Lock-Free Parallelization for Variance-Reduced Stochastic Gradient Descent on Streaming Data
IEEE Transactions on Parallel and Distributed Systems(2020)
摘要
Stochastic Gradient Descent (SGD) is an iterative algorithm for fitting a model to the training dataset in machine learning problems. With low computation cost, SGD is especially suited for learning from large datasets. However, the variance of SGD tends to be high because it uses only a single data point to determine the update direction at each iteration of gradient descent, rather than all avai...
更多查看译文
关键词
Computational modeling,Machine learning,Data models,Training data,Multicore processing,Training,Mathematical model
AI 理解论文
溯源树
样例
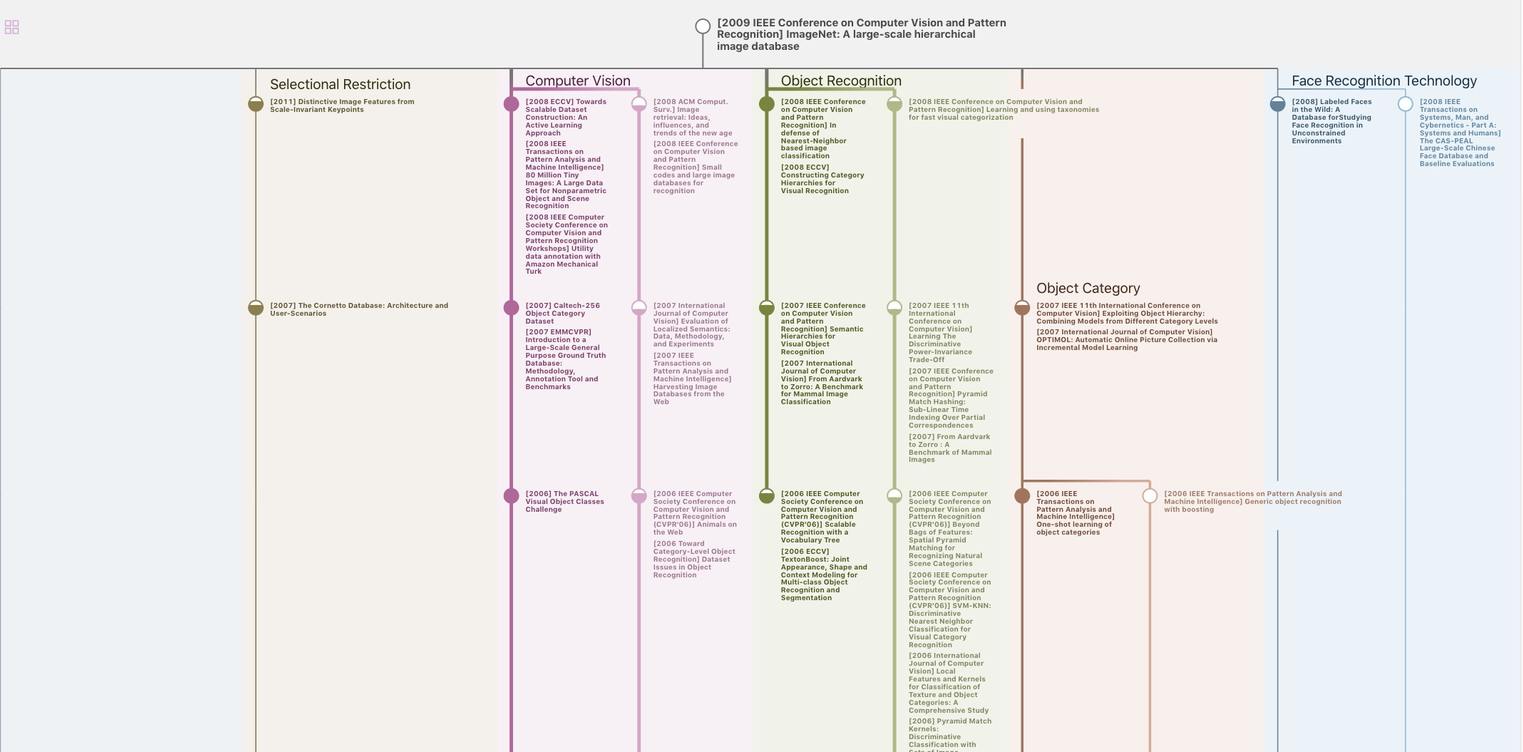
生成溯源树,研究论文发展脉络
Chat Paper
正在生成论文摘要