A privacy-preserving density peak clustering algorithm in cloud computing.
CONCURRENCY AND COMPUTATION-PRACTICE & EXPERIENCE(2020)
摘要
Aiming at preventing the privacy disclosure of sensitive information, issues related to privacy protection in cloud computing have attracted the interest of researchers. To protect the privacy of users during clustering in a cloud computing environment, we present a privacy-preserving density peak clustering (PPDPC) algorithm that neither discloses personal privacy information nor leaks the cluster centers. Our scheme contains two steps of density peak clustering: First, a cloud service provider calculates the cluster centers without knowing each participant's private data and without disclosing any cluster center information to the other participants, and second, participant allocation is secure and every participant is prevented from identifying the other members of the same cluster. Security analysis and comparison experiments show that the proposed PPDPC algorithm not only obtains good accuracy with respect to density peak clustering but also resists collusion attacks even if the cloud service provider is collaborating with all except one participant. Both theoretical analysis and experimental results confirm the security and accuracy of our method.
更多查看译文
关键词
cloud computing,data mining,density peak clustering,homomorphic encryption,privacy preservation
AI 理解论文
溯源树
样例
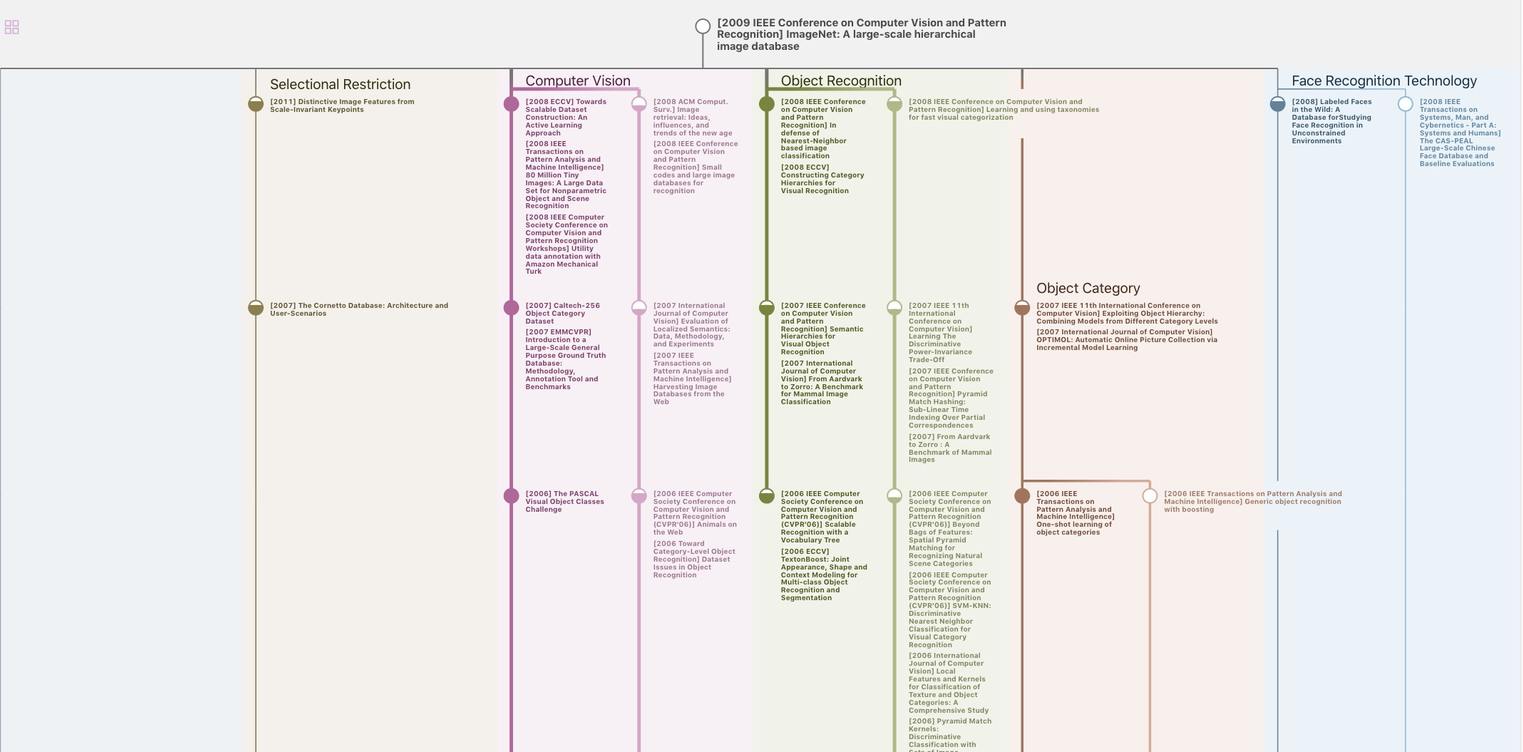
生成溯源树,研究论文发展脉络
Chat Paper
正在生成论文摘要