Fast, Simpler and More Accurate Hybrid ASR Systems Using Wordpieces
Interspeech 2020(2020)
摘要
In this work, we first show that on the widely used LibriSpeech benchmark, our transformer-based context-dependent connectionist temporal classification (CTC) system produces state-of-the-art results. We then show that using wordpieces as modeling units combined with CTC training, we can greatly simplify the engineering pipeline compared to conventional frame-based cross-entropy training by excluding all the GMM bootstrapping, decision tree building and force alignment steps, while still achieving very competitive word-error-rate. Additionally, using wordpieces as modeling units can significantly improve runtime efficiency since we can use larger stride without losing accuracy. We further confirm these findings on two internal \emph{VideoASR} datasets: German, which is similar to English as a fusional language, and Turkish, which is an agglutinative language.
更多查看译文
AI 理解论文
溯源树
样例
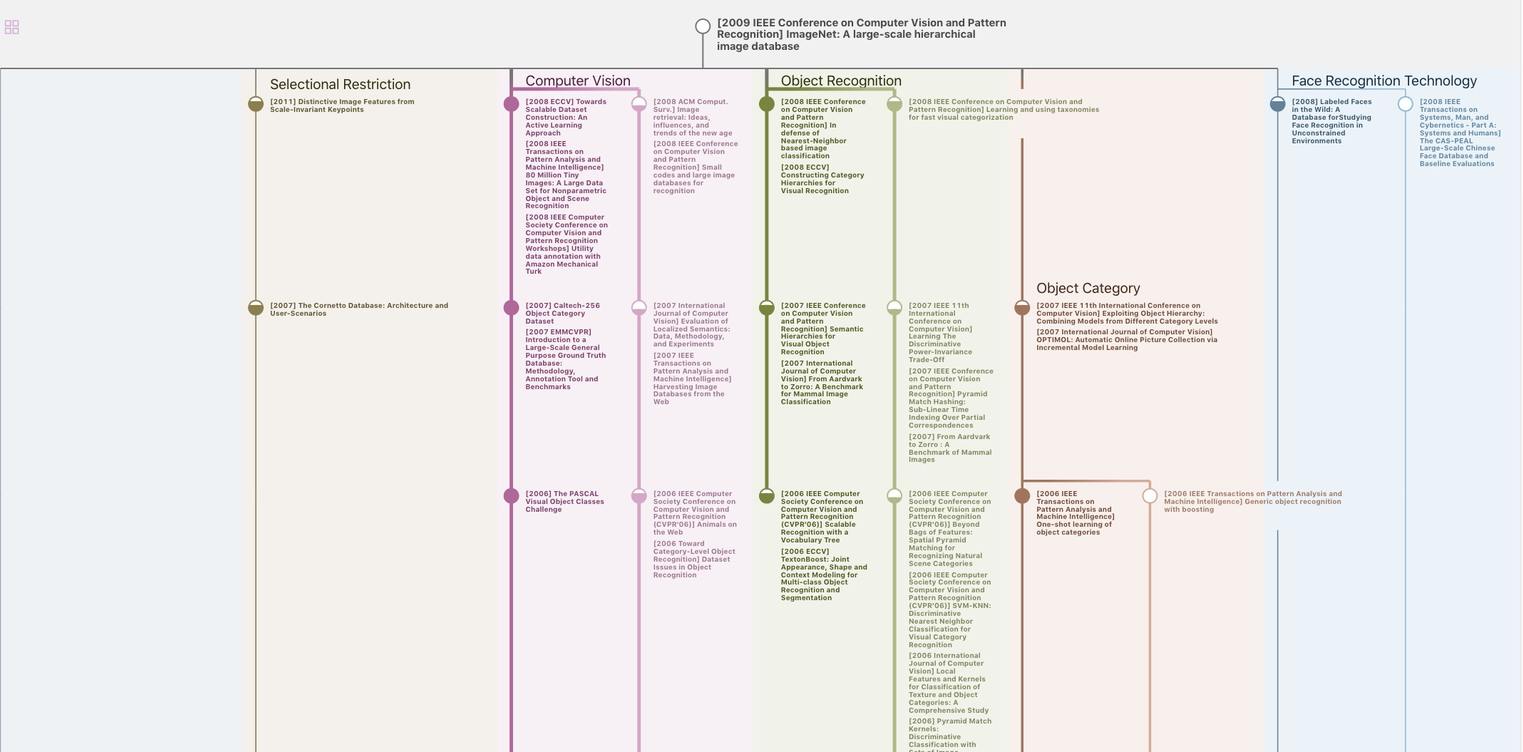
生成溯源树,研究论文发展脉络
Chat Paper
正在生成论文摘要