Robust Lasso-Zero for sparse corruption and model selection with missing covariates
arxiv(2020)
摘要
We propose Robust Lasso-Zero, an extension of the Lasso-Zero methodology [Descloux and Sardy, 2018], initially introduced for sparse linear models, to the sparse corruptions problem. We give theoretical guarantees on the sign recovery of the parameters for a slightly simplified version of the estimator, called Thresholded Justice Pursuit. The use of Robust Lasso-Zero is showcased for variable selection with missing values in the covariates. In addition to not requiring the specification of a model for the covariates, nor estimating their covariance matrix or the noise variance, the method has the great advantage of handling missing not-at random values without specifying a parametric model. Numerical experiments and a medical application underline the relevance of Robust Lasso-Zero in such a context with few available competitors. The method is easy to use and implemented in the R library lass0.
更多查看译文
关键词
incomplete data,informative missing values,Lasso-Zero,sparse corruptions,support recovery
AI 理解论文
溯源树
样例
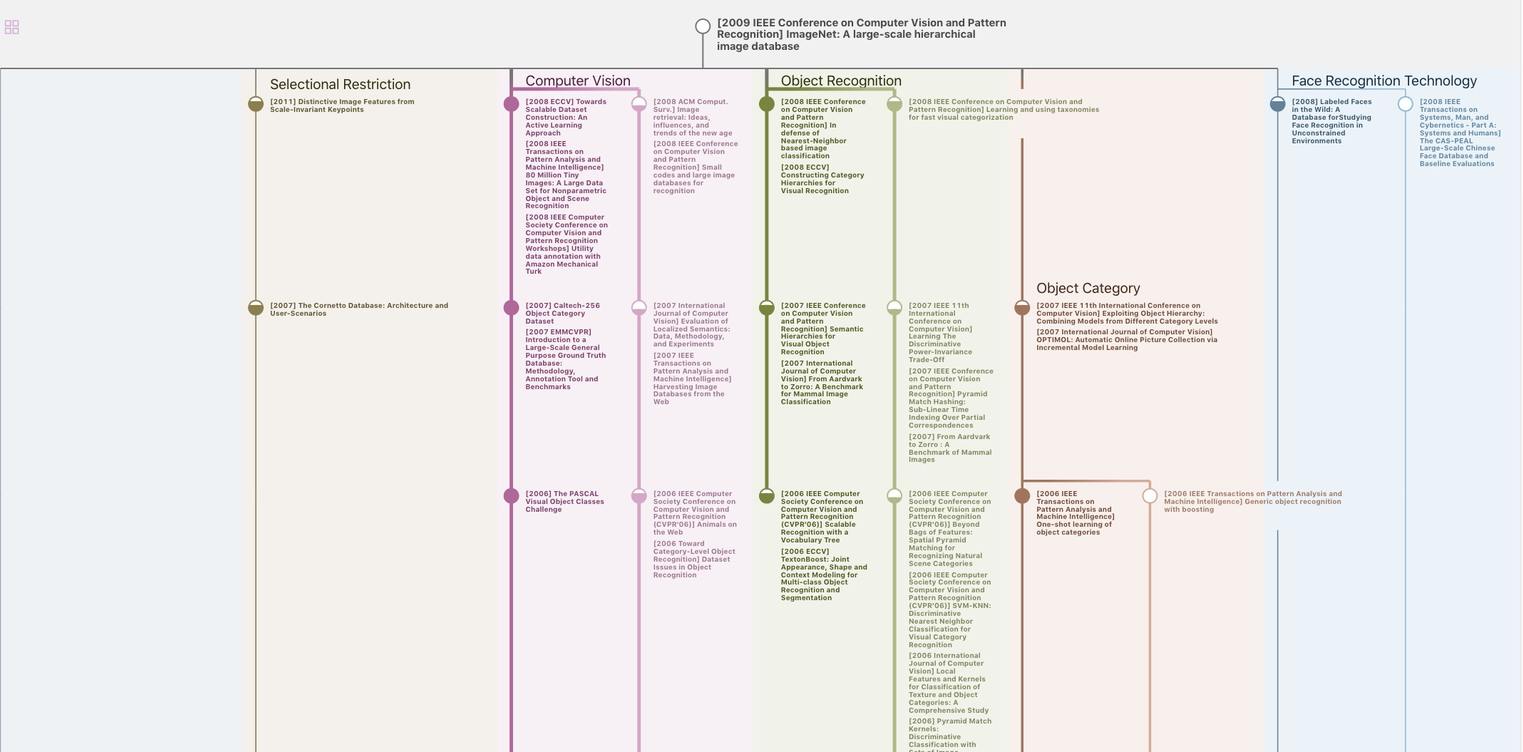
生成溯源树,研究论文发展脉络
Chat Paper
正在生成论文摘要