Visualization Techniques for Outlier Data
2020 International Conference on Artificial Intelligence in Information and Communication (ICAIIC)(2020)
摘要
When deep learning models classify the actual data and outlier data, most of them do not have enough information about the outlier, which might cause misclassification. Therefore, there is a need for an efficient way to analyze outlier data through visualization. We propose a visualization method combining LBP, LLE and SMOTE for outlier data detection. Furthermore, we introduce a new confusion visualization method that uses the similarity of pixel density distributions. We also present a new histogram visualization method using the frequency of pixel position's distribution in EDA. To validate its effectiveness, we compared the proposed method with UMAP and LLE. In evaluation, the outlier data is generated by three types of GAN (Vanilla GAN, DRAGAN, and EBGAN). The results of the proposed visualization method show its usefulness for outlier detection.
更多查看译文
关键词
Combined LBP LLE with Smote visualization,Confusion visualization,Histogram visualization,Outlier data
AI 理解论文
溯源树
样例
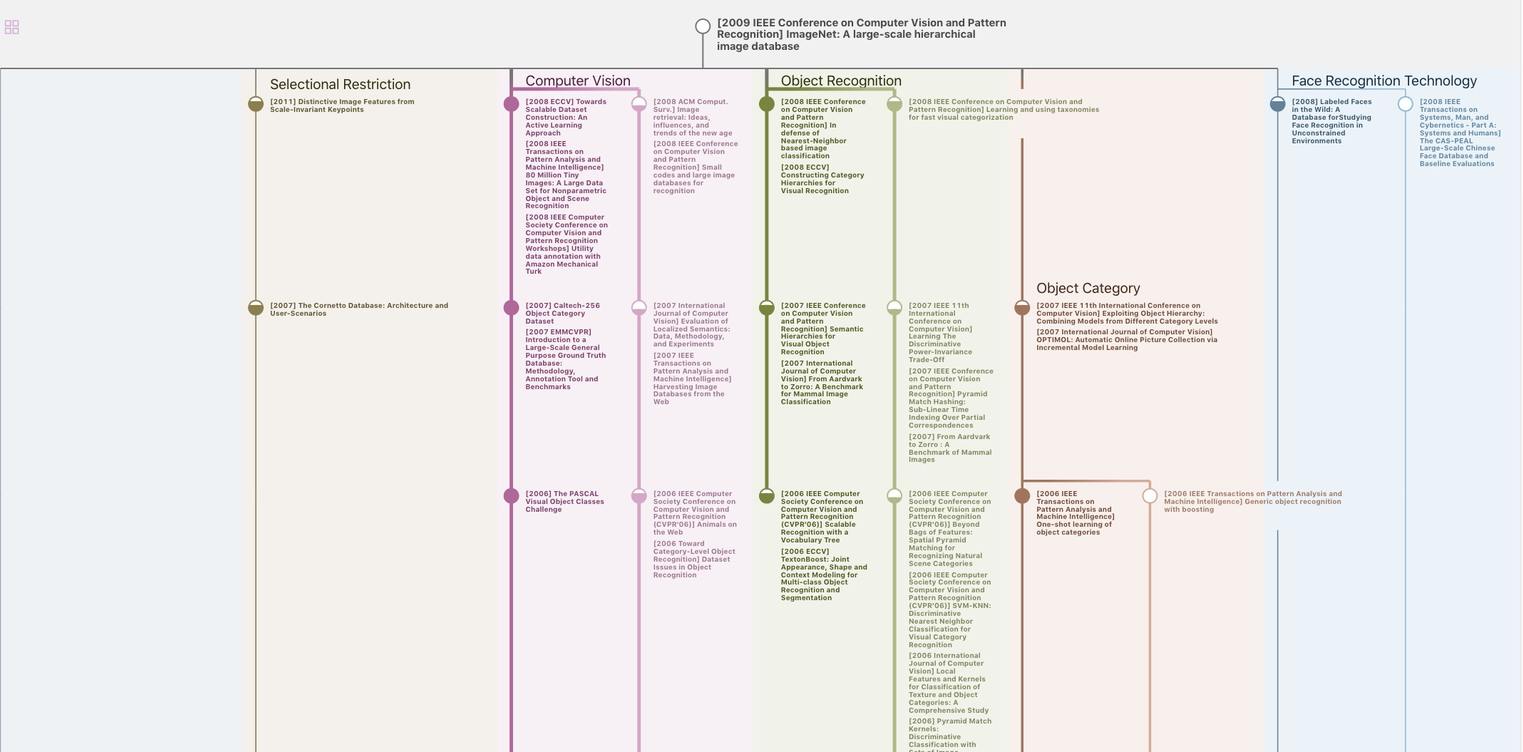
生成溯源树,研究论文发展脉络
Chat Paper
正在生成论文摘要