Deep Adversarial Imitation Learning Of Locomotion Skills From One-Shot Video Demonstration
ieee international conference on cyber technology in automation control and intelligent systems(2019)
Abstract
Traditional imitation learning approaches usually collect demonstrations by teleoperation, kinesthetic teaching or precisely calibrated motion capture devices. These teaching interfaces are cumbersome and subject to the constraints of the environment and robot structures. Learning from observation adopts the idea that the robot can learn skills by observing human's behaviors, which is more convenient and preferable. However, learning from observation shows great challenges since it involves understanding of the environment and human actions, as well as solving the retarget problem. This paper presents a way to learn locomotion skills from a single video demonstration. We first leverage a weak supervised method to extract the pose feature from the experts, and then learn a joint position controller trying to match this feature by using the general adversarial network (GAN). This approach avoids cumbersome demonstrations, and more importantly, GAN can generalize learned skills to different subjects. We evaluated our method on a walking task executed by a 56 -degree-of-freedom (DOE) humanoid robot. The experiment demonstrate that the vision -based imitation learning algorithm can be applied to high -dimensional robot task and achieve comparable performance to methods by using finely calibrated motion capture data, which are of great significance for the research on human -robot interaction and robot skill acquisition.
MoreTranslated text
Key words
imitation learning, GAN, pose estimation, locomotion skills
AI Read Science
Must-Reading Tree
Example
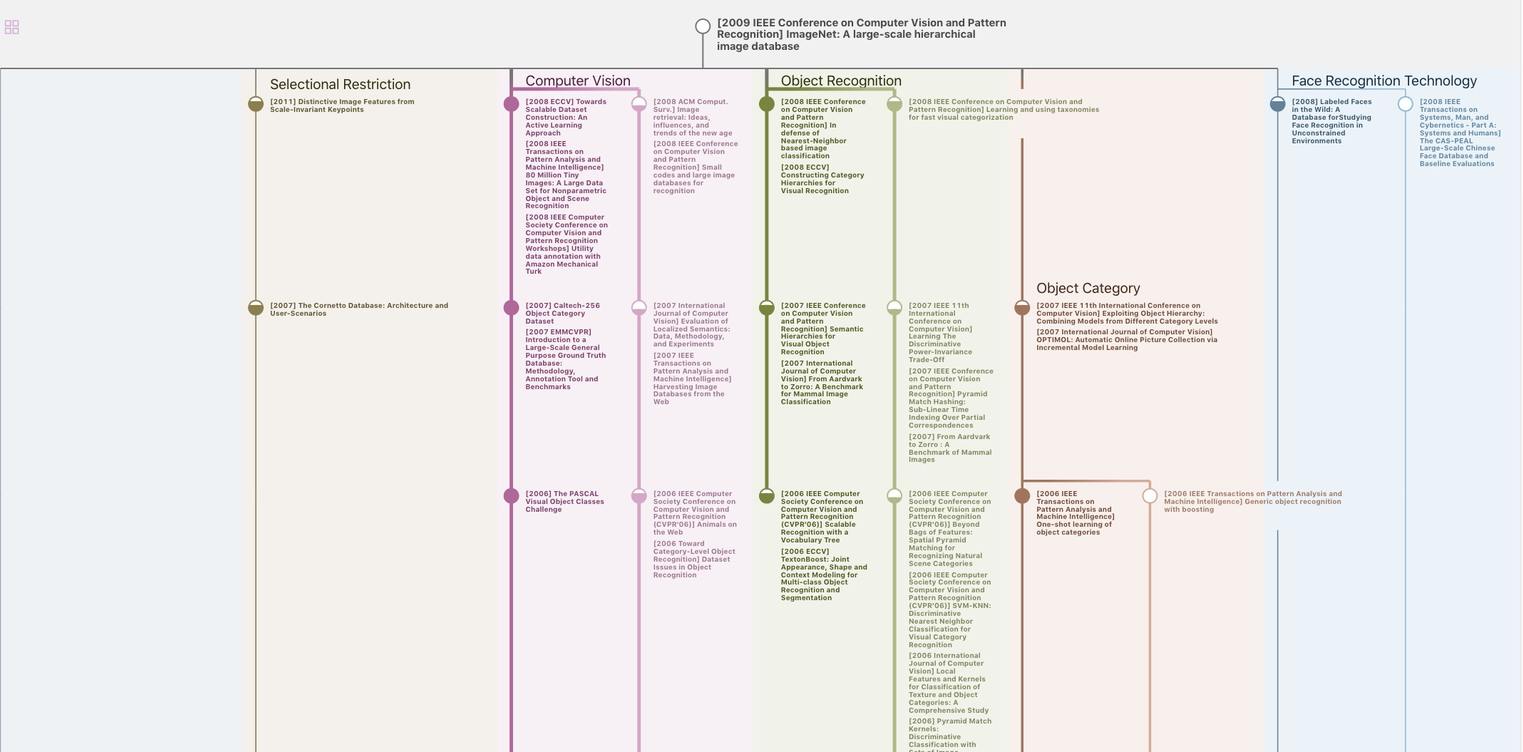
Generate MRT to find the research sequence of this paper
Chat Paper
Summary is being generated by the instructions you defined