Stacked Autoencoder-Based Community Detection Method via an Ensemble Clustering Framework
Information Sciences(2020)
摘要
Community detection is a challenging issue because most existing methods are not well suited for complex social networks with ambiguous structures. In this paper, we propose a novel community detection method named Stacked Autoencoder-Based Community Detection Method via Ensemble Clustering (CDMEC). This is the first time that we have attempted to apply four different complex network similarity representations to the community detection problem. This work makes up for the insufficiency of the single similarity matrix to describe the similarity relationship between nodes. These similarity representations can fully describe and consider the sufficient local information between nodes in a network topology. Our CDMEC framework combines transfer learning and a stacked autoencoder to obtain an efficient low-dimensional feature representation of complex networks and aggregates multiple inputs through a novel ensemble clustering framework. This novel framework first uses the basic clustering results to construct a consistent matrix, and then it employs the nonnegative matrix factorization (NMF)-based clustering method to detect reliable clustering results from the consistent matrix. The results of various extensive experiments on artificial benchmark networks and real-world networks showed that the proposed CDMEC framework is superior to the existing state-of-the-art community detection methods and has great potential in solving the community detection problems.
更多查看译文
关键词
Community detection,Complex networks,Stacked autoencoder,Ensemble clustering
AI 理解论文
溯源树
样例
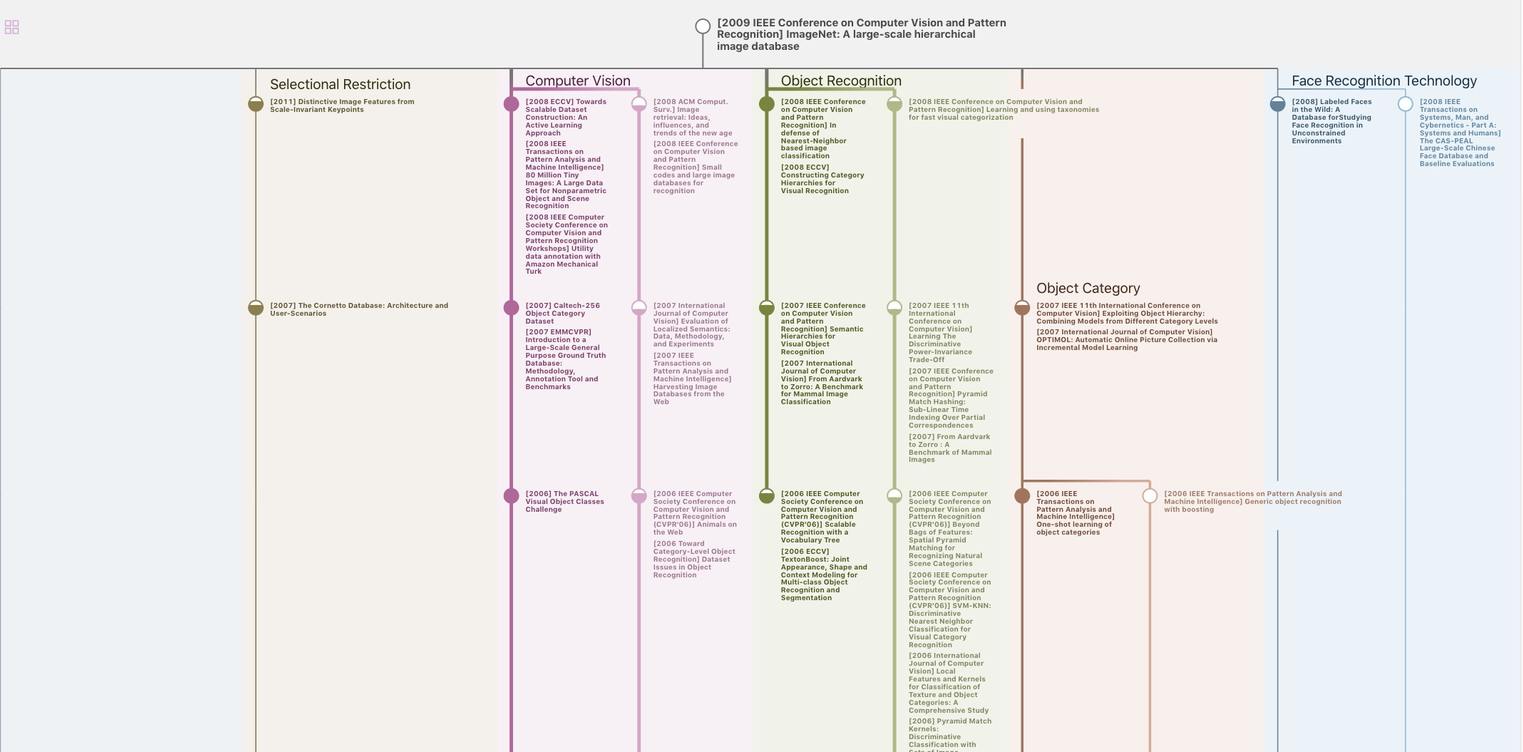
生成溯源树,研究论文发展脉络
Chat Paper
正在生成论文摘要