XNORAM: An Efficient Computing-in-Memory Architecture for Binary Convolutional Neural Networks with Flexible Dataflow Mapping
2020 2nd IEEE International Conference on Artificial Intelligence Circuits and Systems (AICAS)(2020)
摘要
In this paper, an energy-efficient computing-inmemory architecture for binary convolutional neural networks, called XNORAM, is proposed. The XNORAM employs 6T feature cells and 10T weight cells to form one XNORAM column. Multiplexed XNOR operations are embedded in each column. To address the data reuse in convolutional neural networks, flexible dataflow mapping is supported on XNORAM to minimize the external data access. To verify the architecture, we design a 4-KB XNORAM prototype in 65nm CMOS technology. It achieves a throughput of 18. 5GOPs at 100-MHz clock rate and 1.0-V power supply. XNOR-AlexNet is performed on the design achieving 39.86 TOPS/W and 4.63 GOPS/KB utilization with only 1.3% accuracy loss comparing to the original XNOR-Net result on GPUs.
更多查看译文
关键词
XNORAM column,multiplexed XNOR operations,external data access,binary convolutional neural networks,energy-efficient computing-in-memory architecture,feature cells,dataflow mapping,power supply
AI 理解论文
溯源树
样例
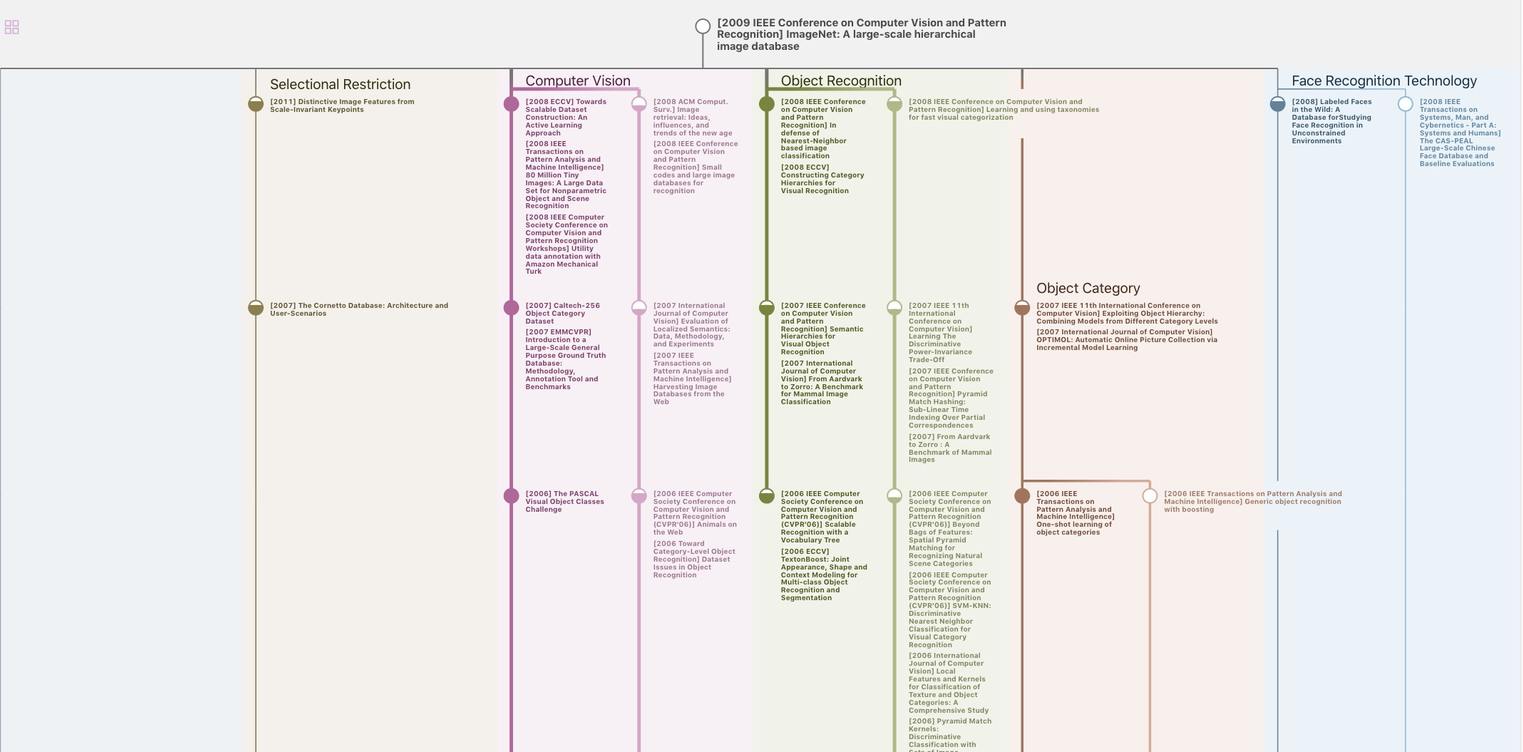
生成溯源树,研究论文发展脉络
Chat Paper
正在生成论文摘要