Structure prediction of surface reconstructions by deep reinforcement learning.
Journal of physics. Condensed matter : an Institute of Physics journal(2020)
摘要
We demonstrate how image recognition and reinforcement learning combined may be used to determine the atomistic structure of reconstructed crystalline surfaces. A deep neural network represents a reinforcement learning agent that obtains training rewards by interacting with an environment. The environment contains a quantum mechanical potential energy evaluator in the form of a density functional theory program. The agent handles the 3D atomistic structure as a series of stacked 2D images and outputs the next atom type to place and the atomic site to occupy. Agents are seen to require 1000-10 000 single point density functional theory evaluations, to learn by themselves how to build the optimal surface reconstructions of anatase TiO2(001)-(1 × 4) and rutile SnO2(110)-(4 × 1).
更多查看译文
关键词
reinforcement learning,global optimization,structure prediction,image recognition,chemical physics,surface reconstruction
AI 理解论文
溯源树
样例
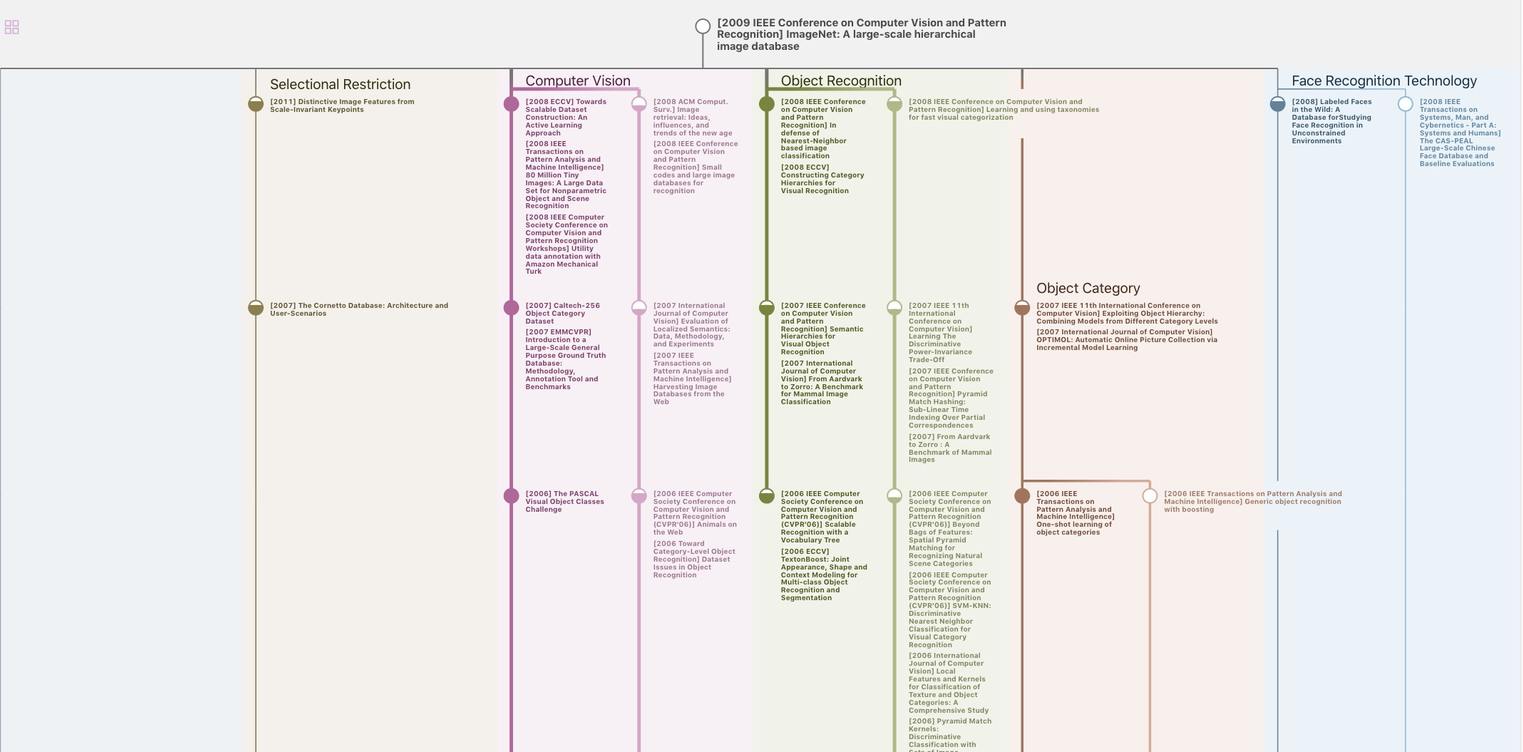
生成溯源树,研究论文发展脉络
Chat Paper
正在生成论文摘要