Fully Convolutional Recurrent Networks For Speech Enhancement
2020 IEEE INTERNATIONAL CONFERENCE ON ACOUSTICS, SPEECH, AND SIGNAL PROCESSING(2020)
摘要
Convolutional recurrent neural networks (CRNs) using convolutional encoder-decoder (CED) structures have shown promising performance for single-channel speech enhancement. These CRNs handle temporal modeling through integrating long short-term memory (LSTM) layers in between convolutional encoder and decoder. However, in such a CRN, the organization of internal representations in feature maps and the focus on local structure of the convolutional mappings has to be discarded for fully-connected LSTM processing. Furthermore, CRNs can be quite restricted concerning the feature space dimension at the input of the LSTM, which, through its fully-connected nature, requires a large amount of trainable parameters. As first novelty, we propose to replace the fully-connected LSTM by a convolutional LSTM (ConvLSTM) and call the resulting network a fully convolutional recurrent network (FCRN). Secondly, since the ConvLSTM retains the structured organization of its input feature maps, we can show that this helps to internally represent the harmonic structure of speech, allowing us to handle high-dimensional input features using less trainable parameters than an LSTM. The proposed FCRN clearly outperforms CRN reference models with similar amounts of trainable parameters in terms of PESQ, STOI, and segmental Delta SNR.
更多查看译文
关键词
Speech enhancement, convolutional recurrent neural networks, convolutional LSTM
AI 理解论文
溯源树
样例
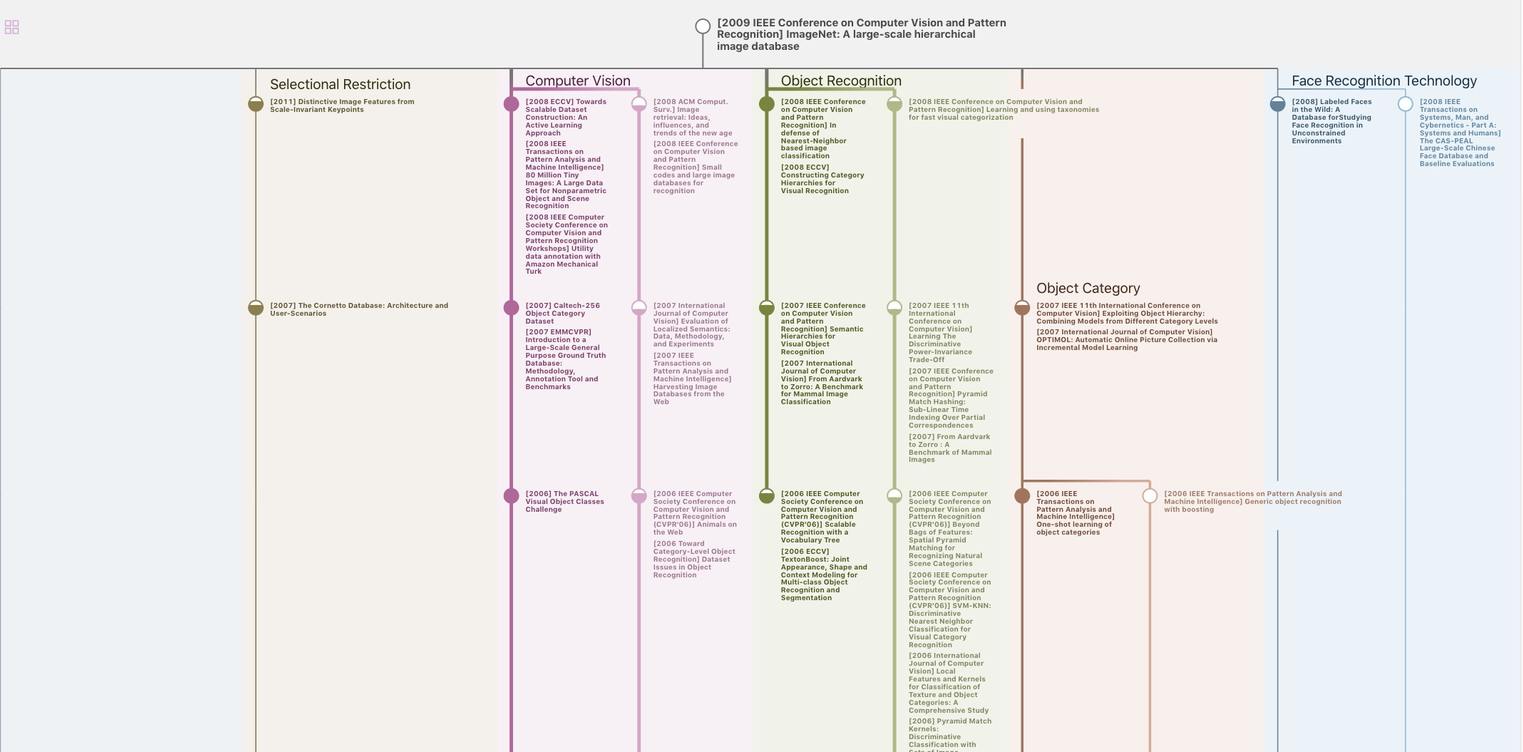
生成溯源树,研究论文发展脉络
Chat Paper
正在生成论文摘要