Generalized Integration Model for Improved Statistical Inference by Leveraging External Summary Data
BIOMETRIKA(2017)
摘要
Meta-analysis has become a powerful tool for improving inference by gathering evidence from multiple sources. It pools summary-level data from different studies to improve estimation efficiency with the assumption that all participating studies are analysed under the same statistical model. It is challenging to integrate external summary data calculated from different models with a newly conducted internal study in which individual-level data are collected. We develop a novel statistical inference framework that can effectively synthesize internal and external data for the integrative analysis. The new framework is versatile enough to assimilate various types of summary data from multiple sources. We establish asymptotic properties for the proposed procedure and prove that the new estimate is theoretically more efficient than the internal data based maximum likelihood estimate, as well as a recently developed constrained maximum likelihood approach that incorporates the external information. We illustrate an application of our method by evaluating cervical cancer risk using data from a large cervical screening program.
更多查看译文
关键词
Constraint maximum likelihood estimate, Empirical likelihood, Estimating equation, Lagrange multiplier, Meta-analysis
AI 理解论文
溯源树
样例
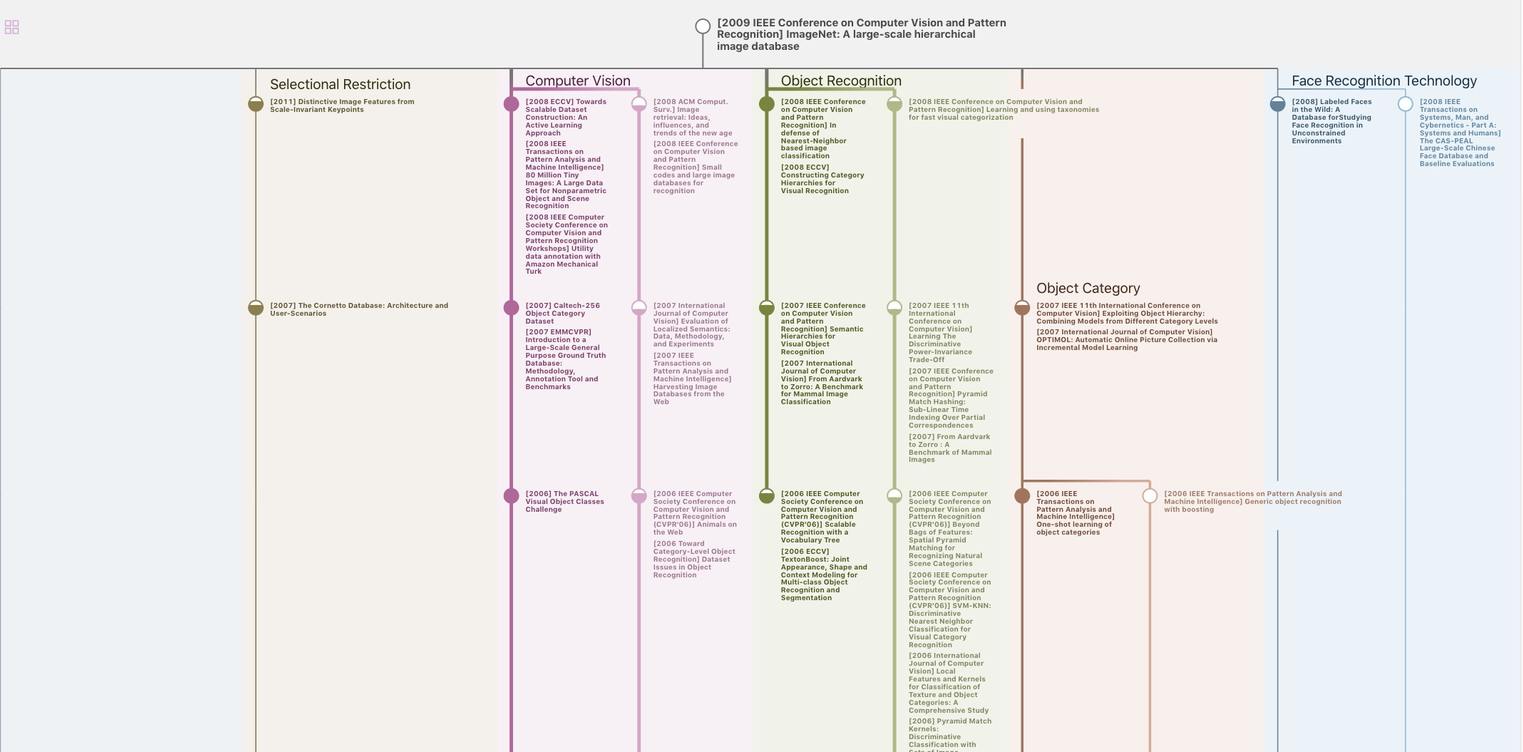
生成溯源树,研究论文发展脉络
Chat Paper
正在生成论文摘要