Out-of-Sample Representation Learning for Multi-Relational Graphs
CoRR(2020)
摘要
Many important problems can be formulated as reasoning in multi-relational graphs. Representation learning has proved extremely effective for transductive reasoning, in which one needs to make new predictions for already observed entities. This is true for both attributed graphs (where each entity has an initial feature vector) and non-attributed graphs(where the only initial information derives from known relations with other entities). For out-of-sample reasoning, where one needs to make predictions for entities that were unseen at training time, much prior work considers attributed graph. However, this problem has been surprisingly left unexplored for non-attributed graphs. In this paper, we introduce the out-of-sample representation learning problem for non-attributed multi-relational graphs, create benchmark datasets for this task, develop several models and baselines, and provide empirical analyses and comparisons of the proposed models and baselines.
更多查看译文
关键词
graphs,representation,learning,out-of-sample,multi-relational
AI 理解论文
溯源树
样例
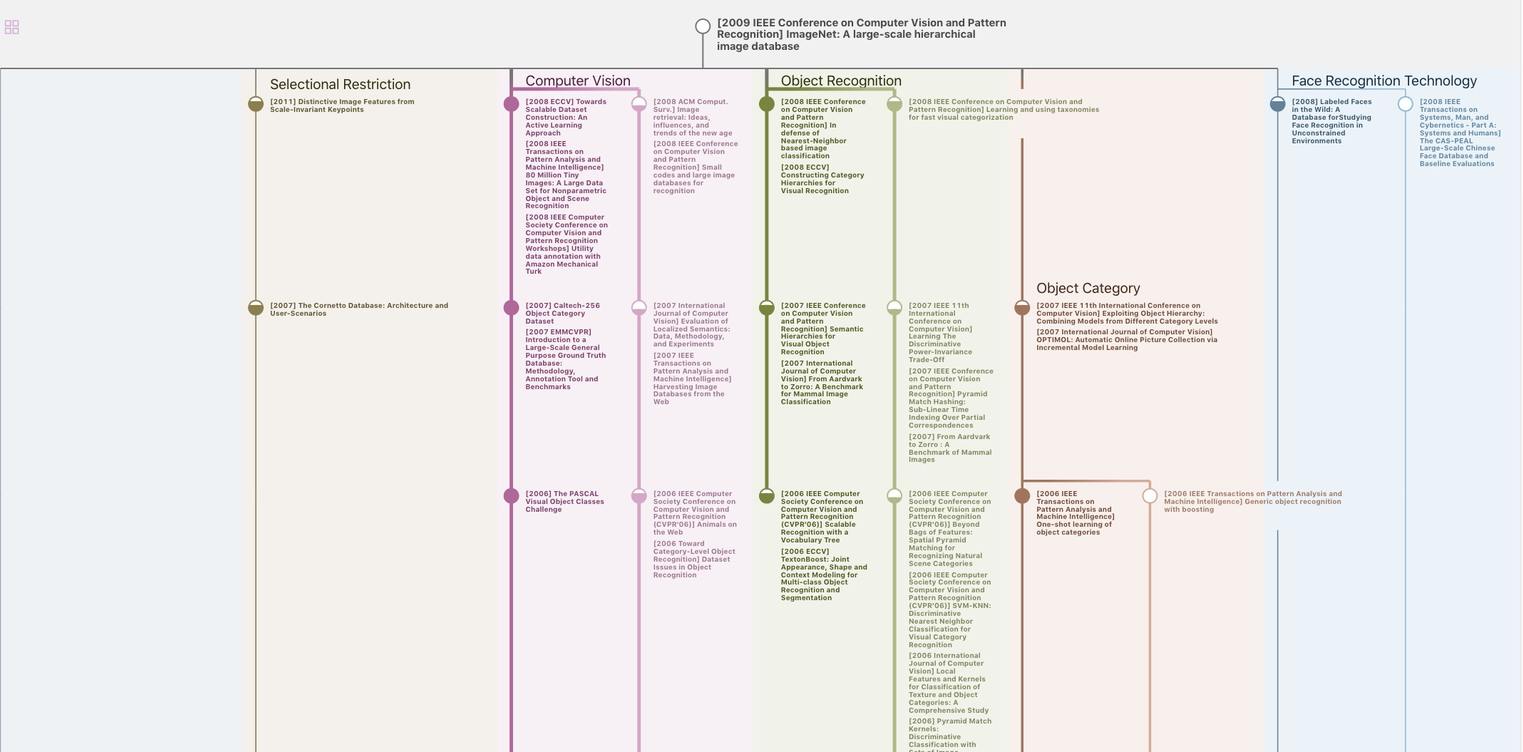
生成溯源树,研究论文发展脉络
Chat Paper
正在生成论文摘要