A category theoretical argument for causal inference
arxiv(2020)
摘要
The goal of this paper is to design a causal inference method accounting for complex interactions between causal factors. The proposed method relies on a category theoretical reformulation of the definitions of dependent variables, independent variables and latent variables in terms of products and arrows in the category of unlabeled partitions. Throughout the paper, we demonstrate how the proposed method accounts for possible hidden variables, such as environmental variables or noise, and how it can be interpreted statistically in terms of $p$-values. This interpretation, from category theory to statistics, is implemented through a collection of propositions highlighting the functorial properties of ANOVA. We use these properties in combination with our category theoretical framework to provide solutions to causal inference problems with both sound algebraic and statistical properties. As an application, we show how the proposed method can be used to design a combinatorial genome-wide association algorithm for the field of genetics.
更多查看译文
关键词
causal inference,category theoretical argument
AI 理解论文
溯源树
样例
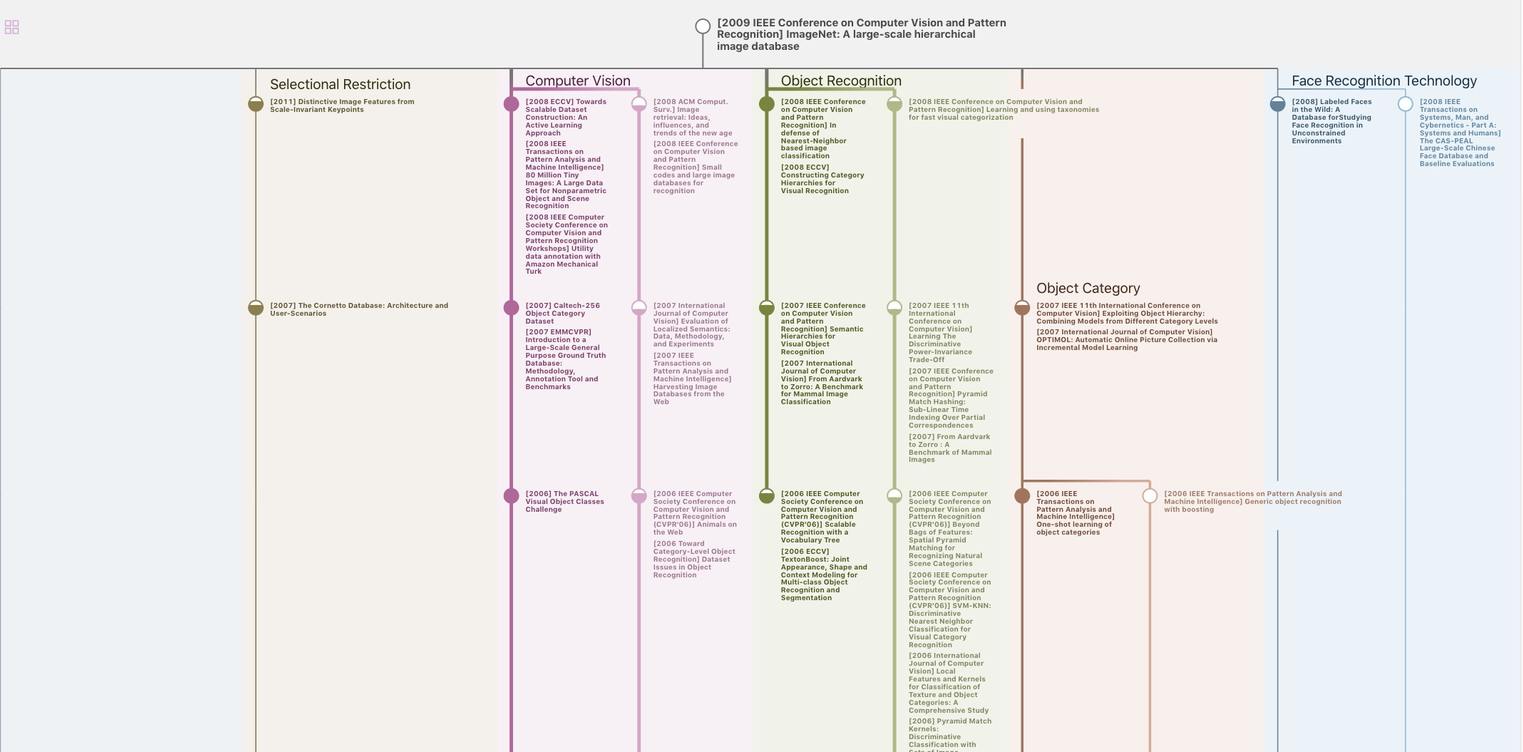
生成溯源树,研究论文发展脉络
Chat Paper
正在生成论文摘要