Achieving proportional fairness in WiFi networks via bandit convex optimization
MLN(2021)
摘要
In this paper, we revisit proportional fair channel allocation in IEEE 802.11 networks. Traditional approaches are either based on the explicit solution of the optimization problem or use iterative solvers to converge to the optimum. Instead, we propose an algorithm able to learn the optimal slot transmission probability only by monitoring the throughput of the network. We have evaluated this algorithm both (i) using the true value of the function to optimize and (ii) considering estimation errors. We provide a comprehensive performance evaluation that includes assessing the sensitivity of the algorithm to different learning and network parameters as well as its reaction to network dynamics. We also evaluate the effect of noisy estimates on the convergence rate and propose a method to alleviate them. We believe our approach is a practical solution to improve the performance of wireless networks as it does not require knowing the network parameters in advance. Yet, we conclude that the setting of the parameters of the algorithm is crucial to guarantee fast convergence.
更多查看译文
关键词
Bandit convex optimization,Proportional fairness,WiFi
AI 理解论文
溯源树
样例
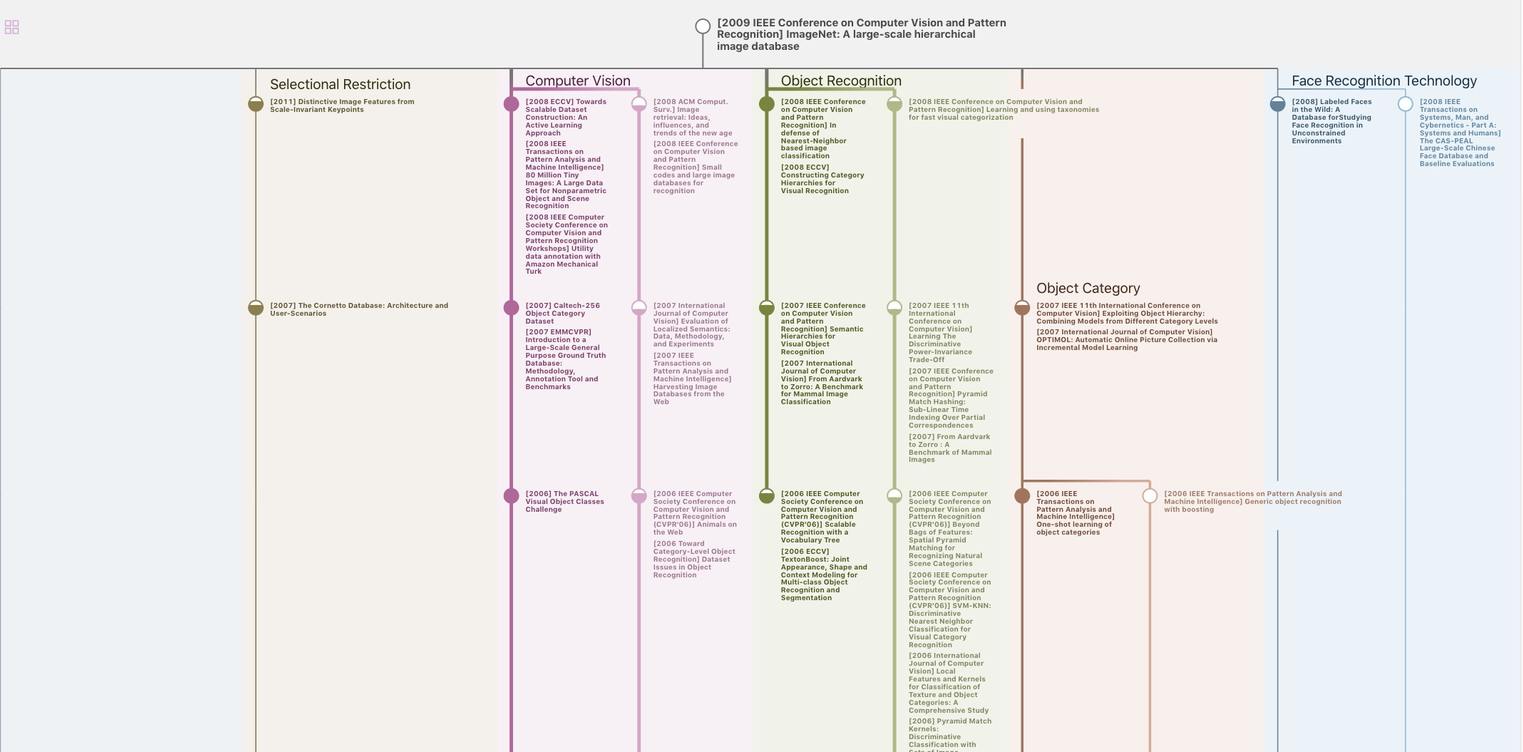
生成溯源树,研究论文发展脉络
Chat Paper
正在生成论文摘要