Wafer-Level Die Re-Test Success Prediction Using Machine Learning
2020 IEEE Latin-American Test Symposium (LATS)(2020)
摘要
For high-volume production, time spent for wafer-level test is a crucial contributor to the overall product cost. At the same time, accurate filtering out of the defective dies at this step is essential to avoid escapes to the later more expensive stages of manufacturing test, e.g. package test, integrated system test, or even test escapes to the customer. The nature of the wafer test setup, in particular the mechanical probing, may imply re-test of the dies failed the test in the first run. This paper proposes a method for applying Machine Learning to efficiently predict whether it is favorable to invest extra time into re-test of a particular failed die. The training relies on supervised learning on a predefined subset of wafers, which include information about the first and re-test runs. The experimental results on actual commercial product data demonstrate efficiency of the method for re-test success prediction and, thus, significant optimization of the manufacturing test time. The demonstrated accuracy of prediction is 78%, subject to selection of suited data features and an efficient neural network model.
更多查看译文
关键词
Machine Learning,Die Inking,Wafer Testing
AI 理解论文
溯源树
样例
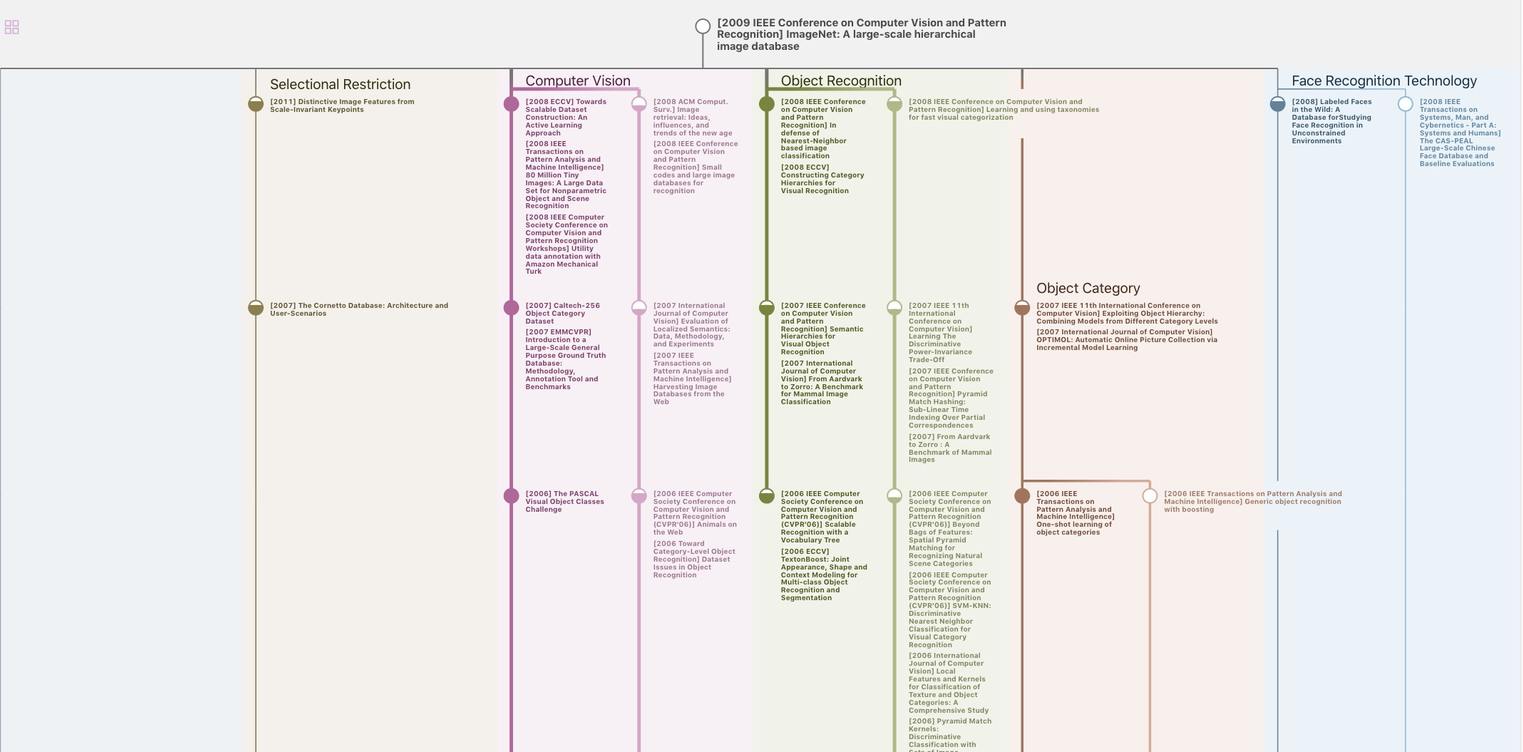
生成溯源树,研究论文发展脉络
Chat Paper
正在生成论文摘要