Using Cognitive Models to Train Big Data Models with Small Data
AAMAS '19: International Conference on Autonomous Agents and Multiagent Systems Auckland New Zealand May, 2020(2020)
摘要
Modeling and predicting human behavior pose a difficult challenge for AI and other related fields. Some current techniques (e.g., cognitive architectures) are able to model people's goals and actions from little data, but have poor predictive capabilities. Other methods (e.g., deep networks) have strong predictive capabilities but require large amounts of data to train the model; such abundant empirical data on human performance is not available for many human-based tasks. We show a novel and general method of generating copious synthetic data of human behavior using a cognitive architecture, and then use the data to train a deep network classifier to predict ensuing human actions. We test our approach by predicting human actions on a supervisory control task; the results show that our approach provides superior prediction when compared to training a classifier with only (limited) empirical data.
更多查看译文
AI 理解论文
溯源树
样例
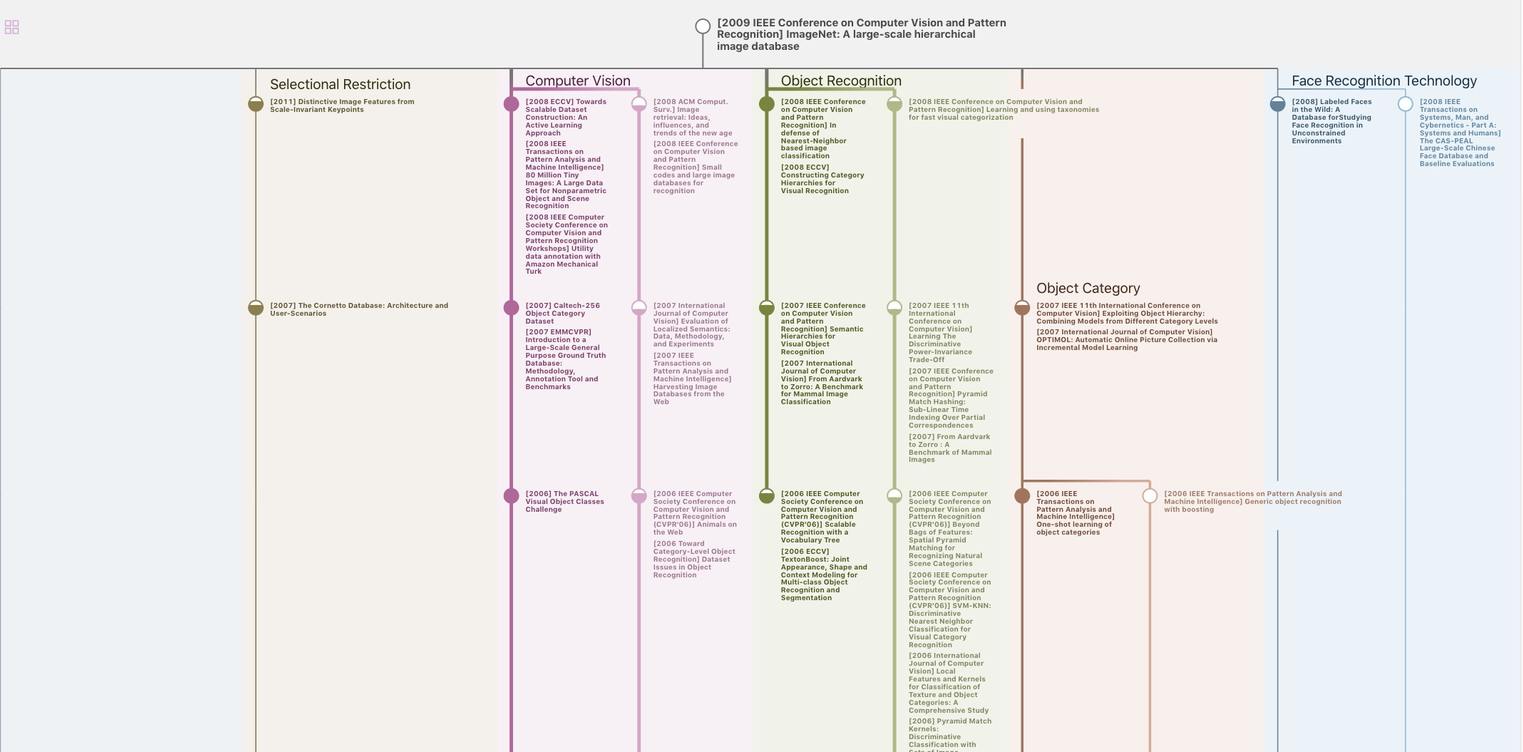
生成溯源树,研究论文发展脉络
Chat Paper
正在生成论文摘要