Conventional vs machine learning-based treatment planning in prostate brachytherapy: Results of a Phase I randomized controlled trial
BRACHYTHERAPY(2020)
摘要
PURPOSE: The purpose of this study was to evaluate the noninferiority of Day 30 dosimetry between a machine learning-based treatment planning system for prostate low-dose-rate (LDR) brachytherapy and the conventional, manual planning technique. As a secondary objective, the impact of planning technique on clinical workflow efficiency was also evaluated. MATERIALS AND METHODS: 41 consecutive patients who underwent I-125 LDR monotherapy for low- and intermediate-risk prostate cancer were accrued into this single-institution study between 2017 and 2018. Patients were 1:1 randomized to receive treatment planning using a machine learning-based prostate implant planning algorithm (PIPA system) or conventional, manual technique. Treatment plan modifications by the radiation oncologist were evaluated by computing the Dice coefficient of the prostate V-150% isodose volume between either the PIPA-or conventional-and final approved plans. Additional evaluations between groups evaluated the total planning time and dosimetric outcomes at preimplant and Day 30. RESULTS: 21 and 20 patients were treated using the PIPA and conventional techniques, respectively. No significant differences were observed in preimplant or Day 30 prostate D-90%, V-100%, rectum V-100, or rectum D-1cc between PIPA and conventional techniques. Although the PIPA group had a larger proportion of patients with plans requiring no modifications (Dice = 1.00), there was no significant difference between the magnitude of modifications between each arm. There was a large significant advantage in mean planning time for the PIPA arm (2.38 +/- 0.96 min) compared with the conventional (43.13 +/- 58.70 min) technique (p >> 0.05). CONCLUSIONS: A machine learning-based planning workflow for prostate LDR brachytherapy has the potential to offer significant time savings and operational efficiencies, while producing noninferior postoperative dosimetry to that of expert, conventional treatment planners. (C) 2020 The Authors. Published by Elsevier Inc. on behalf of American Brachytherapy Society.
更多查看译文
关键词
Machine learning,Brachytherapy,Treatment planning,Dosimetry,Low-dose rate
AI 理解论文
溯源树
样例
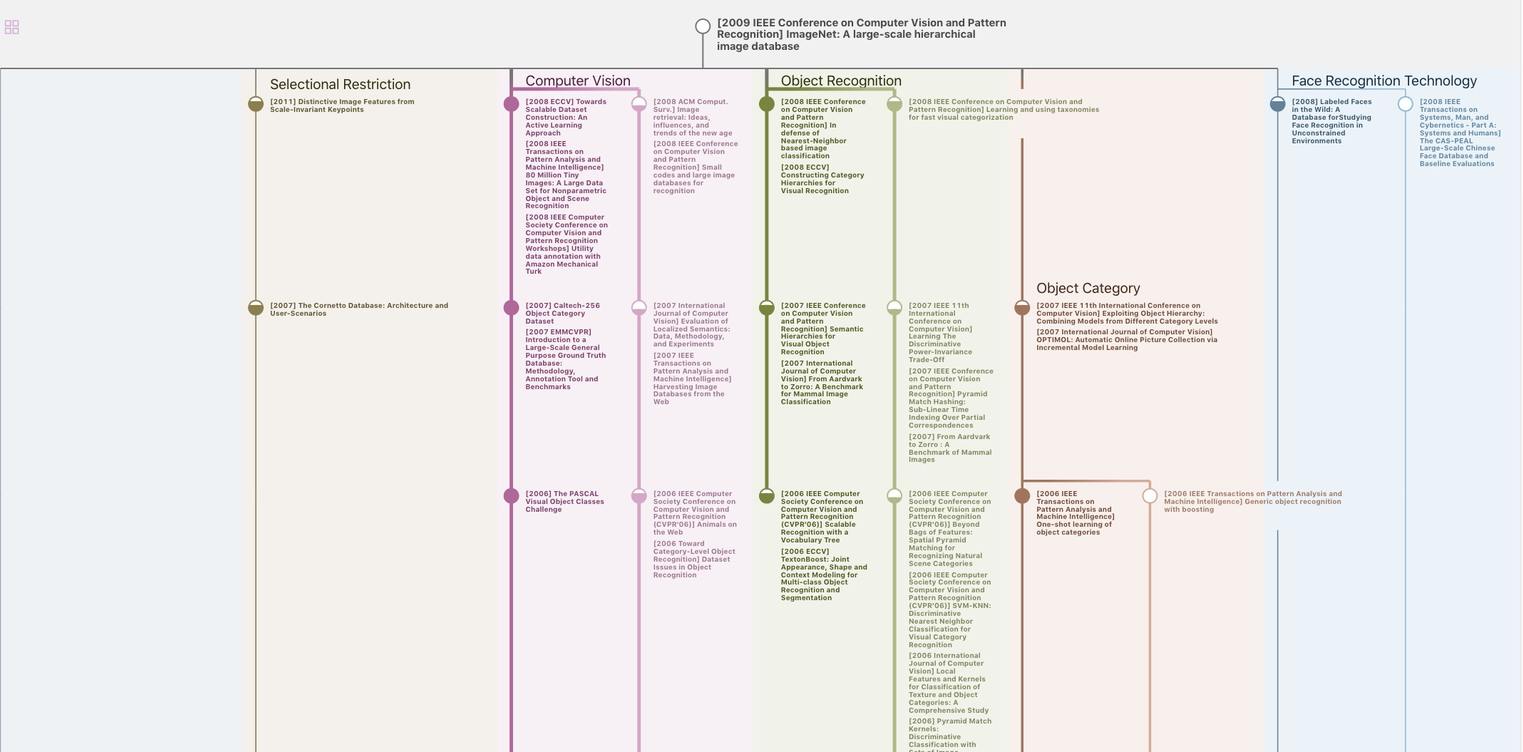
生成溯源树,研究论文发展脉络
Chat Paper
正在生成论文摘要