Functions And Eigenvectors Of Partially Known Matrices With Applications To Network Analysis
APPLIED NUMERICAL MATHEMATICS(2021)
摘要
Matrix functions play an important role in applied mathematics. In network analysis, in particular, the exponential of the adjacency matrix associated with a network provides valuable information about connectivity, as well as about the relative importance or centrality of nodes. Another popular approach to rank the nodes of a network is to compute the left Perron vector of the adjacency matrix for the network. The present article addresses the problem of evaluating matrix functions, as well as computing an approximation to the left Perron vector, when only some of the columns and/or some of the rows of the adjacency matrix are known. Applications to network analysis are considered, when only some sampled columns and/or rows of the adjacency matrix that defines the network are available. A sampling scheme that takes the connectivity of the network into account is described. Computed examples illustrate the performance of the methods discussed. (C) 2020 IMACS. Published by Elsevier B.V. All rights reserved.
更多查看译文
关键词
Matrix function, Arnoldi process, Low-rank approximation, Cross approximation, Column subset selection, Centrality measure
AI 理解论文
溯源树
样例
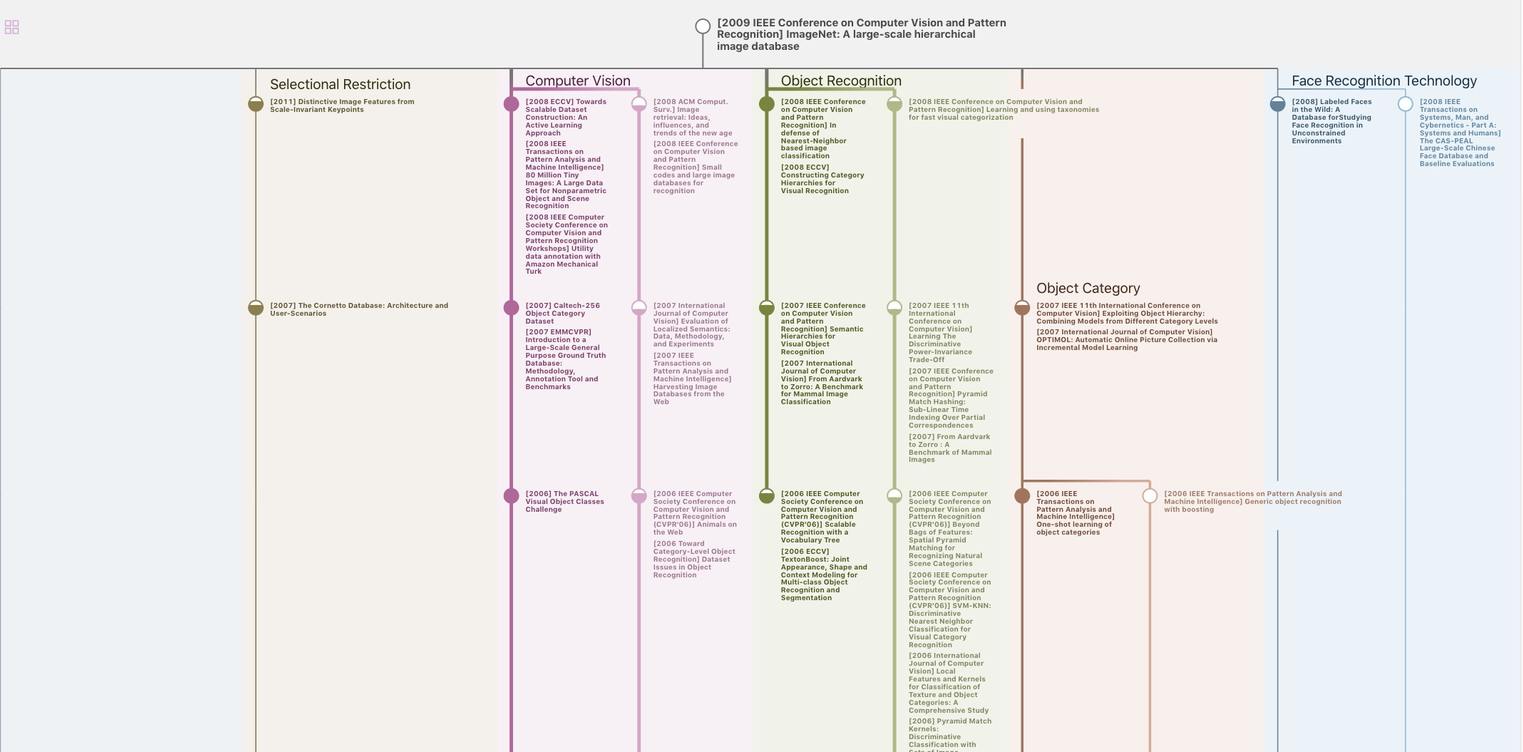
生成溯源树,研究论文发展脉络
Chat Paper
正在生成论文摘要