Every Document Owns Its Structure: Inductive Text Classification via Graph Neural Networks
58TH ANNUAL MEETING OF THE ASSOCIATION FOR COMPUTATIONAL LINGUISTICS (ACL 2020)(2020)
摘要
Text classification is fundamental in natural language processing (NLP), and Graph Neural Networks (GNN) are recently applied in this task. However, the existing graph-based works can neither capture the contextual word relationships within each document nor fulfil the inductive learning of new words. In this work, to overcome such problems, we propose TextING for inductive text classification via GNN. We first build individual graphs for each document and then use GNN to learn the fine-grained word representations based on their local structures, which can also effectively produce embeddings for unseen words in the new document. Finally, the word nodes are aggregated as the document embedding. Extensive experiments on four benchmark datasets show that our method outperforms state-of-the-art text classification methods.
更多查看译文
关键词
inductive text classification,text classification,graph neural networks,neural networks
AI 理解论文
溯源树
样例
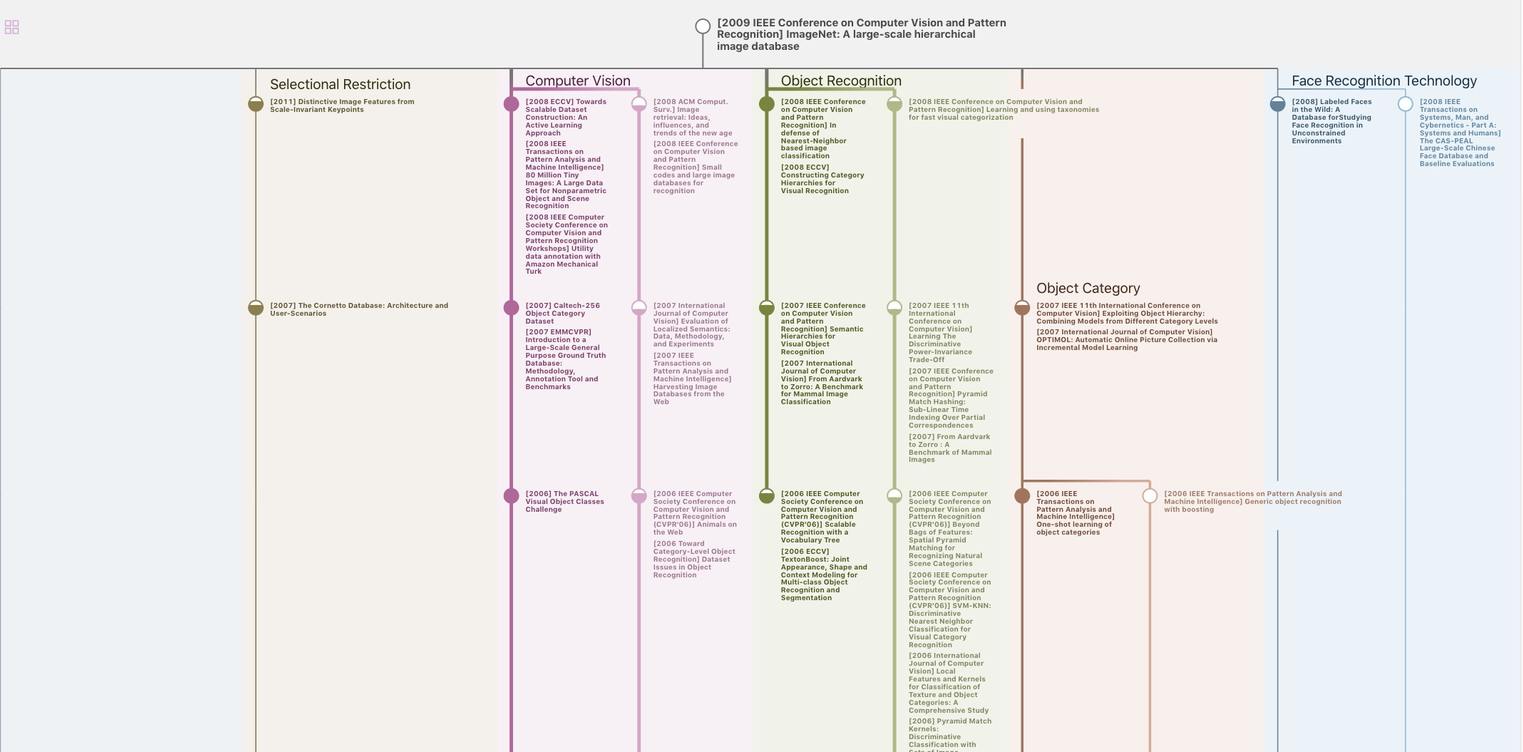
生成溯源树,研究论文发展脉络
Chat Paper
正在生成论文摘要