Mechanistically derived patient-level framework for precision medicine identifies a personalized immune prognostic signature in high-grade serous ovarian cancer
BRIEFINGS IN BIOINFORMATICS(2021)
摘要
An accurate prognosis assessment for cancer patients could aid in guiding clinical decision-making. Reliance on traditional clinical features alone in a complex clinical environment is challenging and unsatisfactory in the era of precision medicine; thus, reliable prognostic biomarkers are urgently required to improve a patient staging system. In this study, we proposed a patient-level computational framework from mechanistic and translational perspectives to establish a personalized prognostic signature (named PLPPS) in high-grade serous ovarian carcinoma (HGSOC). The PLPPS composed of 68 immune genes achieved accurate prognostic risk stratification for 1190 patients in the meta-training cohort and was rigorously validated in multiple cross-platform independent cohorts comprising 792 HGSOC patients. Furthermore, the PLPPS was shown to be the better prognostic factor compared with clinical parameters in the univariate analysis and retained a significant independent association with prognosis after adjusting for clinical parameters in the multivariate analysis. In benchmark comparisons, the performance of PLPPS (hazard ratio (HR), 1.371; concordance index (C-index), 0.604 and area under the curve (AUC), 0.637) is comparable to or better than other published gene signatures (HR, 0.972 to 1.340; C-index, 0.495 to 0.592 and AUC, 0.48-0.624). With further validation in prospective clinical trials, we hope that the PLPPS might become a promising genomic tool to guide personalized management and decision-making of HGSOC in clinical practice.
更多查看译文
关键词
immune, ovarian cancer, personalized signature, precision medicine
AI 理解论文
溯源树
样例
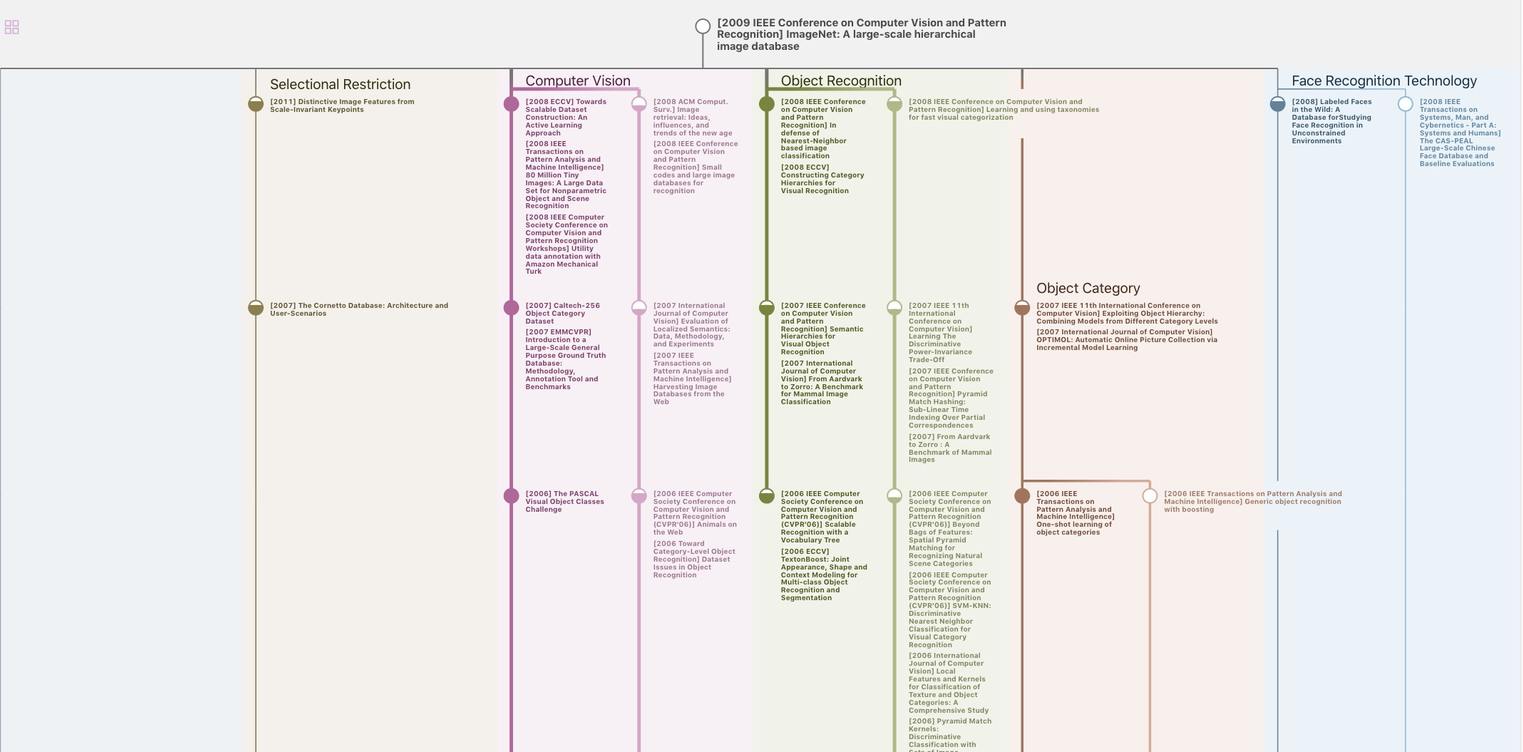
生成溯源树,研究论文发展脉络
Chat Paper
正在生成论文摘要