An invertible crystallographic representation for general inverse design of inorganic crystals with targeted properties
MATTER(2022)
摘要
Realizing general inverse design could greatly accelerate the discovery of new materials with user-defined properties. However, stateof-the-art generative models tend to be limited to a specific composition or crystal structure. Herein, we present a framework capable of general inverse design (not limited to a given set of elements or crystal structures), featuring a generalized invertible representation that encodes crystals in both real and reciprocal space, and a property-structured latent space from a variational autoencoder (VAE). In three design cases, the framework generates 142 new crystals with user-defined formation energies, bandgap, thermoelectric (TE) power factor, and combinations thereof. These generated crystals, absent in the training database, are validated by first-principles calculations. The success rates (number of first-principles-validated target-satisfying crystals/number of designed crystals) ranges between 7.1% and 38.9%. These results represent a significant step toward property-driven general inverse design using generative models, although practical challenges remain when coupled with experimental synthesis.
更多查看译文
关键词
general inverse design,solid-state materials,invertible crystallographic representation,generalized crystallographic representation,property-structured latent space,variational autoencoder,machine learning,thermoelectrics,generative model
AI 理解论文
溯源树
样例
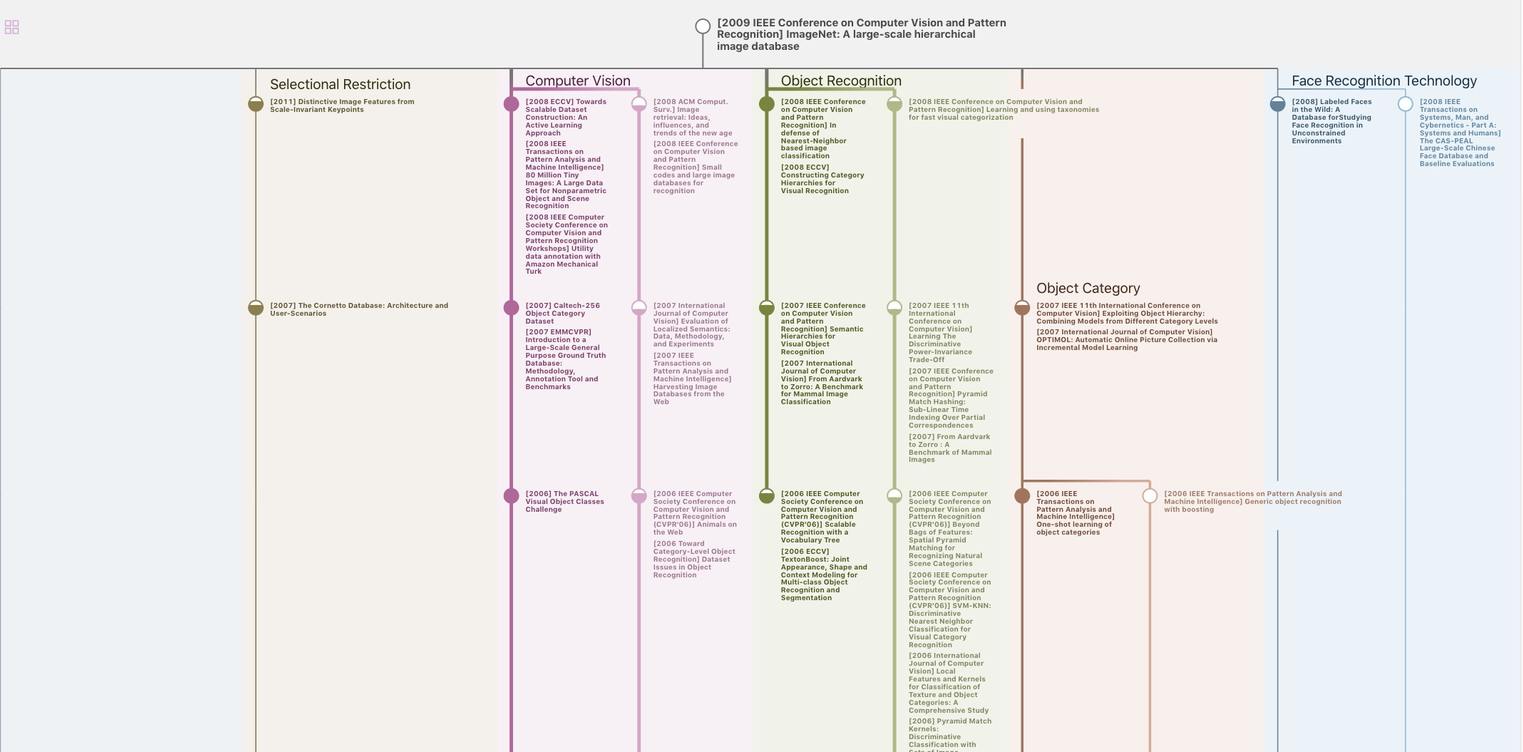
生成溯源树,研究论文发展脉络
Chat Paper
正在生成论文摘要