Mixture Density-PoseNet and its Application to Monocular Camera-Based Global Localization
IEEE Transactions on Industrial Informatics(2021)
摘要
Global localization using a monocular camera is one of the most challenging problems in computer vision and intelligent robotics. In this article, a new deep neural network named Mixture Density (MD)-PoseNet is proposed to address this problem. Unlike existing learning-based global localization methods that return a single guess for the camera pose, MD-PoseNet returns multiple guesses represented in the form of a Gaussian mixture (GM). The key idea of MD-PoseNet is that the network returns the distribution of all probable camera poses instead of the most probable camera pose, and the distribution represents the multiple guesses for the camera pose. The multiple guesses returned by MD-PoseNet are, consequently, exploited in the probabilistic framework of particle filters. Finally, the proposed method is applied to four different environments, and its validity is demonstrated via experiments.
更多查看译文
关键词
CNN,distribution,Gaussian mixture,mixture density,particle filter
AI 理解论文
溯源树
样例
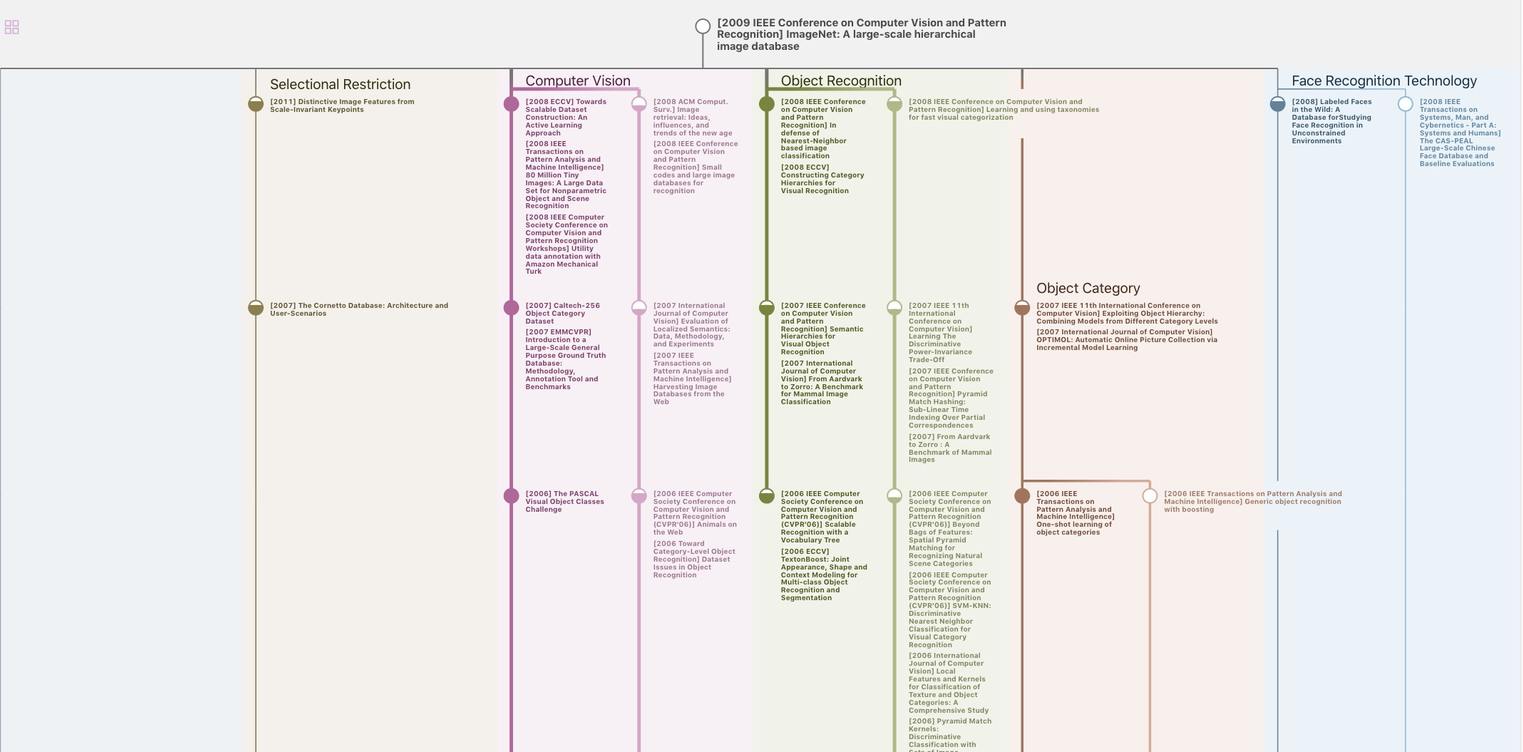
生成溯源树,研究论文发展脉络
Chat Paper
正在生成论文摘要