Multiclass Probit-Based Origin–Destination Estimation Using Multiple Data Types
JOURNAL OF TRANSPORTATION ENGINEERING PART A-SYSTEMS(2018)
Abstract
This paper proposes a bilevel optimization model for multiclass origin-destination (O-D) estimation using various types of data. The multiclass character of the model, a new feature and major contribution to the literature, is important because of increasing interest in simultaneous estimation of O-D tables for various classes of trucks and automobiles. The upper-level optimization is used to derive O-D table entries by minimizing the sum of squared differences between observations from different data sources and the predictions of those values. A probit model is assumed in the lower- level stochastic user equilibrium problem for flow prediction. Extensive experiments have been performed on a test network with different types of link count sensors and turning movements. The tests verify the problem formulation and solution algorithm and offer important insights into the multiclass O-D estimation process with the different types of available data. Adding turning movement data can improve O-D estimation by 71%. Furthermore, classification information is interchangeable among different types of sensors.
MoreTranslated text
Key words
origin–destination estimation,multiple data types,probit-based
AI Read Science
Must-Reading Tree
Example
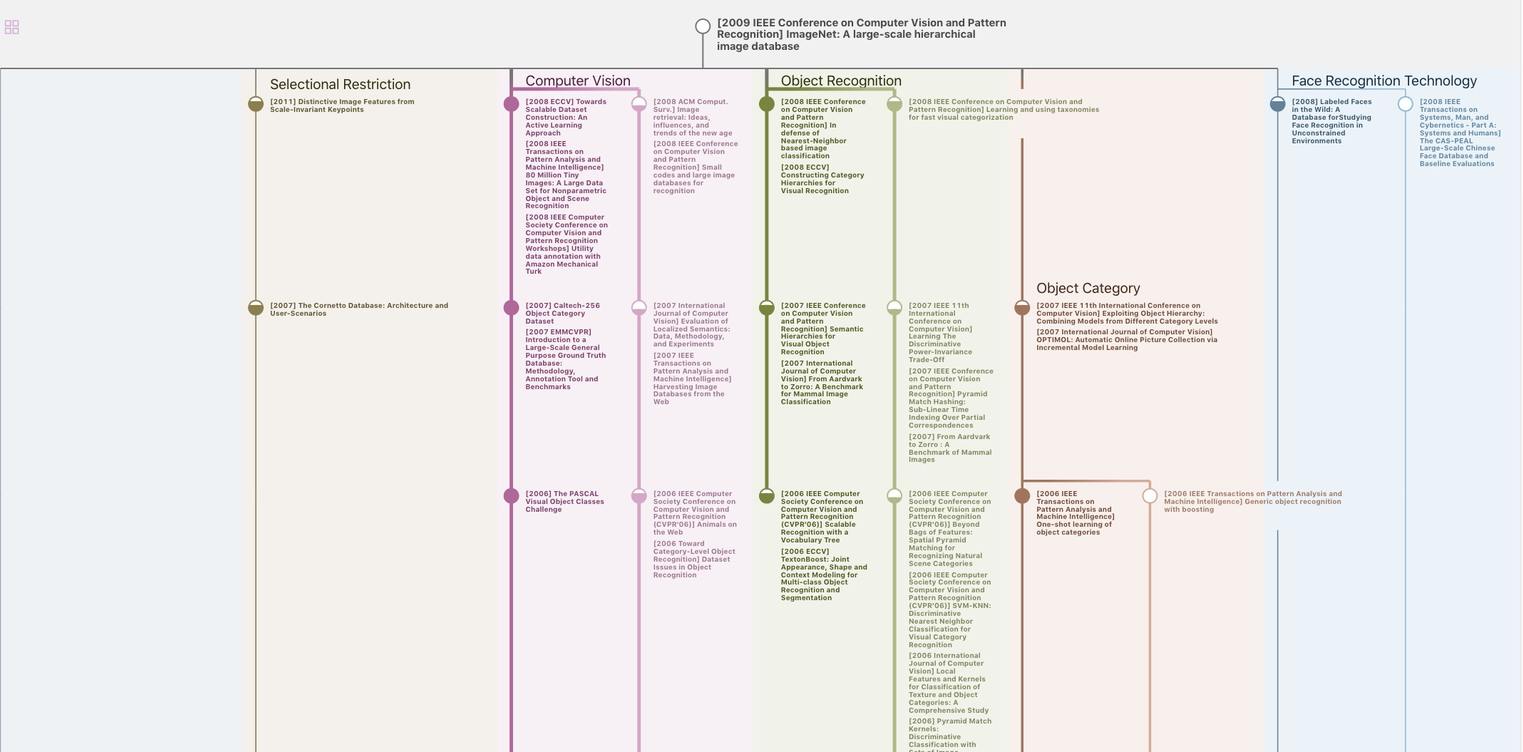
Generate MRT to find the research sequence of this paper
Chat Paper
Summary is being generated by the instructions you defined