Distributed Stochastic Constrained Composite Optimization over Time-Varying Network with a Class of Communication Noise
IEEE TRANSACTIONS ON CYBERNETICS(2022)
摘要
This paper is concerned with distributed stochastic multi-agent constrained optimization problem over time-varying network with a class of communication noise. This paper considers the problem in composite optimization setting which is more general in the literature of noisy network optimization. It is noteworthy that the mainstream existing methods for noisy network optimization are Euclidean projection based. Based on Bregman projection-based mirror descent scheme, we present a non-Euclidean method and investigate their convergence behavior. This method is the distributed stochastic composite mirror descent type method (DSCMD-N) which provides a more general algorithm framework. Some new error bounds for DSCMD-N are obtained. To the best of our knowledge, this is the first work to analyze and derive convergence rates of optimization algorithm in noisy network optimization. We also show that an optimal rate of $O(1/\sqrt{T})$ in nonsmooth convex optimization can be obtained for the proposed method under appropriate communication noise condition. Moreover, novel convergence results are comprehensively derived in expectation convergence, high probability convergence, and almost surely sense.
更多查看译文
关键词
Optimization,Convergence,Noise measurement,Mirrors,Stochastic processes,Optimization methods,Linear programming,Communication noise,composite optimization,distributed optimization,mirror descent,multiagent network
AI 理解论文
溯源树
样例
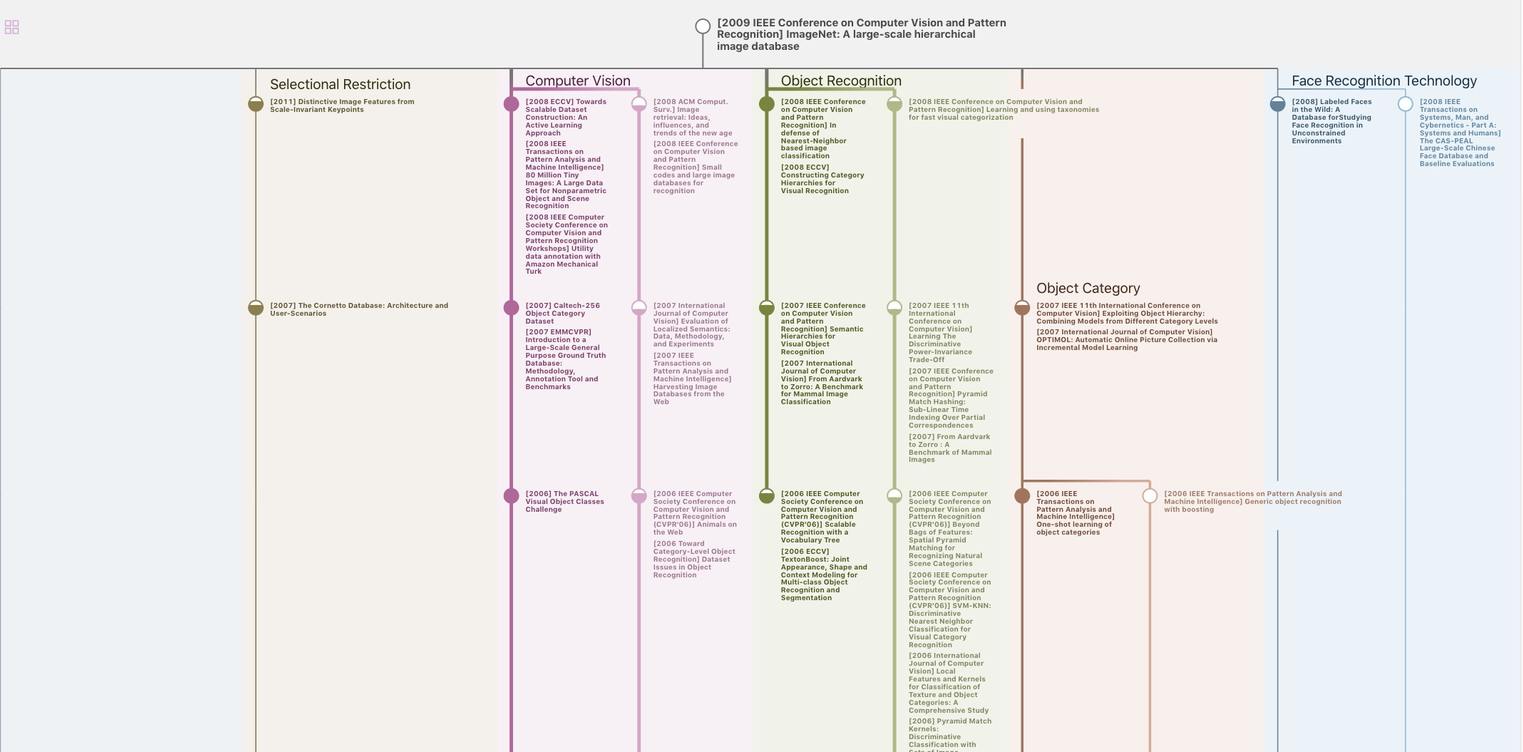
生成溯源树,研究论文发展脉络
Chat Paper
正在生成论文摘要