A predictive model for prolonged hospital length of stay in surgical burn patients.
Burns : journal of the International Society for Burn Injuries(2020)
Abstract
BACKGROUND:Improvement in the care of burn patients has led to decreased mortality. Length of stay (LOS) has been used as a marker for quality of care in this population. However, the historical association of LOS as correlating only with % burn surface area (BSA) injury has been questioned with retrospective data suggesting other factors may also be associated with LOS. A model to predict prolonged LOS does not exist but could provide important information for clinicians and patients.
METHODS:Data from January 2014 to December 2016 was used to develop a predictive model utilizing multivariable logistic regression. Prolonged hospital LOS was the outcome used with multiple covariates utilized to identify various associations. Odds ratios (OR) and their associated 95% confidence interval (CI) were reported for each covariate in the final regression model. Model performance in both the training and validation sets was evaluated using area under the receiver operating characteristic (ROC) curve (AUC) for discrimination and the Hosmer-Lemeshow (HL) test for goodness-of-fit.
RESULTS:A total of 441 patients was included in the final analysis, 296 (67.1%) of which were in the training set. Within the training set, the median hospital LOS was 14 days with a range of 4 to 205 days. Patient age (in decades), hypertension, total BSA, involvement of perineum, and abnormal white blood cell count were independent risk factors for prolonged hospital length of stay. When using this separate dataset, the model had an AUC of 0.81 (95% CI 0.74-0.88) and had good calibration based on the HL-test (p=0.10).
CONCLUSIONS:Prolonged hospitalization following burns is predicted by patient age (in decades), TBSA, hypertension, perineal involvement, and abnormal white blood cell count.
MoreTranslated text
AI Read Science
Must-Reading Tree
Example
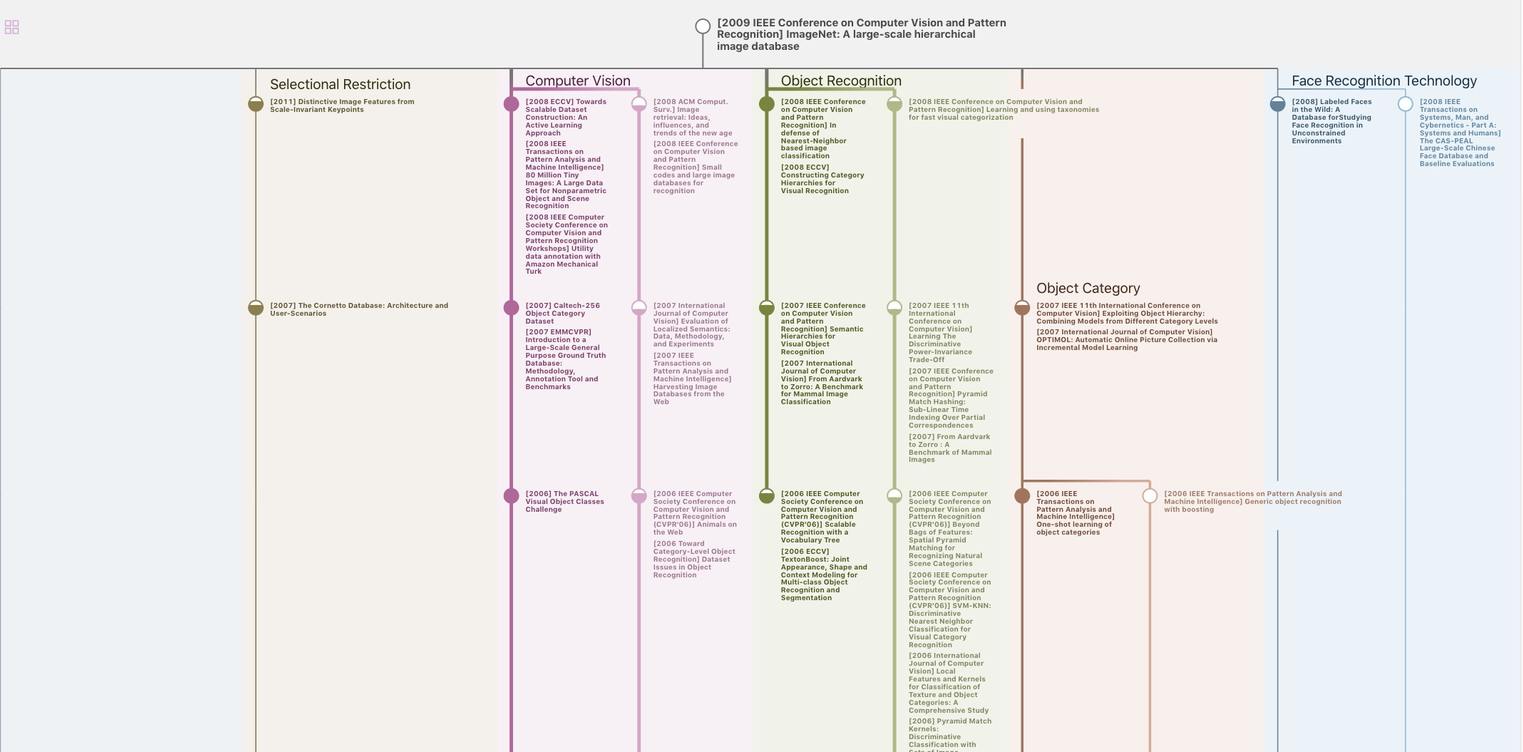
Generate MRT to find the research sequence of this paper
Chat Paper
Summary is being generated by the instructions you defined