Tensor completion using enhanced multiple modes low-rank prior and total variation
CoRR(2020)
Abstract
In this paper, we propose a novel model to recover a low-rank tensor by simultaneously performing double nuclear norm regularized low-rank matrix factorizations to the all-mode matricizations of the underlying tensor. An block successive upper-bound minimization algorithm is applied to solve the model. Subsequence convergence of our algorithm can be established, and our algorithm converges to the coordinate-wise minimizers in some mild conditions. Several experiments on three types of public data sets show that our algorithm can recover a variety of low-rank tensors from significantly fewer samples than the other testing tensor completion methods.
MoreTranslated text
Key words
multiple modes,total variation,low-rank
AI Read Science
Must-Reading Tree
Example
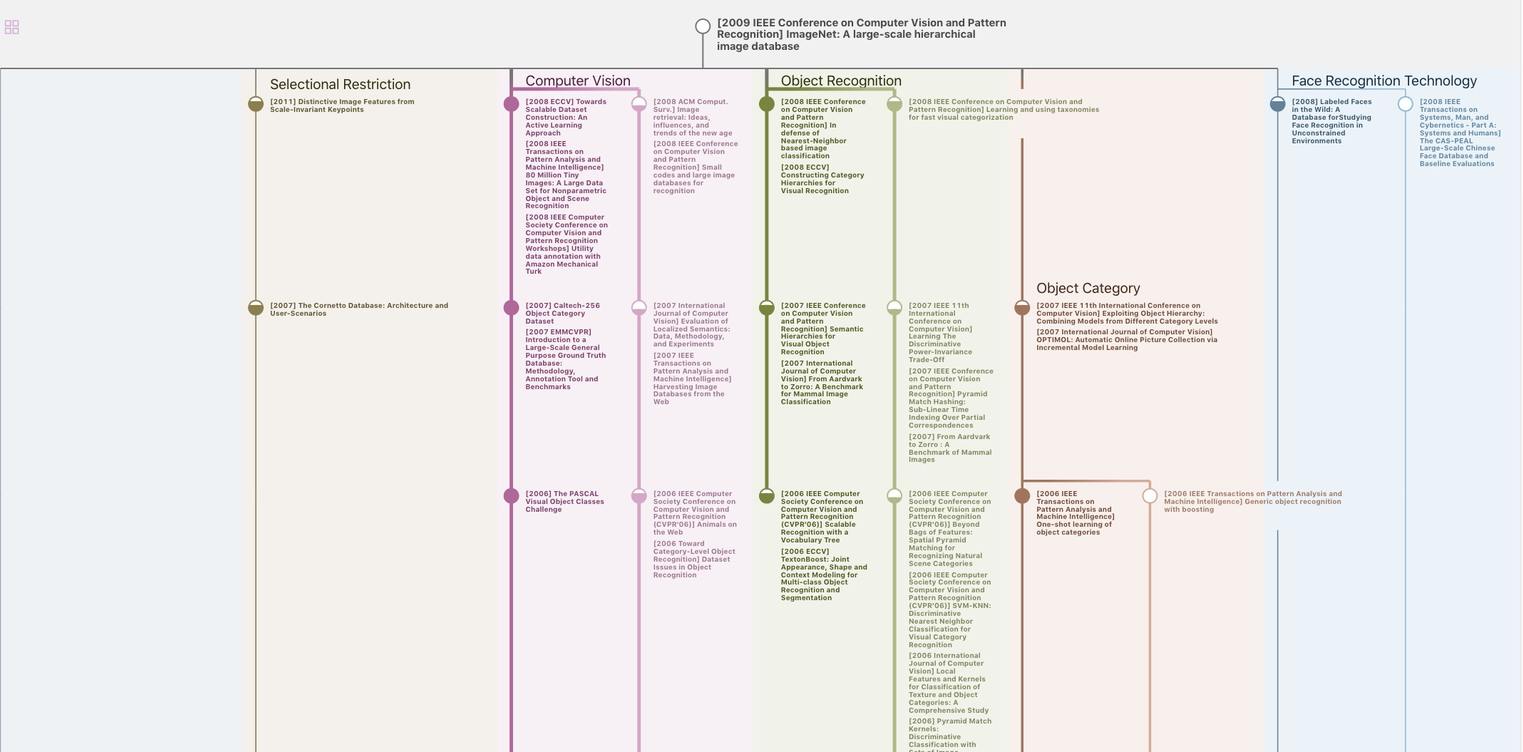
Generate MRT to find the research sequence of this paper
Chat Paper
Summary is being generated by the instructions you defined