Task-Oriented Deep Network For Ischemic Stroke Segmentation In Unenhanced Ct Imaging
2019 IEEE NUCLEAR SCIENCE SYMPOSIUM AND MEDICAL IMAGING CONFERENCE (NSS/MIC)(2019)
摘要
In the clinics, unenhanced CT (unCT) are first used for acute ischemic stroke detection, but sometimes it is difficult to delineate acute infarction in the unCT in the first few hours. Currently, deep learning methods can be used for stroke segmentation, but they are based on a large amount of annotation data, especially in unCT imaging. Meanwhile, the Diffusionweighted magnetic resonance imaging (DWI) can clearly distinguish infarcted brain tissue from healthy region. In this work, we investigate whether external information, i.e., DWI images, can be used to help more clearly delineates acute infraction in the unCT images by a transfer learning network. We detail the network architectures in the transfer learning framework, and describe the training process used to transfer segmentation task in DWI images to unCT images. In particular, intermediate layers in the network trained with DWI images are frozen and then directly are transferred to the network trained with unCT task by parameter fine-tuning. The ISLES2018 dataset is used to validate and evaluate this task-oriented transfer network. Experimental results demonstrate that this taskoriented transfer network can obtain the dice coefficient with 0.793, which is much better than the network that only uses unCT input (dice coefficient: 0.570).
更多查看译文
关键词
task-oriented transfer network,transfer learning network,infarcted brain tissue,diffusion-weighted magnetic resonance imaging,deep learning methods,acute infarction,acute ischemic stroke detection,unenhanced CT imaging,ischemic stroke segmentation,task-oriented deep network
AI 理解论文
溯源树
样例
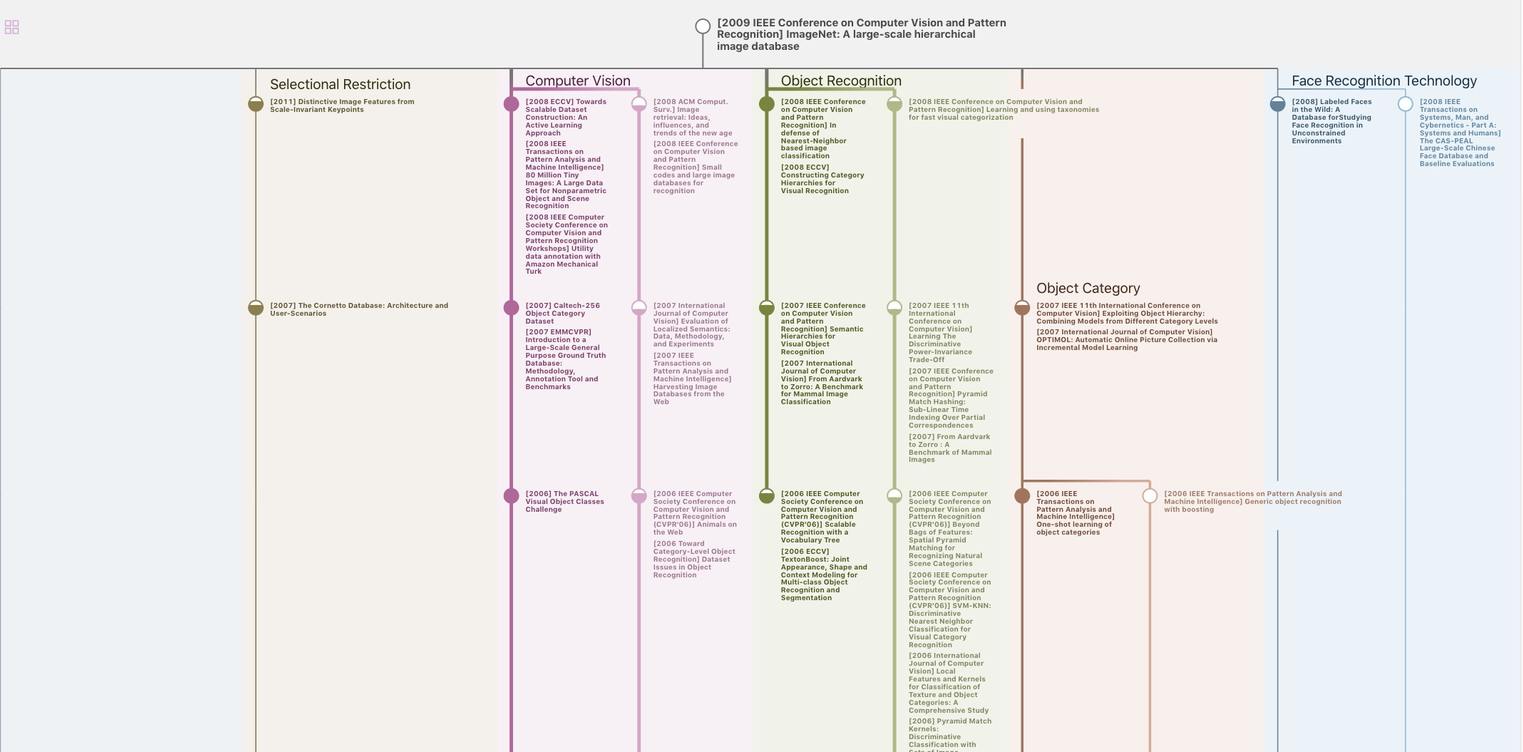
生成溯源树,研究论文发展脉络
Chat Paper
正在生成论文摘要