Sdtcn: Similarity Driven Transmission Computing Network For Image Dehazing
2020 IEEE INTERNATIONAL CONFERENCE ON ACOUSTICS, SPEECH, AND SIGNAL PROCESSING(2020)
Abstract
Transmission similarity is an important feature which can greatly increase the capability of convolutional neural network (CNN) to fit transmission map. However, it is not sufficiently utilized in existing algorithms. In this paper, we propose a novel light-weight similarity driven transmission computing network called SDTCN that is guided by the attributes of transmission similarity. First, we adopt a non-data-driven image segmentation method to acquire the transmission similarity. Compared with CNN based segmentation approaches, our method can not only greatly save computing resources, but also separate the objects and background precisely. Second, a full convolutional network is introduced to reduce blocky effects in SDTCN. Finally, unlike previous "first airlight then transmission" mode, a dependable airlight estimation approach is designed drawing on the transmission map generated by SDTCN, which can improve the accuracy of airlight effectively. Extensive experiments demonstrate that the proposed algorithm outperforms the state-of-the-art methods on synthetic and real-world images.
MoreTranslated text
Key words
Image restoration, image dehazing, CNN, transmission similarity, airlight estimation
AI Read Science
Must-Reading Tree
Example
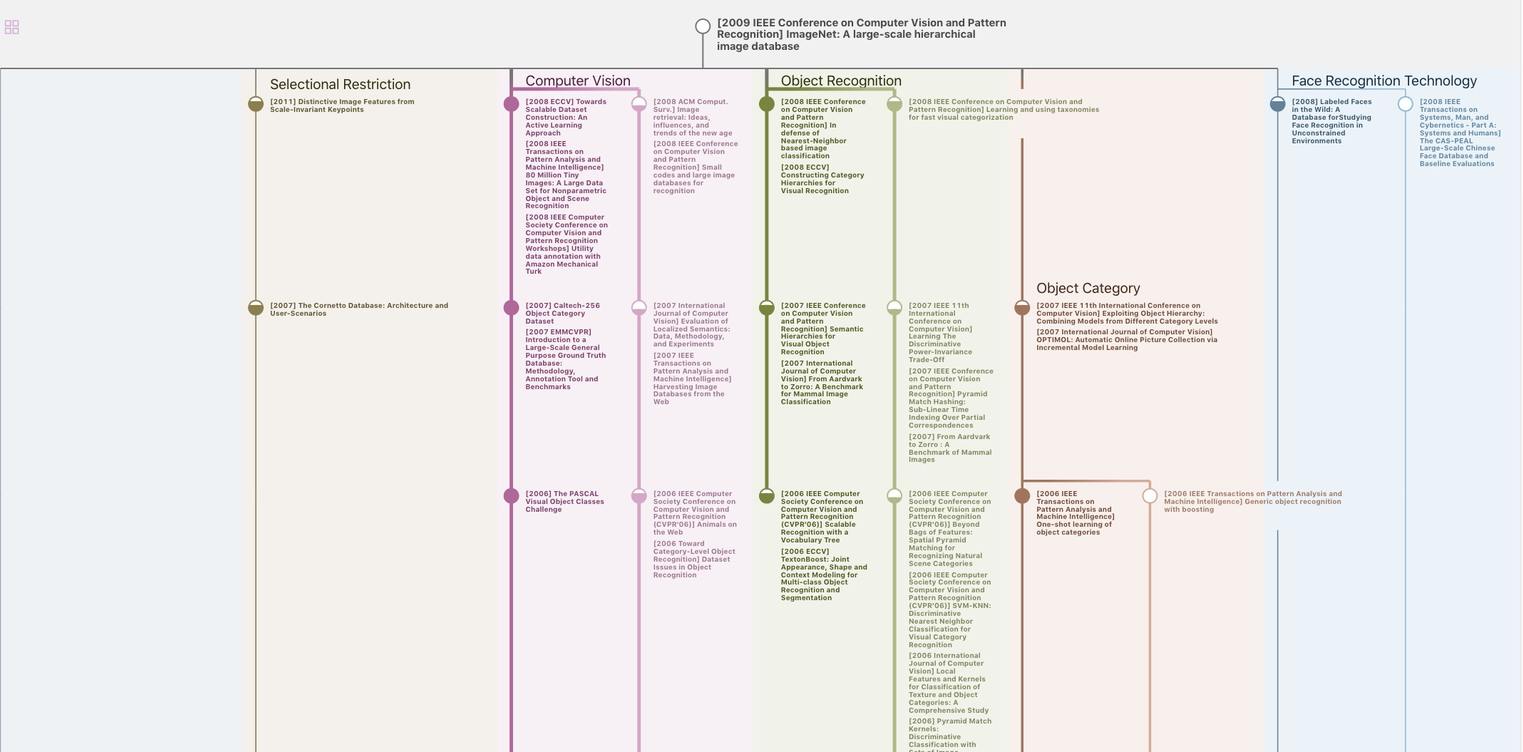
Generate MRT to find the research sequence of this paper
Chat Paper
Summary is being generated by the instructions you defined