Parkpredict: Motion And Intent Prediction Of Vehicles In Parking Lots
2020 IEEE INTELLIGENT VEHICLES SYMPOSIUM (IV)(2020)
摘要
We investigate the problem of predicting driver behavior in parking lots, an environment which is less structured than typical road networks and features complex, interactive maneuvers in a compact space. Using the CARLA simulator, we develop a parking lot environment and collect a dataset of human parking maneuvers. We then study the impact of model complexity and feature information by comparing a multi-modal Long Short-Term Memory (LSTM) prediction model and a Convolution Neural Network LSTM (CNN-LSTM) to a physics-based Extended Kalman Filter (EKF) baseline. Our results show that 1) intent can be estimated well (roughly 85% top-1 accuracy and nearly 100% top-3 accuracy with the LSTM and CNN-LSTM model); 2) knowledge of the human driver's intended parking spot has a major impact on predicting parking trajectory; and 3) the semantic representation of the environment improves long term predictions.
更多查看译文
关键词
intent prediction,motion,vehicles
AI 理解论文
溯源树
样例
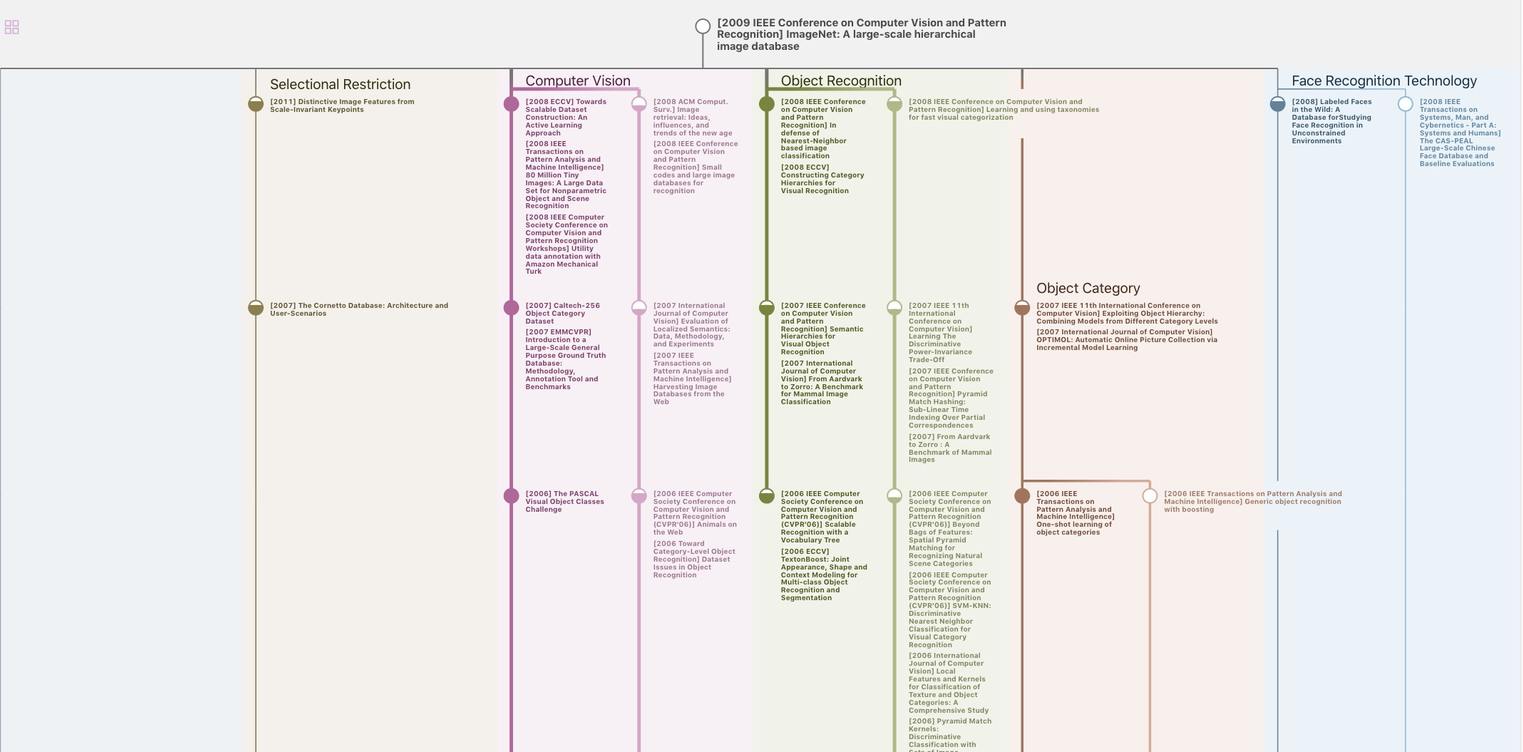
生成溯源树,研究论文发展脉络
Chat Paper
正在生成论文摘要