A random forest model of landslide susceptibility mapping based on hyperparameter optimization using Bayes algorithm
Geomorphology(2020)
摘要
The choice of model parameters in landslide susceptibility mapping makes a major determinant of model accuracy. The purpose of this study is to optimize the hyperparameters based on a Bayesian optimization algorithm, and to obtain a high accuracy random forest landslide susceptibility evaluation model. The research steps are detailed as follows. Firstly, taking a typical landslide prone mountainous area as an example, 16 conditioning factors, such as elevation, annual average rainfall, distance from roads, distance from buildings and so on, were preliminarily selected as the conditioning factors of landslide susceptibility. Combined with 1520 historical landslide events, a geospatial database was established with 30 m resolution. Secondly, the geospatial data sample set was constructed by random sampling according to ratio of historical landslides and non-landslides of 1:10. Based on the whole sample set, the random forest model adopted the Bayesian optimization algorithm to optimize the hyperparameters. Next, the optimal hyperparameters were selected to be trained to get the evaluation model of landslide susceptibility. In addition, they were carried out the analysis of landslide susceptibility mapping for the whole study area. After that, the recursive feature elimination method was used to screen out the dominant conditioning factors that can explain the degree of landslide susceptibility. The results indicated that the area under curve (AUC) values of receiver operating characteristic (ROC) curve in training data set, verification data set and regional simulation were 0.95, 0.87 and 0.93, respectively. 65% of the historical landslides fell between the high susceptibility and very high susceptibility regions, which made up <20% of the research area. The model was in good agreement to the distribution characteristics of historical landslides in the study area. We noted that all the three recent landslides with impact on the study area occurred at the locations predicted by the model to have high or very high susceptibility in terms of typical landslides in the near future. As for conditioning factors, the contribution related to human activities accounted for a large proportion. In conclusion, an evaluation model with high precision for random forest landslide susceptibility can be built based on hyperparameter optimization with Bayesian optimization algorithm. Simultaneously, using recursive feature elimination method, a random forest landslide susceptibility model with fewer dominant conditioning factors and guaranteed evaluation accuracy can also be built to save the running time and input data resources of the model.
更多查看译文
关键词
Bayes algorithm,Random forest,Landslide susceptibility mapping,Hyperparameter optimization,Factor screening
AI 理解论文
溯源树
样例
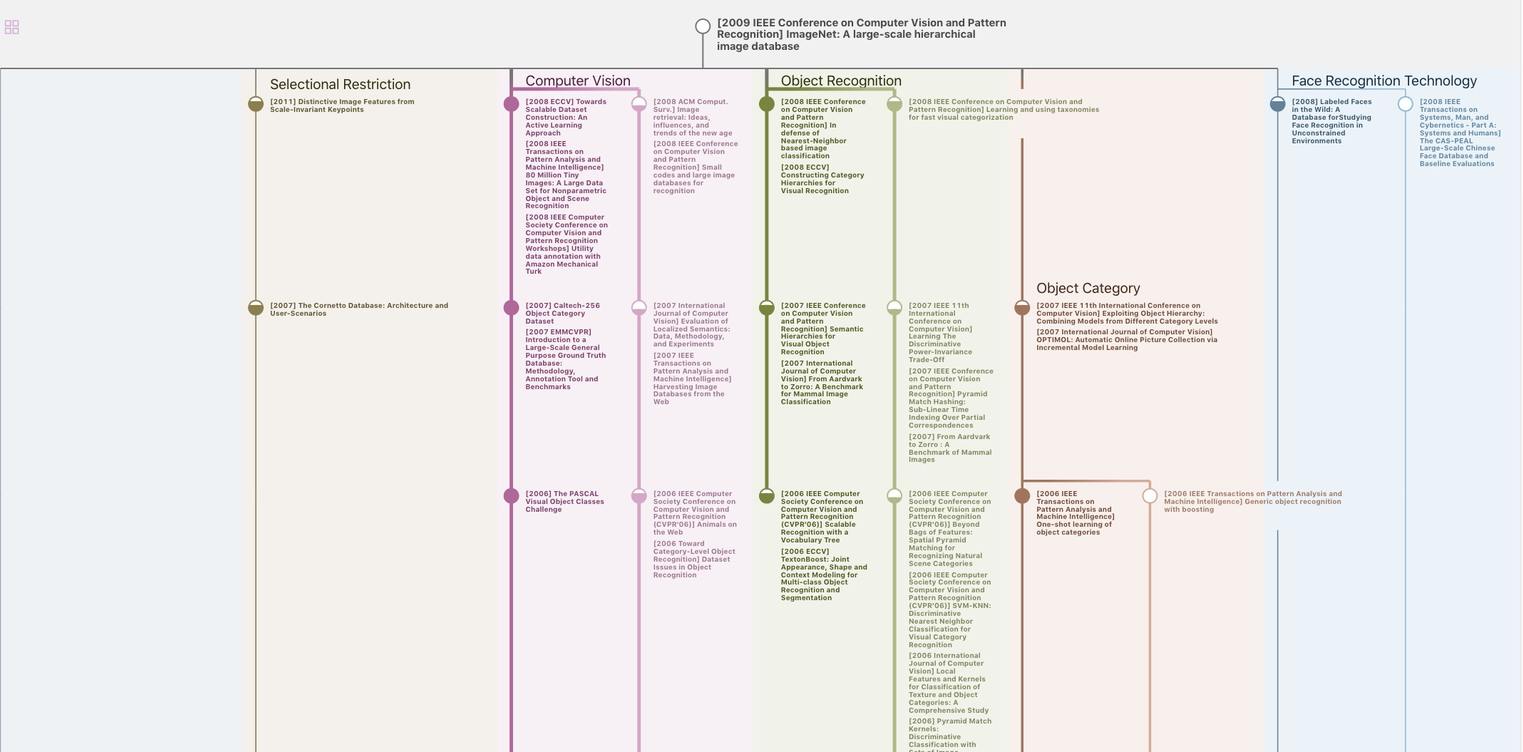
生成溯源树,研究论文发展脉络
Chat Paper
正在生成论文摘要