Multi-Label Consistent Convolutional Transform Learning: Application To Non-Intrusive Load Monitoring
2020 IEEE INTERNATIONAL CONFERENCE ON ACOUSTICS, SPEECH, AND SIGNAL PROCESSING(2020)
摘要
Convolutional transform learning is an unsupervised framework we introduced recently, for feature generation based on learnt convolutions. In this work, we propose a supervised formulation for convolutional transform so as to address the multi-label classification problem. Unlike the simple multiclass classification, in multi-label problems, each sample can correspond to multiple classes simultaneously, making the problem quite challenging. We propose to make use of a label consistency penalty and develop a suitable minimization algorithm for the training step. We illustrate the performance of the developed formulation on the practical problem of non-intrusive load monitoring. Comparisons with popular techniques show that our proposed approach yields better results on benchmark datasets.
更多查看译文
关键词
Convolutive dictionary, transform learning, multi-label classification, proximal alternating method, NILM, energy disaggregation
AI 理解论文
溯源树
样例
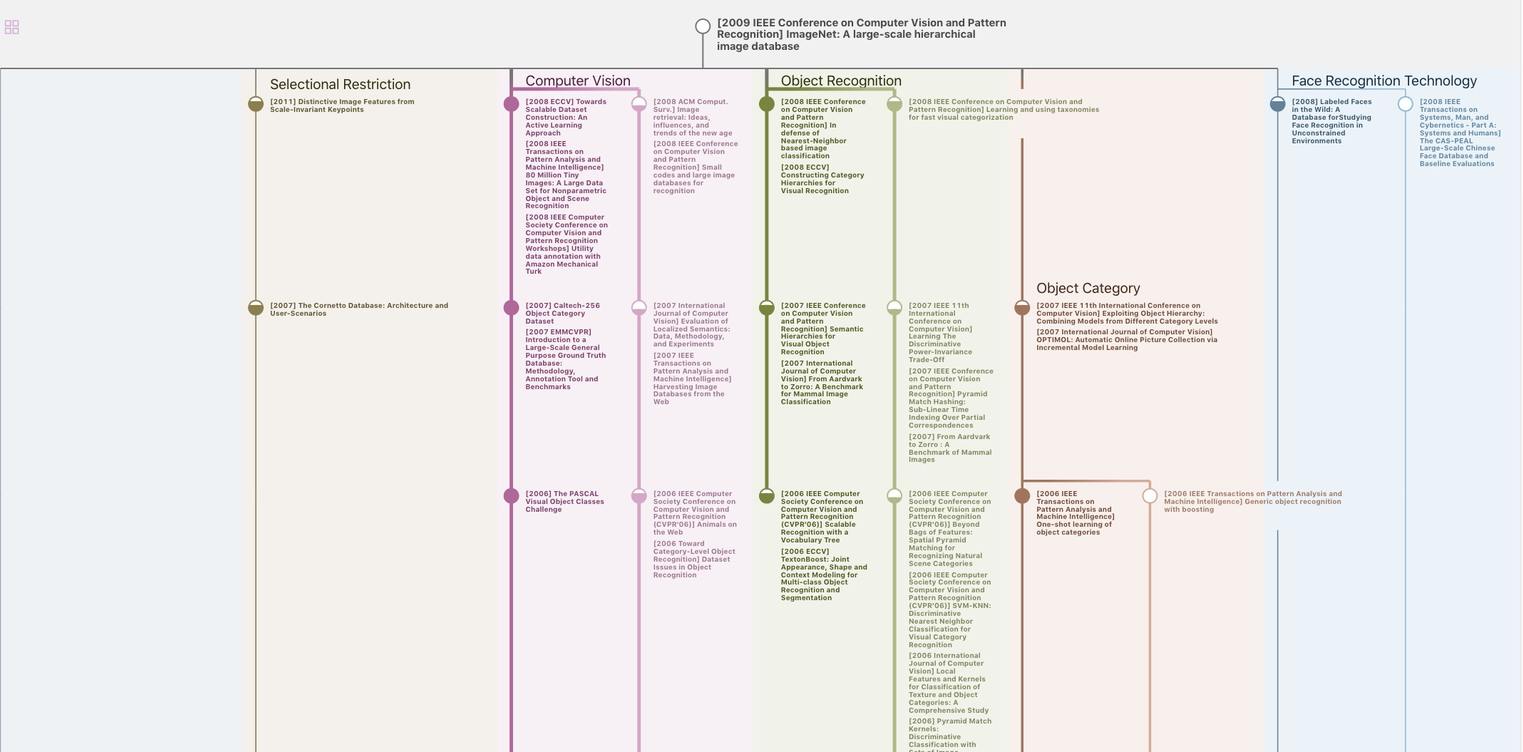
生成溯源树,研究论文发展脉络
Chat Paper
正在生成论文摘要