North Atlantic Right Whales Up-call Detection Using Multimodel Deep Learning
arXiv (Cornell University)(2020)
Abstract
A new method for North Atlantic Right Whales (NARW) up-call detection using Multimodel Deep Learning (MMDL) is presented in this paper. In this approach, signals from passive acoustic sensors are first converted to spectrogram and scalogram images, which are time-frequency representations of the signals. These images are in turn used to train an MMDL detec-tor, consisting of Convolutional Neural Networks (CNNs) and Stacked Auto Encoders (SAEs). Our experimental studies revealed that CNNs work better with spectrograms and SAEs with sca-lograms. Therefore in our experimental design, the CNNs are trained by using spectrogram im-ages, and the SAEs are trained by using scalogram images. A fusion mechanism is used to fuse the results from individual neural networks. In this paper, the results obtained from the MMDL detector are compared with those obtained from conventional machine learning algorithms trained with handcraft features. It is shown that the performance of the MMDL detector is sig-nificantly better than those of the representative conventional machine learning methods in terms of up-call detection rate, non-up-call detection rate, and false alarm rate.
MoreTranslated text
Key words
north atlantic right whales,deep learning,up-call
AI Read Science
Must-Reading Tree
Example
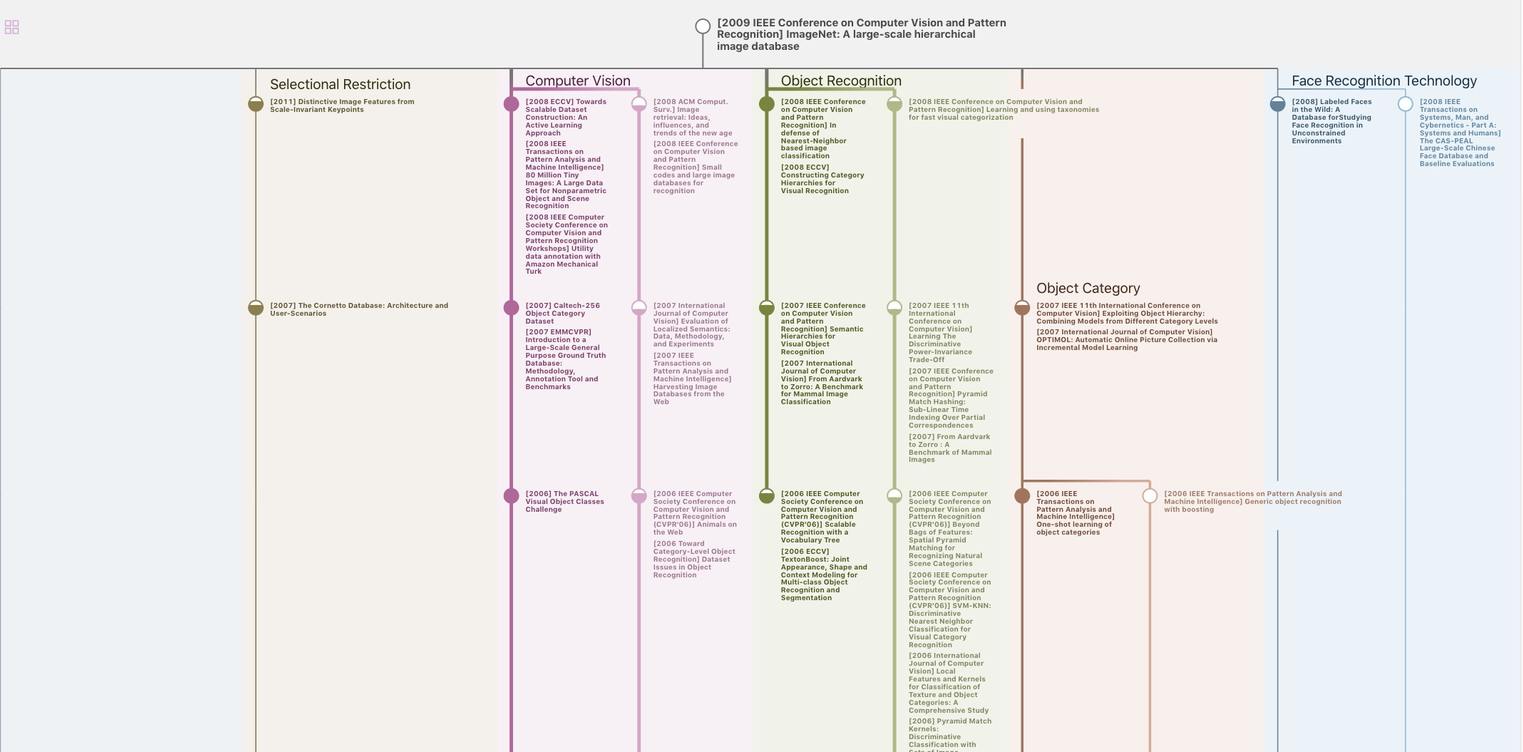
Generate MRT to find the research sequence of this paper
Chat Paper
Summary is being generated by the instructions you defined