Neural Q- Learning Based on Residual Gradient for Nonlinear Control Systems
2019 International Conference on Control, Automation and Information Sciences (ICCAIS)(2019)
摘要
To solve the control problem of nonlinear system under continuous state space, this paper puts forward a neural Q-learning algorithm based on residual gradient method. Firstly, the multi-layer feedforward neural network is utilized to approximate the Q-value function, overcoming the “dimensional disaster” in the classical reinforcement learning. Then based on the residual gradient method, a mini-batch gradient descent is implemented by the experience replay to update the neural network parameters, which can effectively reduce the iterations number and increase the learning speed. Moreover, the momentum optimization method is introduced to ensure the stability of the training process further and improve the convergence. In order to balance exploration and utilization better, ε-decreasing strategy replaces ε-greedy for action selection. The simulation results of CartPole control task show the correctness and effectiveness of the proposed algorithm.
更多查看译文
关键词
Q-Iearning,feedforward neural network,value function approximation,residual gradient method,nonlinear control systems
AI 理解论文
溯源树
样例
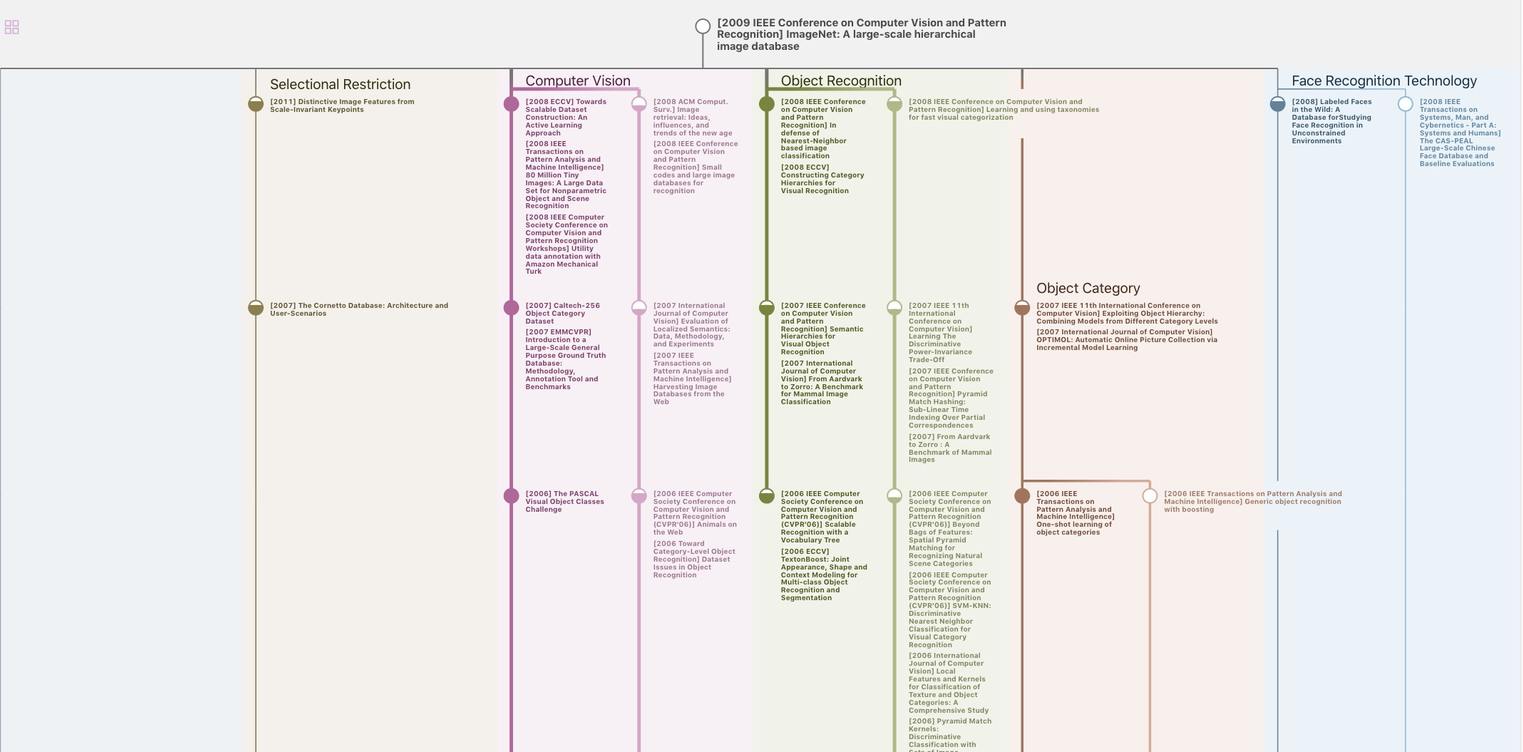
生成溯源树,研究论文发展脉络
Chat Paper
正在生成论文摘要