Bypassing MRI Pre-processing in Alzheimer's Disease Diagnosis using Deep Learning Detection Network
2020 16th IEEE International Colloquium on Signal Processing & Its Applications (CSPA)(2020)
摘要
Although promising results have been achieved in the area of Alzheimer's Disease diagnosis via hippocampal atrophy analysis, most of these solutions are heavily dependent on various MRI pre-processing techniques to obtain a good result. Besides, most recent works using deep learning methods such as Convolutional Neural Network (CNN) solve Alzheimer's Disease diagnosis based on a classification problem, leaving a research gap to use deep learning object detection method on Alzheimer's Disease diagnosis. In this study, we make two contributions to solve this problem. Firstly, we are the first group to propose an Alzheimer's Disease diagnosis solution without requiring any MRI pre-processing technique. Secondly, we introduce recent deep learning object detection architectures such as Faster R-CNN, SSD and YOLOv3 into the area of Alzheimer's Disease diagnosis. As a side product of our research, we provide a new Deep Learning Alzheimer's Disease/Normal Control (AD/NC) object detection benchmark dataset which includes 500 raw, unprocessed screening instances per class from Alzheimer's Disease Neuroimaging Initiative (ADNI) dataset for future object detection research. This dataset consists of a collection of T1 weighted sagittal MRI dicom slices in MP-Rage series in DICOM 16-bit and PNG 16-bit image format, annotated with their respective class label and bounding box in Pascal VOC format. We call this benchmark dataset UTM-ADNI-RAW*. In this study, without using any MRI preprocessing technique, we managed to obtain a detection accuracy of 0.998 for YOLOv3, 0.982 for SSD and 0.988 for Faster R-CNN in AD/NC territory while surpassing 0.75 IoU threshold across all three deep learning architectures.
更多查看译文
关键词
Alzheimer' ‘s Disease,MRI,Deep Learning,CNN,Object Detection,UTM-ADNI-RAW
AI 理解论文
溯源树
样例
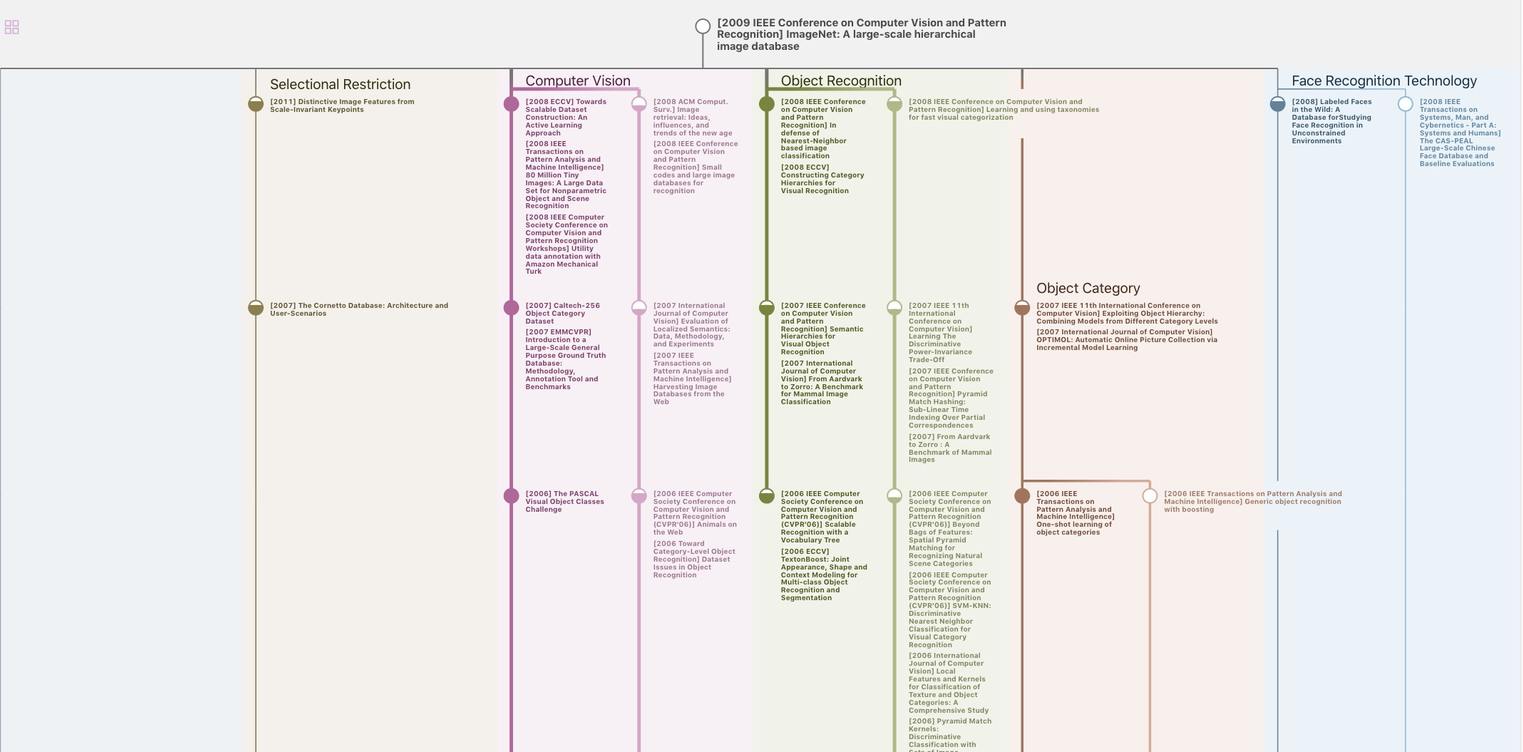
生成溯源树,研究论文发展脉络
Chat Paper
正在生成论文摘要