Disentangling Aspect and Opinion Words in Sentiment Analysis Using Lifelong PU Learning.
NFMCP@PKDD/ECML(2019)
摘要
While sentiment analysis can mine valuable information from online reviews, performing a fine-grained sentiment analysis task is very challenging due to the complex patterns in text. In this work, we focus on a Fine-grained Target-based Sentiment Analysis (FTSA) task, which is to identify target-specific aspect words and opinion words. This task is very useful in practice. However, existing solutions cannot generate satisfactory results, especially when we have limited or no labeled data. To provide a holistic solution, we design a novel two-stage approach. Stage one groups the target-related words (call t-words) for a given target, which is relatively easy. Stage two separates the aspect and opinion words from the grouped t-words, which is more challenging due to the lack of sufficient word-level aspect and opinion labels. To address it, we formulate the task in a Positive-Unlabeled (PU) learning setting and incorporate the idea of lifelong learning, which achieves promising results.
更多查看译文
关键词
sentiment analysis,opinion words,aspect,learning
AI 理解论文
溯源树
样例
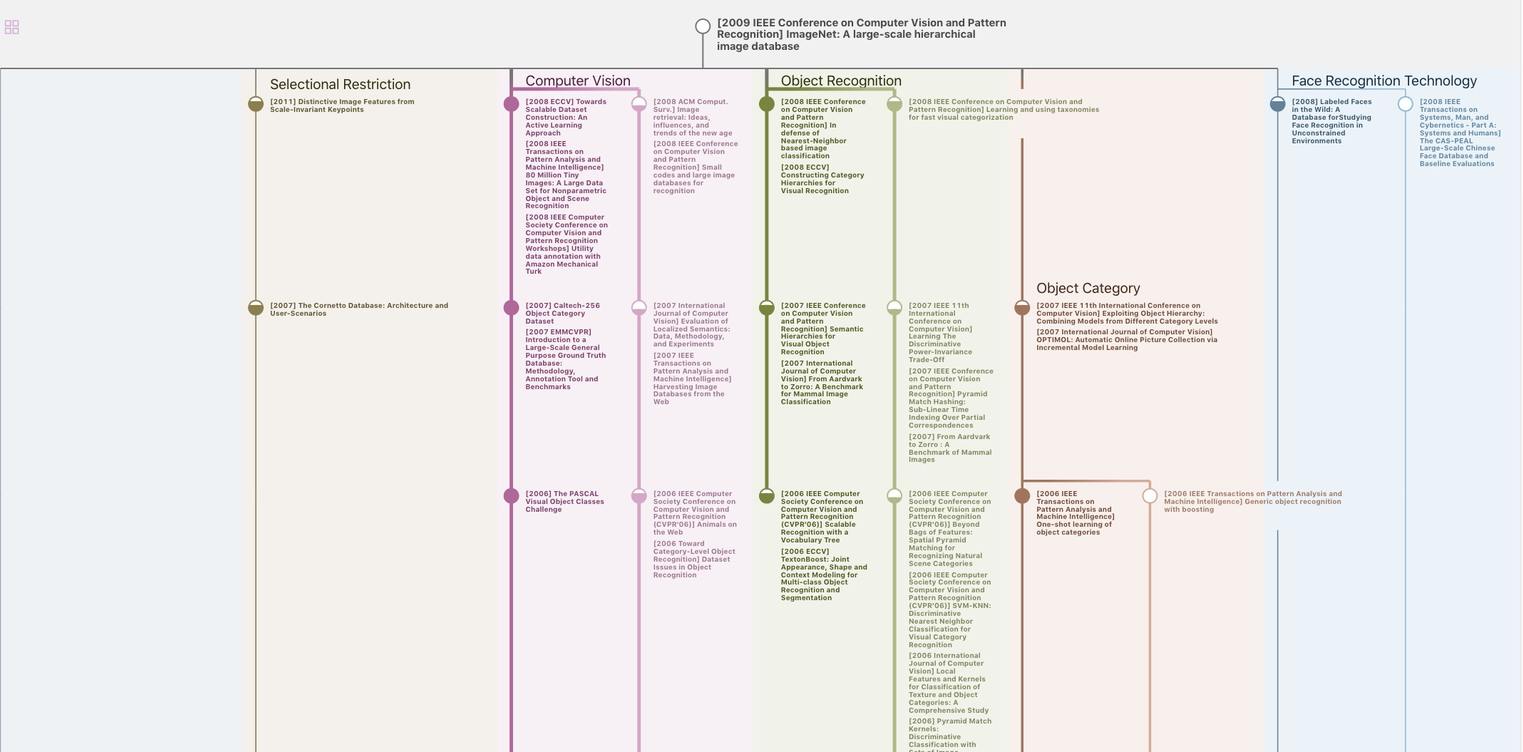
生成溯源树,研究论文发展脉络
Chat Paper
正在生成论文摘要