Deploying Network Functions for Multi-Access Edge-IoT with Deep Reinforcement Learning
IEEE Internet of Things Journal(2020)
摘要
Edge computing is a promising technology to empower the Internet of Things (IoT) by providing additional processing ability, where the tasks can be offloaded to the edge servers for efficient computing. To enable easy management and improve the resource utilization of edge computing, network function virtualization (NFV) is often employed to quickly deploy the requested network functions (NFs) for serving front-end devices. Considering that the edge servers are close to the front-end users, the time-varying geographical distribution of users has a significant influence on the NF deployment in the network of edge servers. Based on this observation, we propose a deep-reinforcement-learning (DRL)-based approach for the NF deployment, which has the following two salient features. First, we employ DRL to estimate the underlying wireless features affected by user mobility, which has a direct impact on the task performance in the edge-IoT systems. Second, we implicitly utilize the multiaccess opportunity to deploy the NFs, considering the estimated features and user requests. Compared to the existing works, the proposed work can significantly improve the resource utilization of edge servers as well as the computational efficiency of IoT tasks. The evaluation results obtained from extensive simulation experiments show that our approach can be well adapted to the time-varying user geodistributions and achieve improved performance in terms of both resource utilization of edge servers and task executions of the IoT devices.
更多查看译文
关键词
Noise measurement,Servers,Delays,Image edge detection,Task analysis,Resource management,Wireless communication
AI 理解论文
溯源树
样例
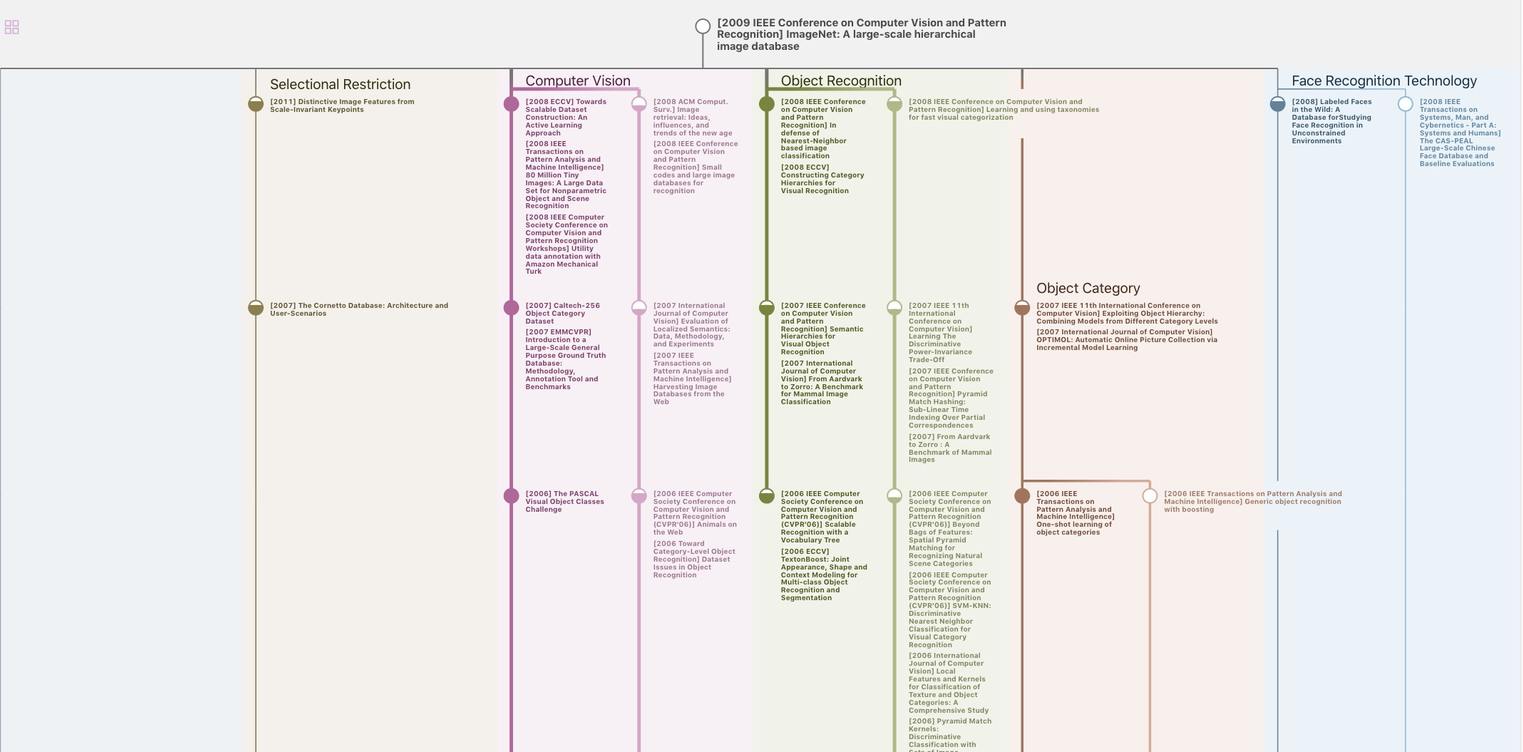
生成溯源树,研究论文发展脉络
Chat Paper
正在生成论文摘要