Improving Deep Learning Models via Constraint-Based Domain Knowledge: a Brief Survey
CoRR(2020)
Abstract
Deep Learning (DL) models proved themselves to perform extremely well on a wide variety of learning tasks, as they can learn useful patterns from large data sets. However, purely data-driven models might struggle when very difficult functions need to be learned or when there is not enough available training data. Fortunately, in many domains prior information can be retrieved and used to boost the performance of DL models. This paper presents a first survey of the approaches devised to integrate domain knowledge, expressed in the form of constraints, in DL learning models to improve their performance, in particular targeting deep neural networks. We identify five (non-mutually exclusive) categories that encompass the main approaches to inject domain knowledge: 1) acting on the features space, 2) modifications to the hypothesis space, 3) data augmentation, 4) regularization schemes, 5) constrained learning.
MoreTranslated text
Key words
deep learning models,domain knowledge,deep learning,constraint-based
AI Read Science
Must-Reading Tree
Example
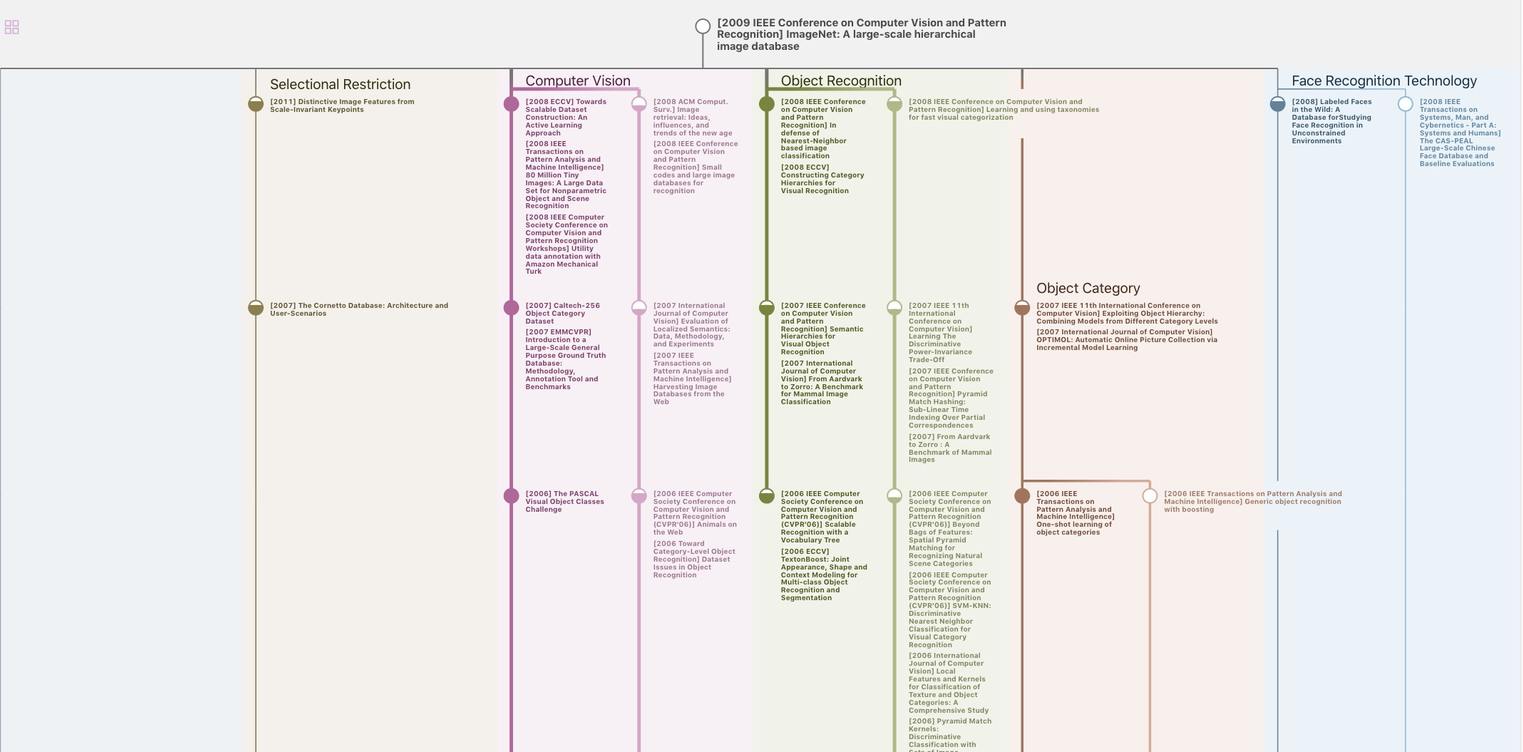
Generate MRT to find the research sequence of this paper
Chat Paper
Summary is being generated by the instructions you defined