Spatio-temporal and geometry constrained network for automobile visual odometry
2020 IEEE INTERNATIONAL CONFERENCE ON ACOUSTICS, SPEECH, AND SIGNAL PROCESSING(2020)
Abstract
Visual odometry (VO) is an essence of vision-based localization and mapping system where existing learning-based approaches utilize CNN and RNN to model camera motion and gain promising results. However, these methods lack full use of the relationship between spatial characteristics and temporal clues, as well as geometry constraints in VO. To overcome these deficiencies, an end-to-end framework that leverages spatio-temporal relevance and geometrical knowledge is proposed. In particular, a spatial response module (SRM) is designed to extract the visual motion features by emphasizing the most interconnected regions while suppressing the irrelevant areas. A module named temporal response module (TRM) is used to regress the camera motion via adopting the optimal motion features. Moreover, a geometry constrained (GC) loss that minimizes the estimated inter-frame pose errors and the accumulated pose errors within a local period is introduced. Actually, the GC loss utilizes adaptive learnable balance factors for balancing losses. Experimental results on KITTI and Malaga datasets demonstrate that the proposed model outperforms state-of-the-art monocular methods.
MoreTranslated text
Key words
Visual Odometry,Spatio-Temporal Relevance,Geometry Constrained Loss
AI Read Science
Must-Reading Tree
Example
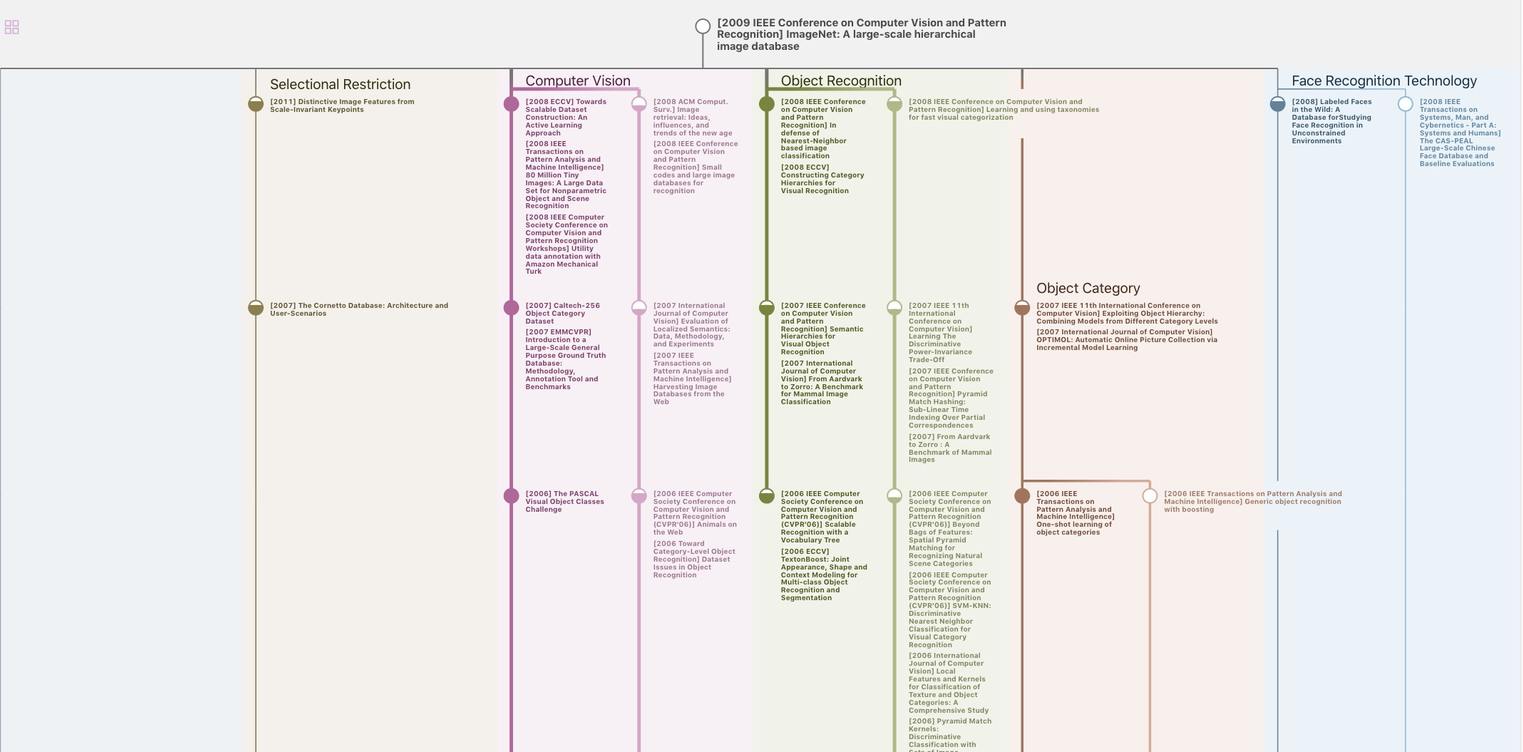
Generate MRT to find the research sequence of this paper
Chat Paper
Summary is being generated by the instructions you defined