Multi Receptive Field Network for Semantic Segmentation
2020 IEEE WINTER CONFERENCE ON APPLICATIONS OF COMPUTER VISION (WACV)(2020)
摘要
Semantic segmentation is one of the key tasks in computer vision, which is to assign a category label to each pixel in an image. Despite significant progress achieved recently, most existing methods still suffer from two challenging issues: 1) the size of objects and stuff in an image can be very diverse, demanding for incorporating multi-scale features into the fully convolutional networks (FCNs); 2) the pixels close to or at the boundaries of object/stuff are hard to classify due to the intrinsic weakness of convolutional networks. To address the first issue, we propose a new Multi-Receptive Field Module (MRFM), explicitly taking multi-scale features into account. For the second issue, we design an edge-aware loss which is effective in distinguishing the boundaries of object/stuff. With these two designs, our Multi Receptive Field Network achieves new state-of-the-art results on two widely-used semantic segmentation benchmark datasets. Specifically, we achieve a mean IoU of 83.0 on the Cityscapes dataset and 88.4 mean IoU on the Pascal VOC2012 dataset.
更多查看译文
关键词
computer vision,category label,incorporating multiscale features,fully convolutional networks,semantic segmentation benchmark datasets,multireceptive field network,multireceptive field module
AI 理解论文
溯源树
样例
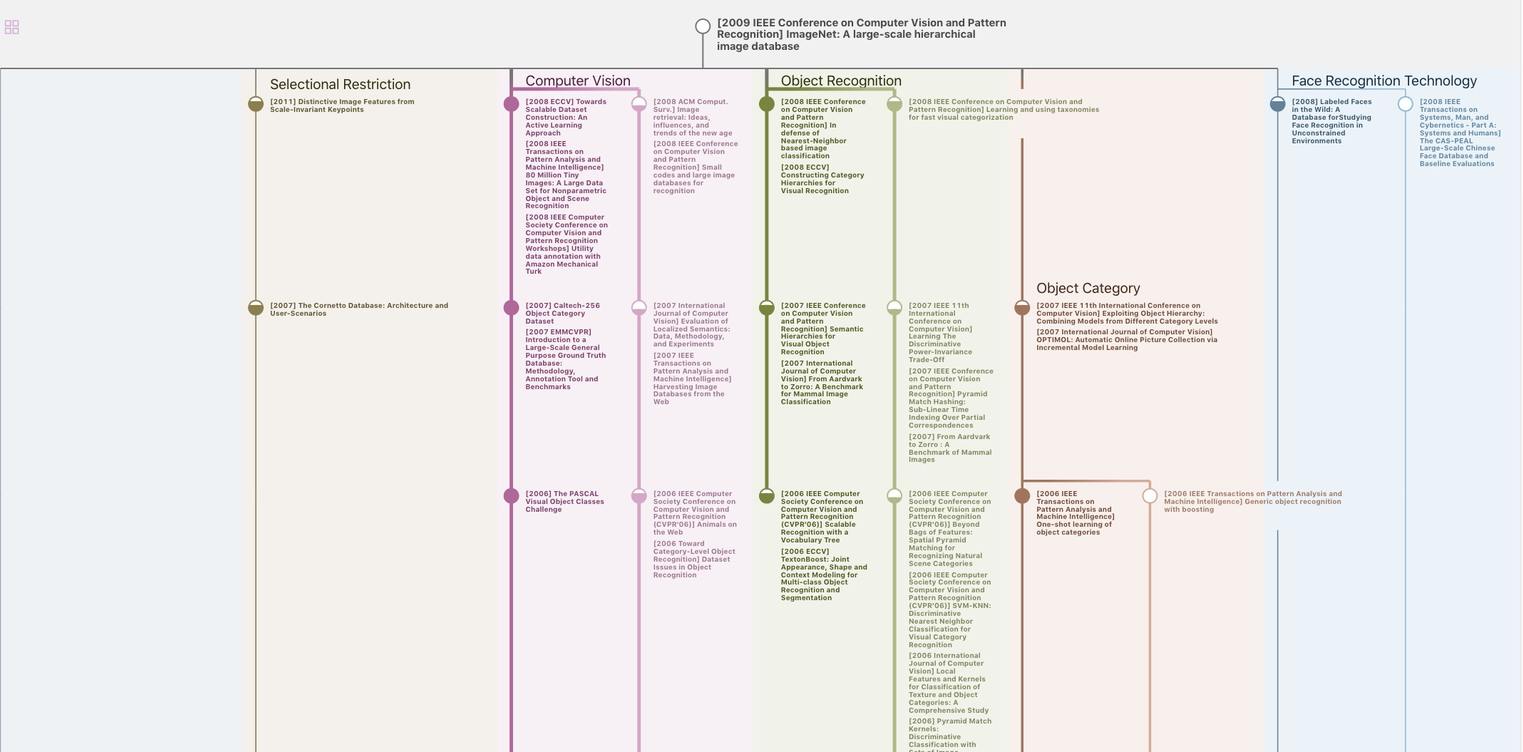
生成溯源树,研究论文发展脉络
Chat Paper
正在生成论文摘要