Evaluating and Boosting Reinforcement Learning for Intra-Domain Routing
2019 IEEE 16th International Conference on Mobile Ad Hoc and Sensor Systems (MASS)(2019)
摘要
The success of machine learning in domains such as computer vision and computer games has triggered a surge of interest in applying machine learning in computer networks. This paper tries to answer a broadly-debated question: can we improve the performance of intradomain routing, one of the most fundamental blocks in the Internet, with reinforcement learning (RL)? Due to the complex network traffic conditions and the large action space in routing, it is difficult to give a definite answer for existing RL-based routing solutions. To gain an in-depth understanding on the challenges of RL-based routing, we systematically classify different RL-based routing solutions and investigate the performance of several representative approaches, in terms of scalability, stability, robustness, and convergence. With the lessons learned in evaluating various RL-based routing solutions, we propose two methods, called supervised Q-network routing (SQR) and discrete link weight-based routing (DLWR), which boost the performance of RL-based routing and outperform the de facto shortest path intradomain routing.
更多查看译文
关键词
Intradomain routing,reinforcement learning,RL-based routing
AI 理解论文
溯源树
样例
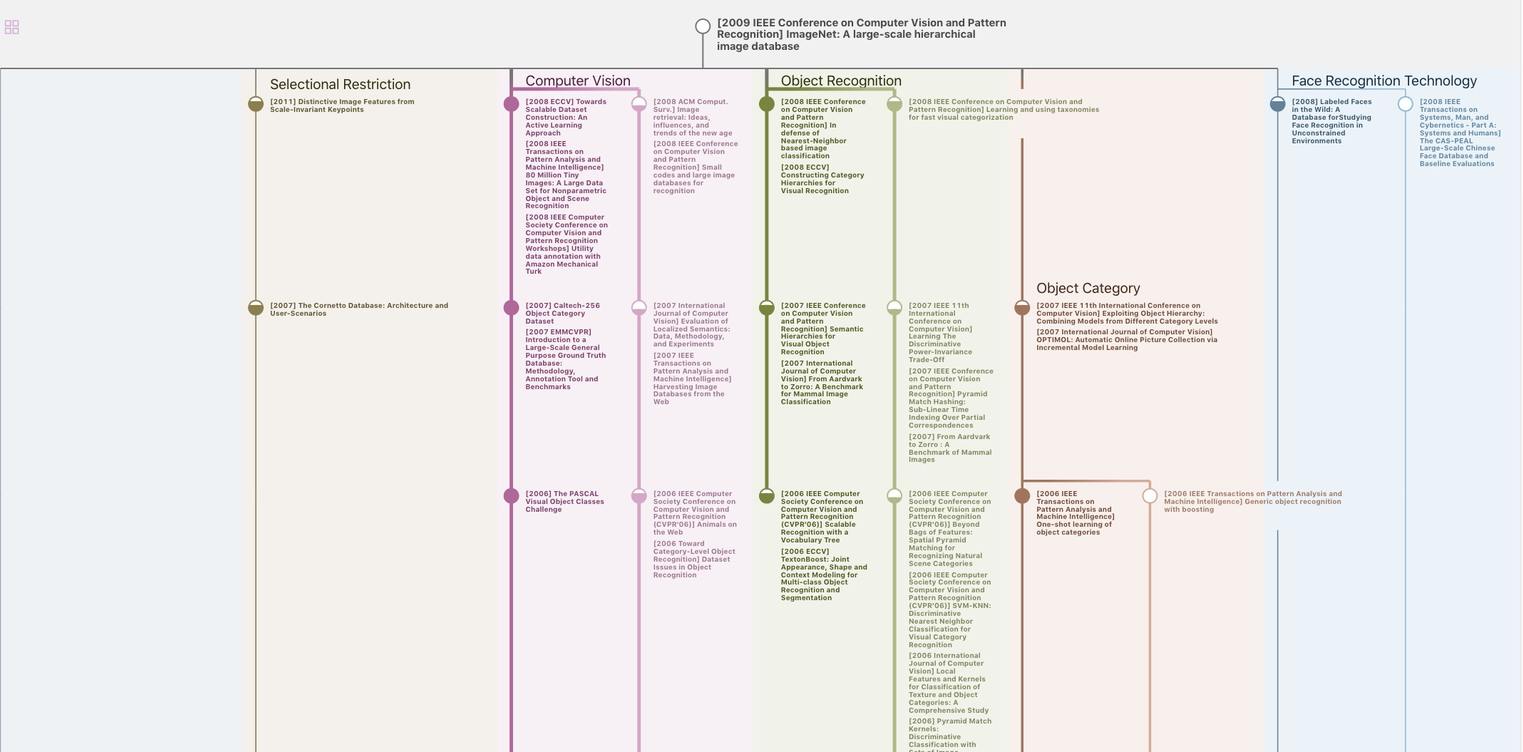
生成溯源树,研究论文发展脉络
Chat Paper
正在生成论文摘要