamentac¸ao do Estrogenio Sintetico 17´ α-etinilestradiol em Matrizes aquaticas na Europa, Estados Unidos e Brasil. Cad Saude Publica 2016;32:e00056715. Crossref, Medline, Google Scholar4. Reis Filho RW, Araujo J, Vieira EM. Hormonios Sexuais Estrogenos: Contaminantes Bioativos. Quim Nova 2006;29(4):817–822. Crossref, Google Scholar5. Riva S. Laccases: Blue enzymes for green chemistry. Trends Biotechnol 2006;24(5):219–226. Crossref, Medline, Google Scholar6. Couto SR, Herrera JLT. Industrial and biotechnological applications of laccases: A review. Biotechnol Adv 2006;24(5):500–513. Crossref, Medline, Google Scholar7. Chen PC, Ma Z, Zhu XY, et al. Fabrication and optimization of a lipase immobilized enzymatic membrane bioreactor based on polysulfone gradient-pore hollow fiber membrane. Catalysts 2019;9(6):495. Crossref, Google Scholar8. Lloret L, Eibes G, Feijoo G, et al. Degradation of estrogens by laccase from Myceliophthora thermophila in fed-batch and enzymatic membrane reactors. J Hazard Mater 2012;213:175–183. Crossref, Medline, Google Scholar9. Lindeque RM, Woodley JM. Reactor selection for effective continuous biocatalytic production of pharmaceuticals. Catalysts 2019;9(3):262. Crossref, Google Scholar10. Frasca V. Using isothermal titration calorimetry techniques to quantify enzyme kinetics. Ind Biotechnol 2016;12(4):207–211. Link, Google Scholar11. Bajwa PK, Harrington S, Dashtban M, et al. Expression and characterization of glycosyl hydrolase family 115 α-glucuronidase from Scheffersomyces stipitis. Ind Biotechnol 2016;12(2):98–104. Link, Google Scholar12. Sunwoo I, Kwon JE, Jeong GT, et al. Optimization of hyper-thermal acid hydrolysis and enzymatic saccharification of Ascophyllum nodosum for ethanol production with mannitol-adapted yeasts. Bioprocess Biosyst Eng 2019;42(8):1–8. Medline, Google Scholar13. Pereira FM, Oliveira SC. Occurrence of dead core in catalytic particles containing immobilized enzymes: analysis for the Michaelis–Menten kinetics and assessment of numerical methods. Bioprocess Biosyst Eng 2016;39(11):1717–1727. Crossref, Medline, Google Scholar14. Tavares B, de Almeida Felipe MdG, dos Santos JC, et al. An experimental and modeling approach for ethanol production by Kluyveromyces marxianus in stirred tank bioreactor using vacuum extraction as a strategy to overcome product inhibition. Renew Energ 2019;131:261–267. Crossref, Google Scholar15. Pereira FM, Loures CC, Amaral MS, et al. Evaluation of fatty acids production by Chlorella minutissima in batch bubble-column photobioreactor. Fuel 2018;230:155–162. Crossref, Google Scholar16. Ingdal M, Johnsen R, Harrington DA. The Akaike information criterion in weighted regression of immittance data. Electrochim Acta 2019;317:648–653. Crossref, Google Scholar17. Cavanaugh JE, Neath AA. The Akaike information criterion: Background, derivation, properties, application, interpretation, and refinements. Wiley Interdiscip Rev Comput Stat 2019;11(3):e1460. Crossref, Google Scholar18. Al-Rubaie KS, Godefroid LB, Lopes JA. Statistical modeling of fatigue crack growth rate in Inconel alloy 600. Int J Fatigue 2007;29(5):931–940. Crossref, Google Scholar19. Gomes FM, Pereira FM, Silva AF, et al. Multiple response optimization: Analysis of genetic programming for symbolic regression and assessment of desirability functions. Knowl Based Syst 2019;179:21–33. Crossref, Google ScholarFiguresReferencesRelatedDetails Volume 16Issue 2Apr 2020 InformationCopyright 2020, Mary Ann Liebert, Inc., publishersTo cite this article:Camila L. S. Carvalho, Fabrício M. Gomes, and Félix M. Pereira.Mathematical Modeling of Estrogen Degradation in Enzymatic Membrane Reactor.Industrial Biotechnology.Apr 2020.50-55.http://doi.org/10.1089/ind.2020.29200.cscPublished in Volume: 16 Issue 2: April 17, 2020PDF download
更多查看译文
AI 理解论文
溯源树
样例
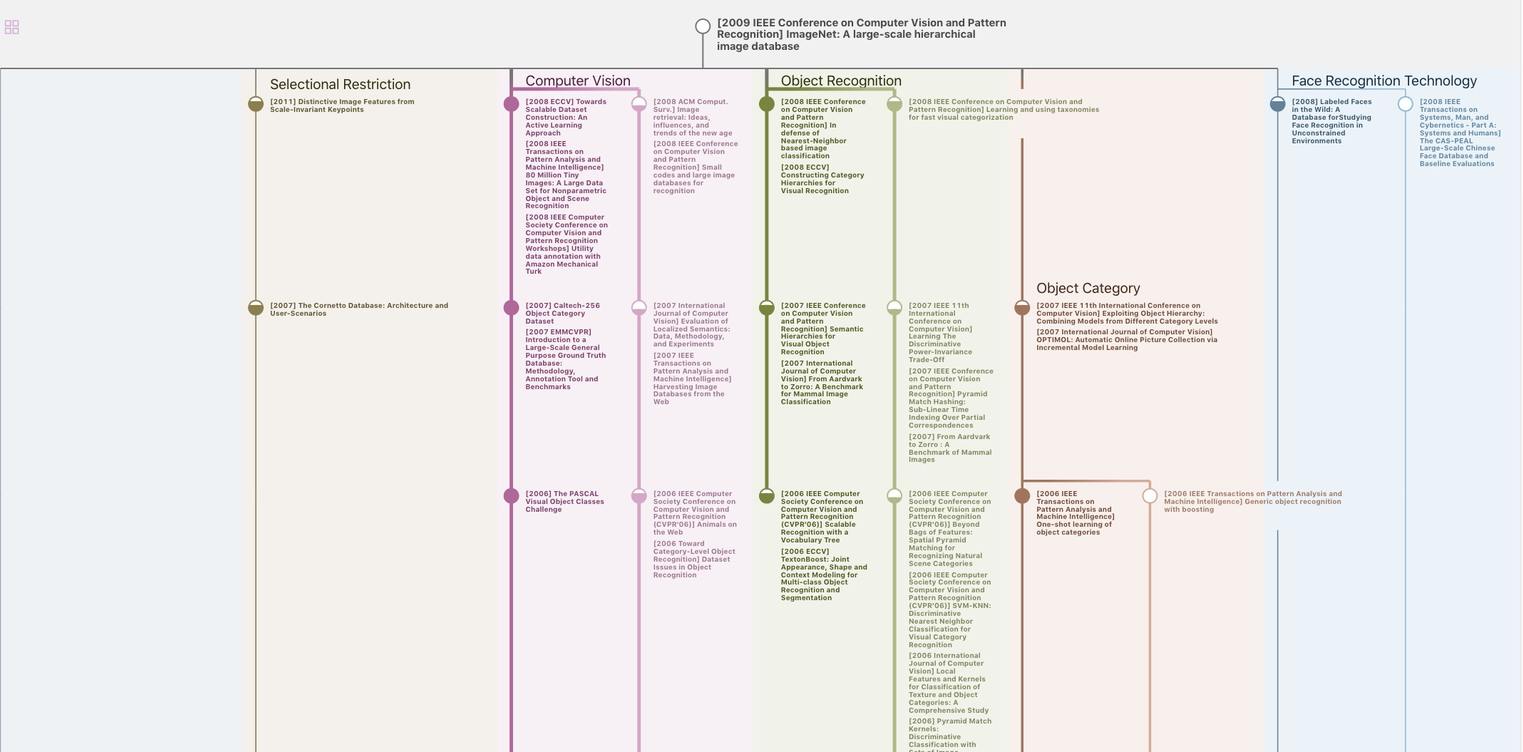
生成溯源树,研究论文发展脉络
Chat Paper
正在生成论文摘要