Predicting the Disease Genes of Multiple Sclerosis Based on Network Representation Learning.
FRONTIERS IN GENETICS(2020)
摘要
Multiple sclerosis (MS) is an autoimmune disease for which it is difficult to find exact disease-related genes. Effectively identifying disease-related genes would contribute to improving the treatment and diagnosis of multiple sclerosis. Current methods for identifying disease-related genes mainly focus on the hypothesis of guilt-by-association and pay little attention to the global topological information of the whole protein-protein-interaction (PPI) network. Besides, network representation learning (NRL) has attracted a huge amount of attention in the area of network analysis because of its promising performance in node representation and many downstream tasks. In this paper, we try to introduce NRL into the task of disease-related gene prediction and propose a novel framework for identifying the disease-related genes multiple sclerosis. The proposed framework contains three main steps: capturing the topological structure of the PPI network using NRL-based methods, encoding learned features into low-dimensional space using a stacked autoencoder, and training a support vector machine (SVM) classifier to predict disease-related genes. Compared with three state-of-the-art algorithms, our proposed framework shows superior performance on the task of predicting disease-related genes of multiple sclerosis.
更多查看译文
关键词
multiple sclerosis,network embedding,disease gene prediction,PPI network,deep learning
AI 理解论文
溯源树
样例
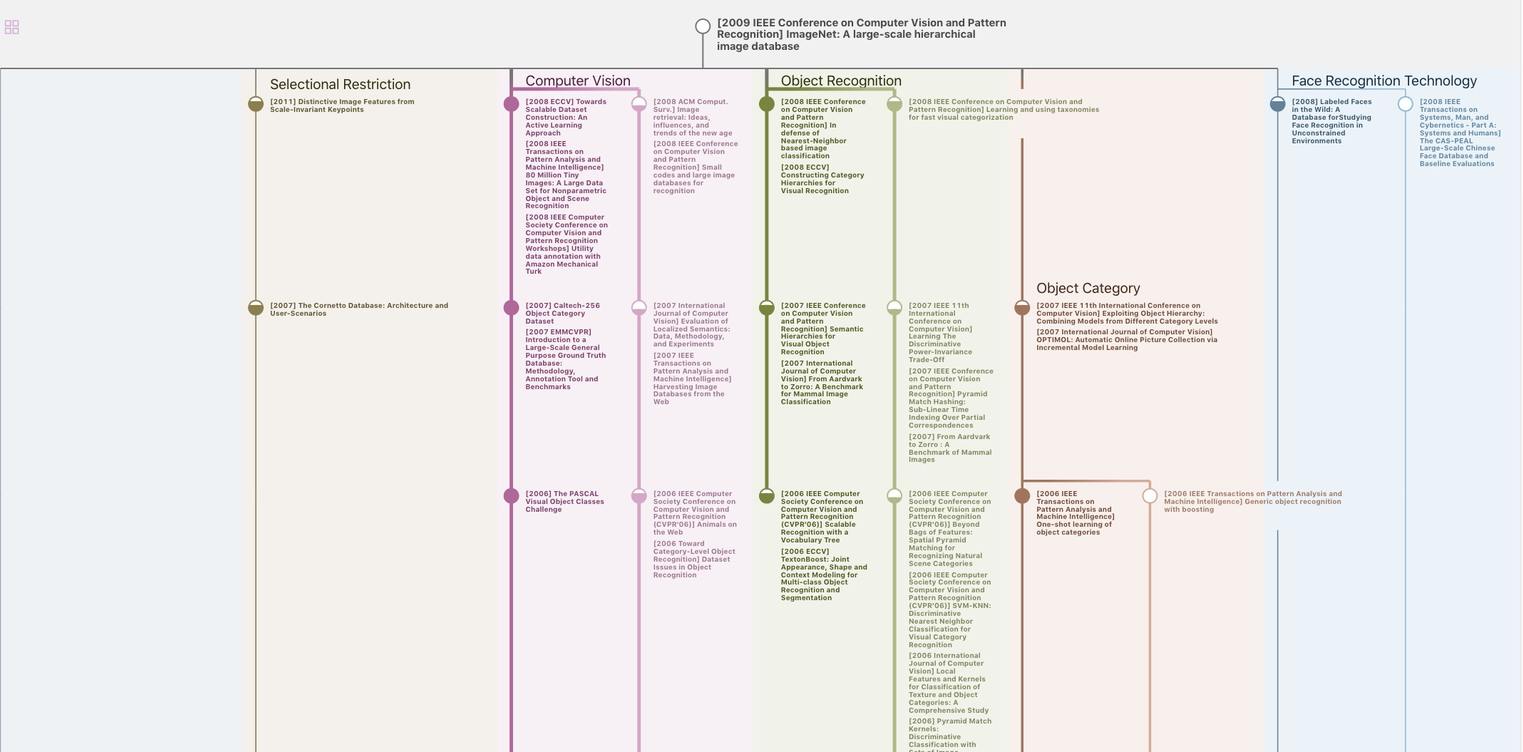
生成溯源树,研究论文发展脉络
Chat Paper
正在生成论文摘要