Formulating Divergence Framework For Multiclass Motor Imagery Eeg Brain Computer Interface
2020 IEEE INTERNATIONAL CONFERENCE ON ACOUSTICS, SPEECH, AND SIGNAL PROCESSING(2020)
摘要
The ubiquitous presence of non-stationarities in the EEG signals significantly perturb the feature distribution thus deteriorating the performance of Brain Computer Interface. In this work, a novel method is proposed based on Joint Approximate Diagonalization (JAD) to optimize stationarity for multiclass motor imagery Brain Computer Interface (BCI) in an information theoretic framework. Specifically, in the proposed method, we estimate the subspace which optimizes the discriminability between the classes and simultaneously preserve stationarity within the motor imagery classes. We determine the subspace for the proposed approach through optimization using gradient descent on an orthogonal manifold. The performance of the proposed stationarity enforcing algorithm is compared to that of baseline One-Versus-Rest (OVR)-CSP and JAD on publicly available BCI competition IV dataset IIa. Results show that an improvement in average classification accuracies across the subjects over the baseline algorithms and thus essence of alleviating within session non-stationarities.
更多查看译文
关键词
Brain Computer Interface (BCI), Electroencephalogram (EEG), Motor Imagery (MI), Divergence, Common Spatial Patterns (CSP), Joint Approximate Diagonalization (JAD), Information theoretic (IT)
AI 理解论文
溯源树
样例
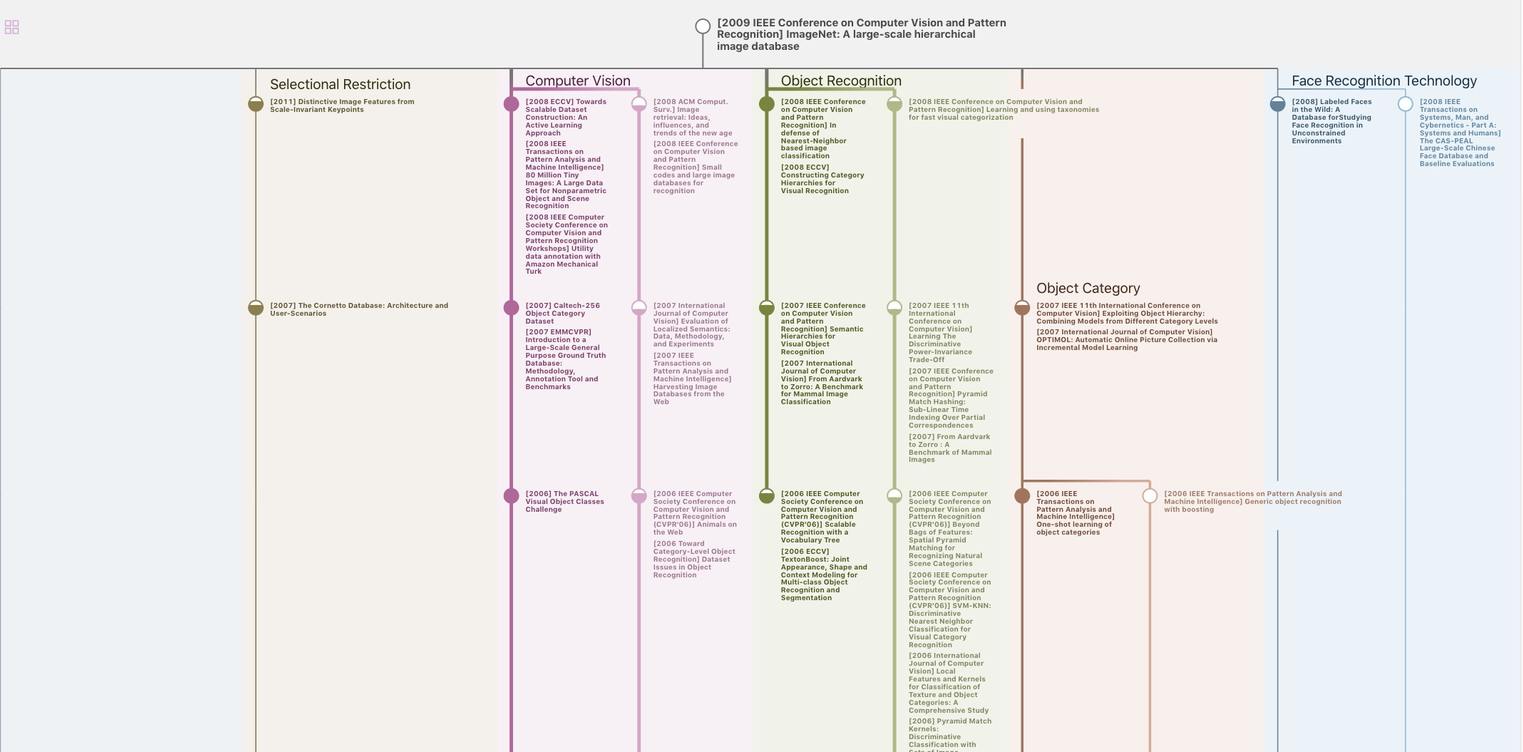
生成溯源树,研究论文发展脉络
Chat Paper
正在生成论文摘要